Self-supervised Learning for Physiologically-Based Pharmacokinetic Modeling in Dynamic PET
MEDICAL IMAGE COMPUTING AND COMPUTER ASSISTED INTERVENTION, MICCAI 2023, PT I(2023)
摘要
Dynamic Positron Emission Tomography imaging (dPET) provides temporally resolved images of a tracer. Voxel-wise physiologically-based pharmacokinetic modeling of the Time Activity Curves (TAC) extracted from dPET can provide relevant diagnostic information for clinical workflow. Conventional fitting strategies for TACs are slow and ignore the spatial relation between neighboring voxels. We train a spatio-temporal UNet to estimate the kinetic parameters given TAC from dPET. This work introduces a self-supervised loss formulation to enforce the similarity between the measured TAC and those generated with the learned kinetic parameters. Our method provides quantitatively comparable results at organ level to the significantly slower conventional approaches while generating pixel-wise kinetic parametric images which are consistent with expected physiology. To the best of our knowledge, this is the first self-supervised network that allows voxel-wise computation of kinetic parameters consistent with a non-linear kinetic model.
更多查看译文
关键词
Kinetic modelling,PBPK models,Dynamic PET
AI 理解论文
溯源树
样例
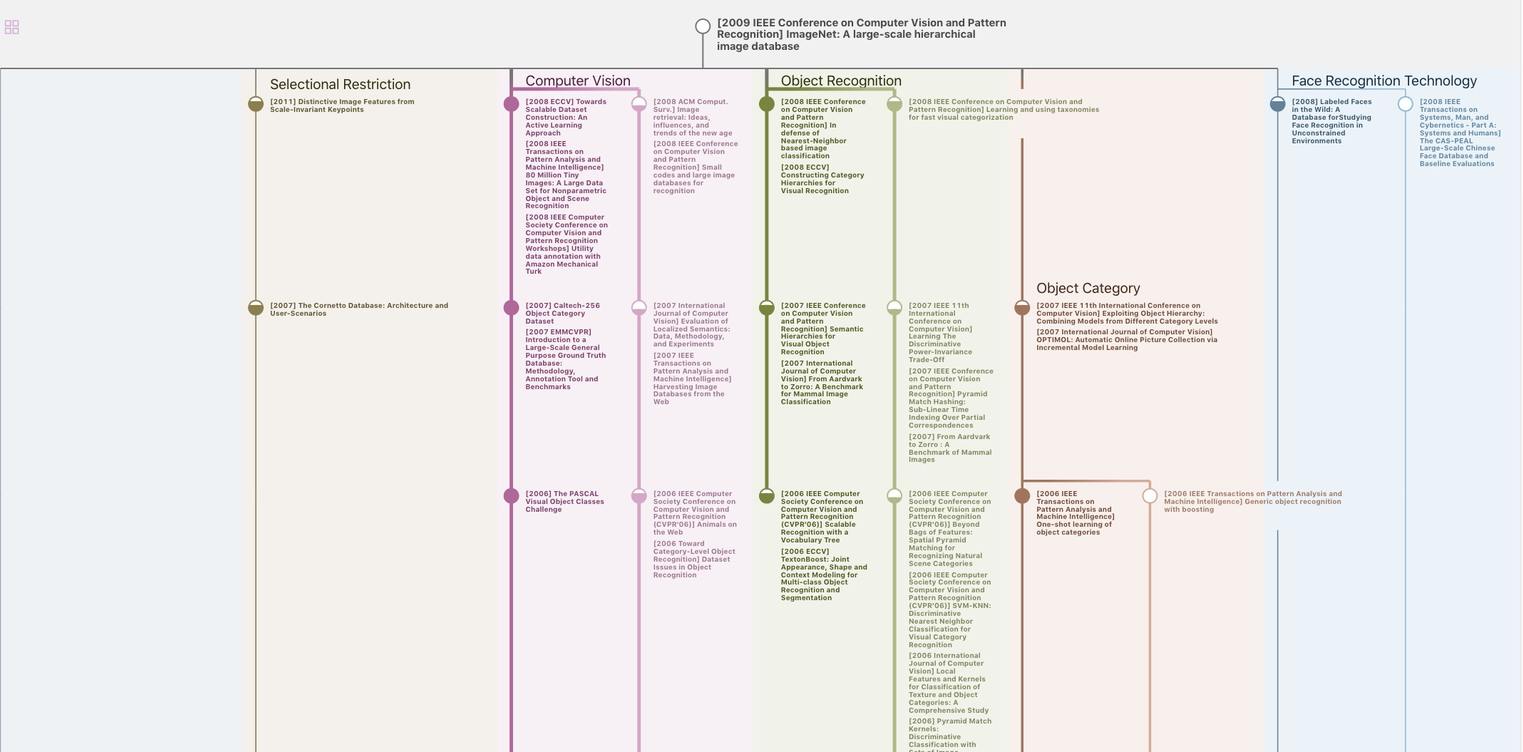
生成溯源树,研究论文发展脉络
Chat Paper
正在生成论文摘要