Benchmarking Robustness Beyond $$l_p$$ Norm Adversaries
Computer Vision – ECCV 2022 Workshops(2023)
摘要
Recently, a significant boom has been noticed in the generation of a variety of malicious examples ranging from adversarial perturbations to common noises to natural adversaries. These malicious examples are highly effective in fooling almost ‘any’ deep neural network. Therefore, to protect the integrity of deep networks, research efforts have been started in building the defense against these anomalies of the individual category. The prime reason for such individual handling of noises is the lack of one unique dataset which can be used to benchmark against multiple malicious examples and hence in turn can help in building a true ‘universal’ defense algorithm. This research work is an aid towards that goal that created a dataset termed “wide angle anomalies” containing 19 different malicious categories. On top of that, an extensive experimental evaluation has been performed on the proposed dataset using popular deep neural networks to detect these wide-angle anomalies. The experiments help in identifying a possible relationship between different anomalies and how easy or difficult to detect an anomaly if it is seen or unseen during training-testing. We assert that the experiments in seen and unseen category attack training-testing reveals several surprising and interesting outcomes including possible connection among adversaries. We believe it can help in building a universal defense algorithm.
更多查看译文
关键词
robustness,norm adversaries
AI 理解论文
溯源树
样例
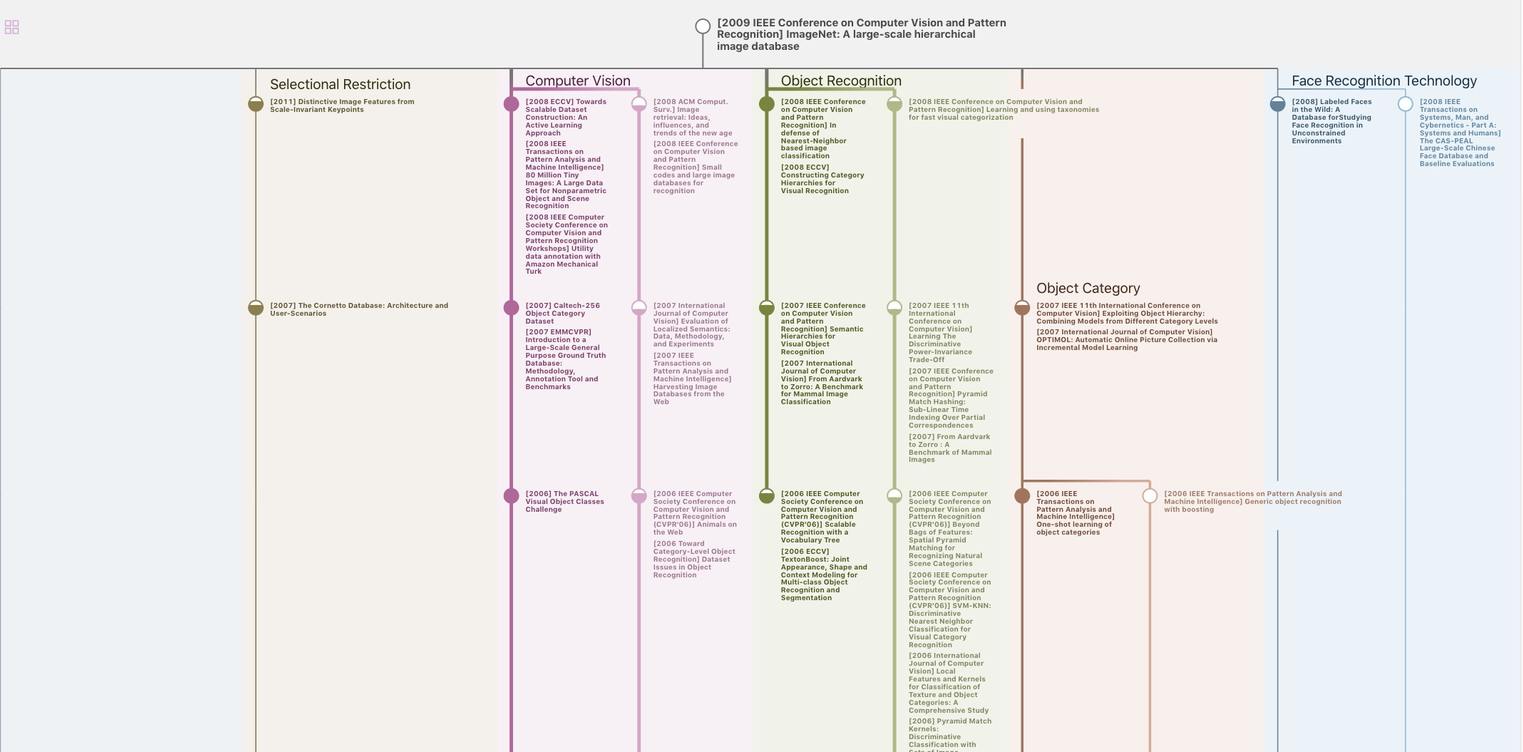
生成溯源树,研究论文发展脉络
Chat Paper
正在生成论文摘要