Temporal Convolutional Network with Attention Mechanism for Bearing Remaining Useful Life Prediction
Proceedings of TEPEN 2022(2023)
摘要
The reliability and safety of complex rotating equipment is attracting more and more attention from the industry and academia. The prediction of remaining useful life (RUL) is the most challenge task in this area. As the bearings of rotating machine usually fail with multiple faults, it is difficult to build dynamic model of its degradation process. With the development of deep learning neural network, researchers nowadays focus on the data-driven method in this field gradually. Due to the limitations of CNNs and RNNs, a modified temporal convolutional neural network (TCN) with attention mechanism is designed to solve problem of RUL prediction in this paper. Vibration signals are used as input without preprocessing. After the original TCN network, attention mechanism is used to help calculation of dynamic threshold which is the key component to sperate distinctive features from noise with different levels. In order to test the prediction ability of the model, PHM 2012 challenge data are used, the result showed that the TCN with attention mechanism has made a great improvement to the traditional 1D CNN neural network.
更多查看译文
关键词
Remaining useful life,Temporal convolutional network,Attention mechanism
AI 理解论文
溯源树
样例
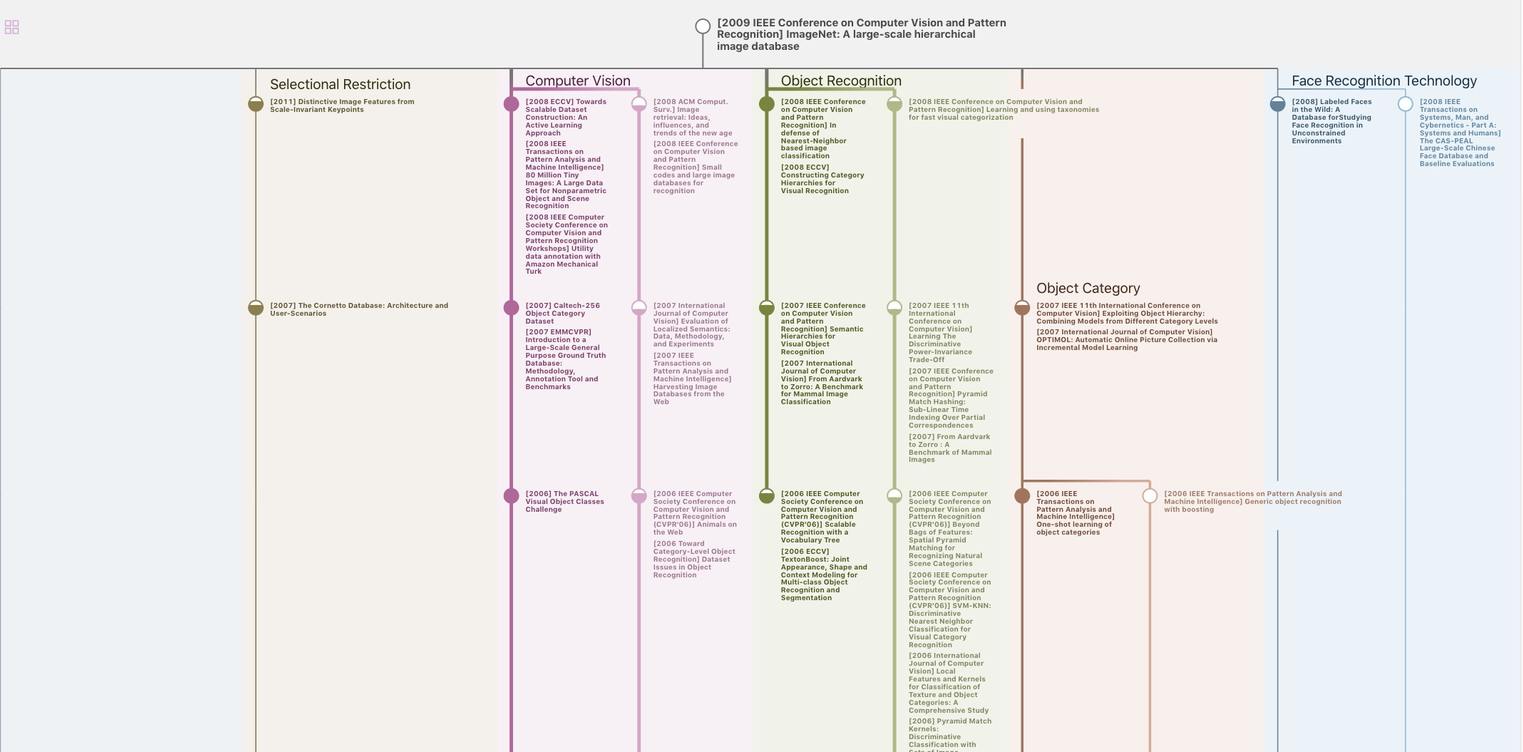
生成溯源树,研究论文发展脉络
Chat Paper
正在生成论文摘要