Enhanced Embedded AutoEncoders: An Attribute-Preserving Face De-Identification Framework
IEEE Internet of Things Journal(2023)
摘要
Nowadays, face recognition technology has been dramatically boosted by the advances in deep learning and big data fields. However, this also poses grand challenges in protecting personal identity information in intelligent applications of the Internet of Things (IoT). Existing methods based on the
$K$
-Same algorithm have low effectiveness for protecting personal identity while preserving face attributes. In this article, we propose an attribute-preserving face de-identification framework called Enhanced Embedded AutoEncoders to address this problem. Our framework consists of three parts: 1) a privacy removal network (PRN); 2) a feature selection network; and 3) a privacy evaluation network. The main purpose of our framework is to ensure that the PRN is capable of discarding information involving identity privacy and retaining desired face attributes for certain prediction applications. In order to achieve this goal, the design of the PRN is crucial. Specifically, we employ two different autoencoders, one of which is embedded within the other. Extensive experimental results show that our framework outperforms existing methods by an average of 3.42%–26.22% in terms of data utility under comparable face de-identification performance, which indicates that the proposed framework can not only effectively retain face attributes but also protect personal identity well.
更多查看译文
关键词
Autoencoders,deep learning,face attributes,face de-identification,Internet of Things (IoT),privacy
AI 理解论文
溯源树
样例
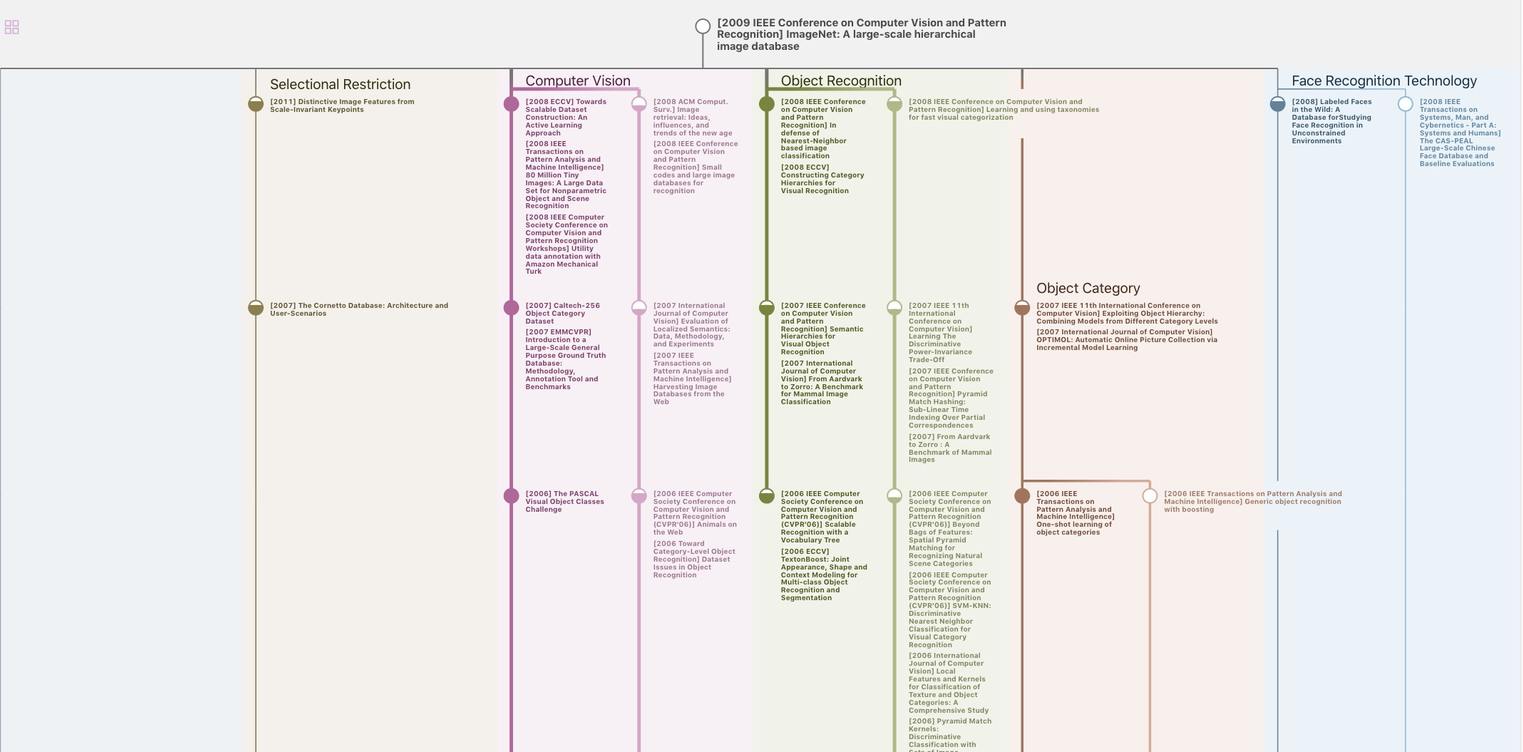
生成溯源树,研究论文发展脉络
Chat Paper
正在生成论文摘要