Reinforcement Learning-Based Dynamic Path Allocation in IoT Systems
Pan-African Artificial Intelligence and Smart Systems(2023)
摘要
With an increasingly interconnected world, Internet of Things (IoT) technologies are progressively turning into an integral part of our day-to-day lives. IoT systems, which can be seen as a set of sensor nodes connected to each other to exchange information, are subject to energy limitations. As a result of that, efficient delivery schemes for data transmission between sensor nodes are required in order to extend the network lifetime, and therefore reduce nodes’ energy drain. Various studies have been conducted to address this issue. However, only a few of them propose strategies that take into account the energy context in the network during data transmission. A lot of attention has drifted towards reinforcement learning lately, especially given its success in addressing problems that involve clustering and data collection challenges. This paper focuses on its application in Dynamic Path Prediction: an approach through which an agent, operating in a network, achieves a behavior that allows the selection of an optimal path for data transmission while minimizing network energy consumption. We performed a comparative analysis between the proposed approach and the traditional heuristics, including Dijkstra and A star. The results obtained show that our model increases the network lifetime by a factor of 2.26 compared to 1.58 obtained with A star and Dijkstra algorithms.
更多查看译文
关键词
Internet of things, Wireless sensor network, Network lifetime, Reinforcement learning
AI 理解论文
溯源树
样例
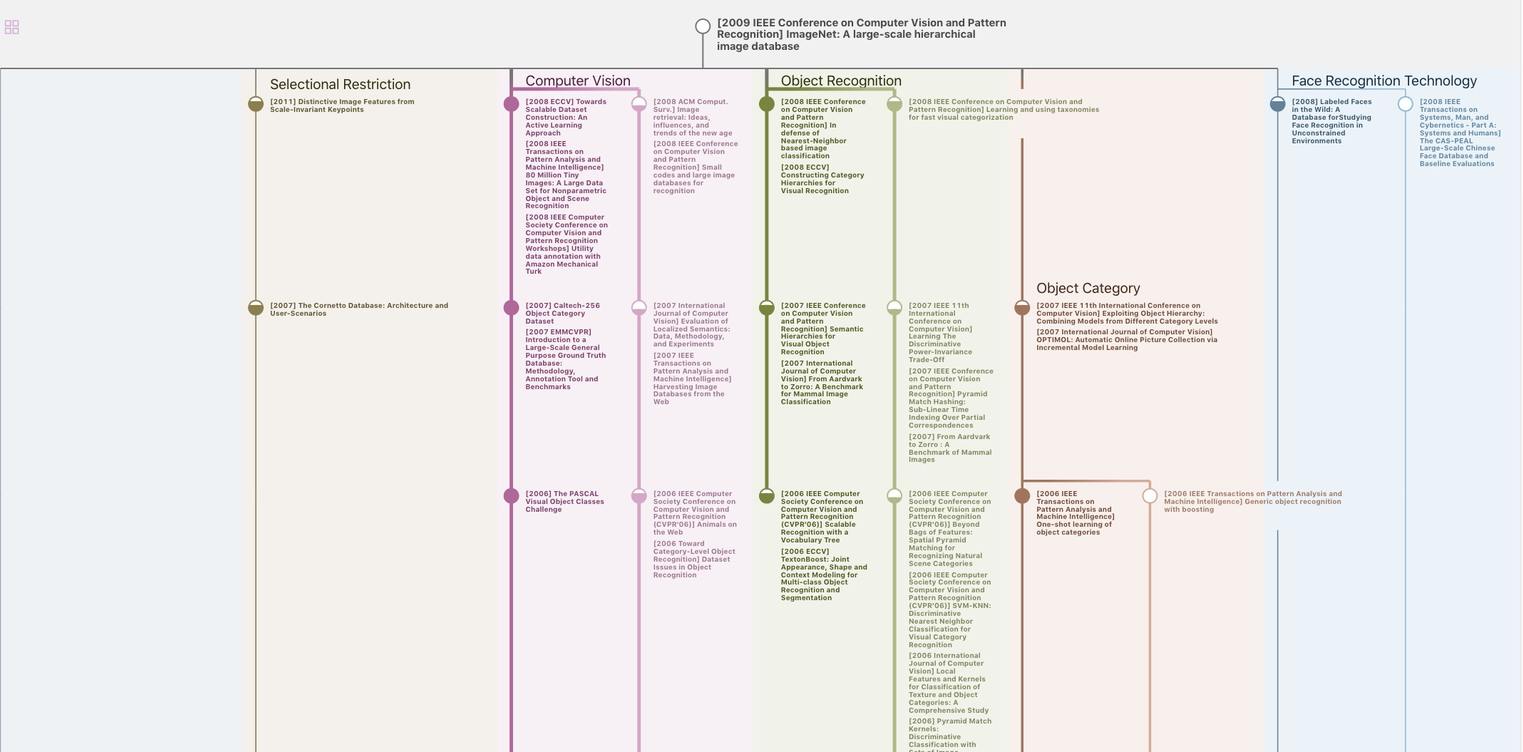
生成溯源树,研究论文发展脉络
Chat Paper
正在生成论文摘要