ZeroPrompt: Streaming Acoustic Encoders are Zero-Shot Masked LMs
CoRR(2023)
摘要
In this paper, we present ZeroPrompt (Figure 1-(a)) and the corresponding Prompt-and-Refine strategy (Figure 3), two simple but effective \textbf{training-free} methods to decrease the Token Display Time (TDT) of streaming ASR models \textbf{without any accuracy loss}. The core idea of ZeroPrompt is to append zeroed content to each chunk during inference, which acts like a prompt to encourage the model to predict future tokens even before they were spoken. We argue that streaming acoustic encoders naturally have the modeling ability of Masked Language Models and our experiments demonstrate that ZeroPrompt is engineering cheap and can be applied to streaming acoustic encoders on any dataset without any accuracy loss. Specifically, compared with our baseline models, we achieve 350 $\sim$ 700ms reduction on First Token Display Time (TDT-F) and 100 $\sim$ 400ms reduction on Last Token Display Time (TDT-L), with theoretically and experimentally equal WER on both Aishell-1 and Librispeech datasets.
更多查看译文
关键词
acoustic encoders,zero-shot
AI 理解论文
溯源树
样例
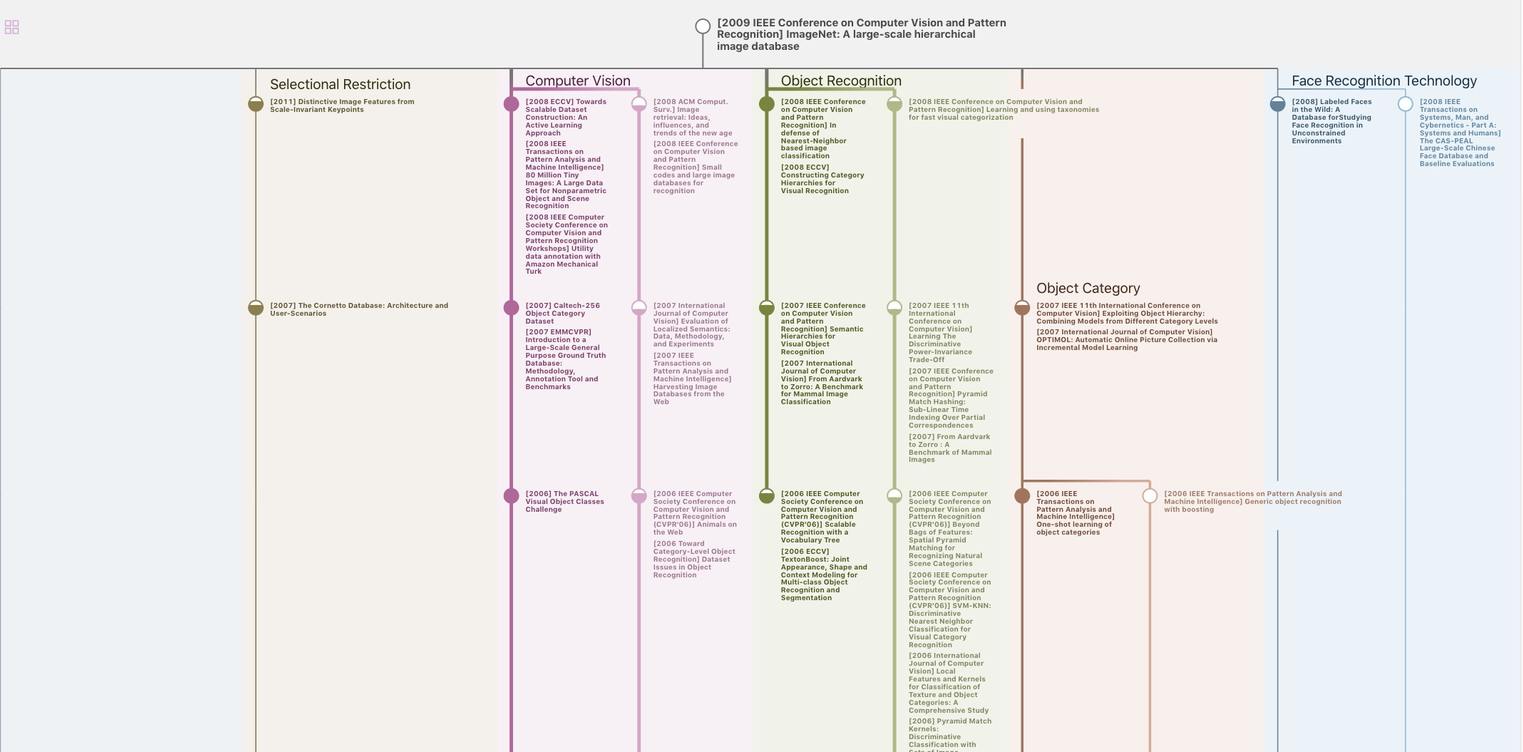
生成溯源树,研究论文发展脉络
Chat Paper
正在生成论文摘要