Improving Speaker Verification with Self-Pretrained Transformer Models
arXiv (Cornell University)(2023)
摘要
Recently, fine-tuning large pre-trained Transformer models using downstream datasets has received a rising interest. Despite their success, it is still challenging to disentangle the benefits of large-scale datasets and Transformer structures from the limitations of the pre-training. In this paper, we introduce a hierarchical training approach, named self-pretraining, in which Transformer models are pretrained and finetuned on the same dataset. Three pre-trained models including HuBERT, Conformer and WavLM are evaluated on four different speaker verification datasets with varying sizes. Our experiments show that these self-pretrained models achieve competitive performance on downstream speaker verification tasks with only one-third of the data compared to Librispeech pretraining, such as VoxCeleb1 and CNCeleb1. Furthermore, when pre-training only on the VoxCeleb2-dev, the Conformer model outperforms the one pre-trained on 94k hours of data using the same fine-tuning settings.
更多查看译文
关键词
speaker verification,transformer,self-pretrained
AI 理解论文
溯源树
样例
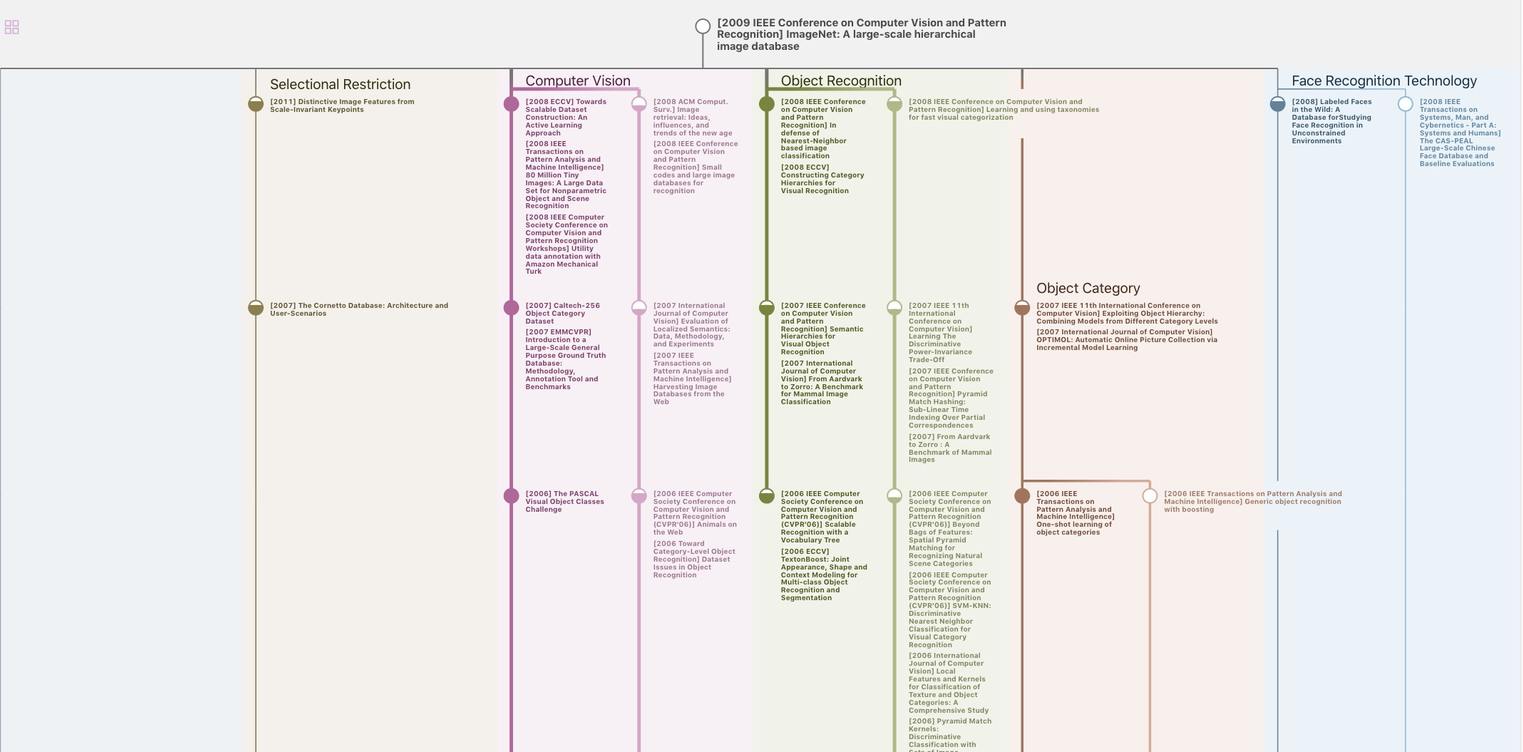
生成溯源树,研究论文发展脉络
Chat Paper
正在生成论文摘要