Scalable and Safe Remediation of Defective Actions in Self-Learning Conversational Systems
conf_acl(2023)
摘要
Off-Policy reinforcement learning has been a driving force for the state-of-the-art conversational AIs leading to more natural humanagent interactions and improving the user satisfaction for goal-oriented agents. However, in large-scale commercial settings, it is often challenging to balance between policy improvements and experience continuity on the broad spectrum of applications handled by such system. In the literature, off-policy evaluation and guard-railing on aggregate statistics has been commonly used to address this problem. In this paper, we propose a method for curating and leveraging high-precision samples sourced from historical regression incident reports to validate, safe-guard, and improve policies prior to the online deployment. We conducted extensive experiments using data from a real-world conversational system and actual regression incidents. The proposed method is currently deployed in our production system to protect customers against broken experiences and enable long-term policy improvements.
更多查看译文
关键词
defective actions,conversational systems,self-learning
AI 理解论文
溯源树
样例
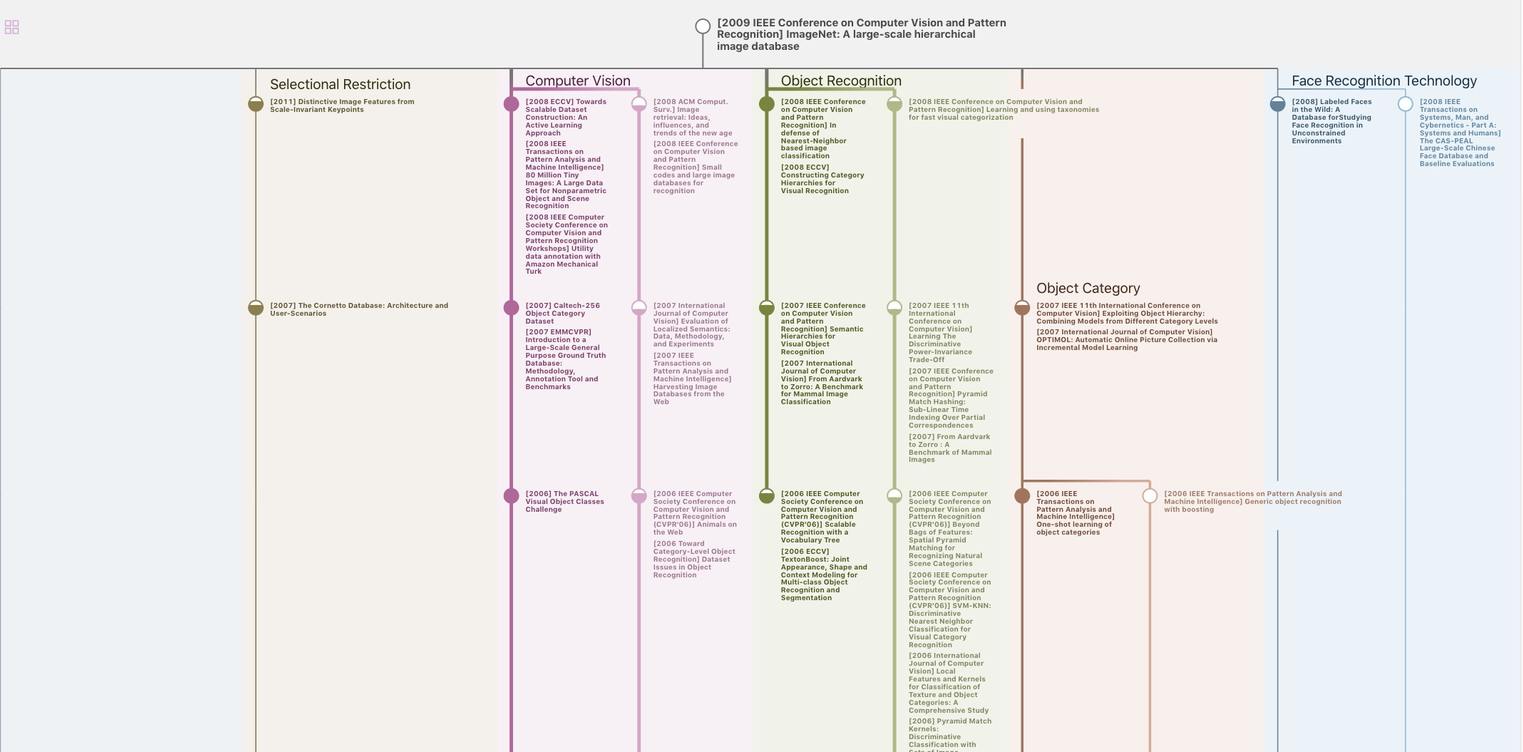
生成溯源树,研究论文发展脉络
Chat Paper
正在生成论文摘要