Lyapunov-Driven Deep Reinforcement Learning for Edge Inference Empowered by Reconfigurable Intelligent Surfaces
CoRR(2023)
摘要
In this paper, we propose a novel algorithm for energy-efficient, low-latency, accurate inference at the wireless edge, in the context of 6G networks endowed with reconfigurable intelligent surfaces (RISs). We consider a scenario where new data are continuously generated/collected by a set of devices and are handled through a dynamic queueing system. Building on the marriage between Lyapunov stochastic optimization and deep reinforcement learning (DRL), we devise a dynamic learning algorithm that jointly optimizes the data compression scheme, the allocation of radio resources (i.e., power, transmission precoding), the computation resources (i.e., CPU cycles), and the RIS reflectivity parameters (i.e., phase shifts), with the aim of performing energy-efficient edge classification with end-to-end (E2E) delay and inference accuracy constraints. The proposed strategy enables dynamic control of the system and of the wireless propagation environment, performing a low-complexity optimization on a per-slot basis while dealing with time-varying radio channels and task arrivals, whose statistics are unknown. Numerical results assess the performance of the proposed RIS-empowered edge inference strategy in terms of trade-off between energy, delay, and accuracy of a classification task.
更多查看译文
关键词
Edge intelligence,inference,reconfigurable intelligent surfaces,Lyapunov optimization,reinforcement learning
AI 理解论文
溯源树
样例
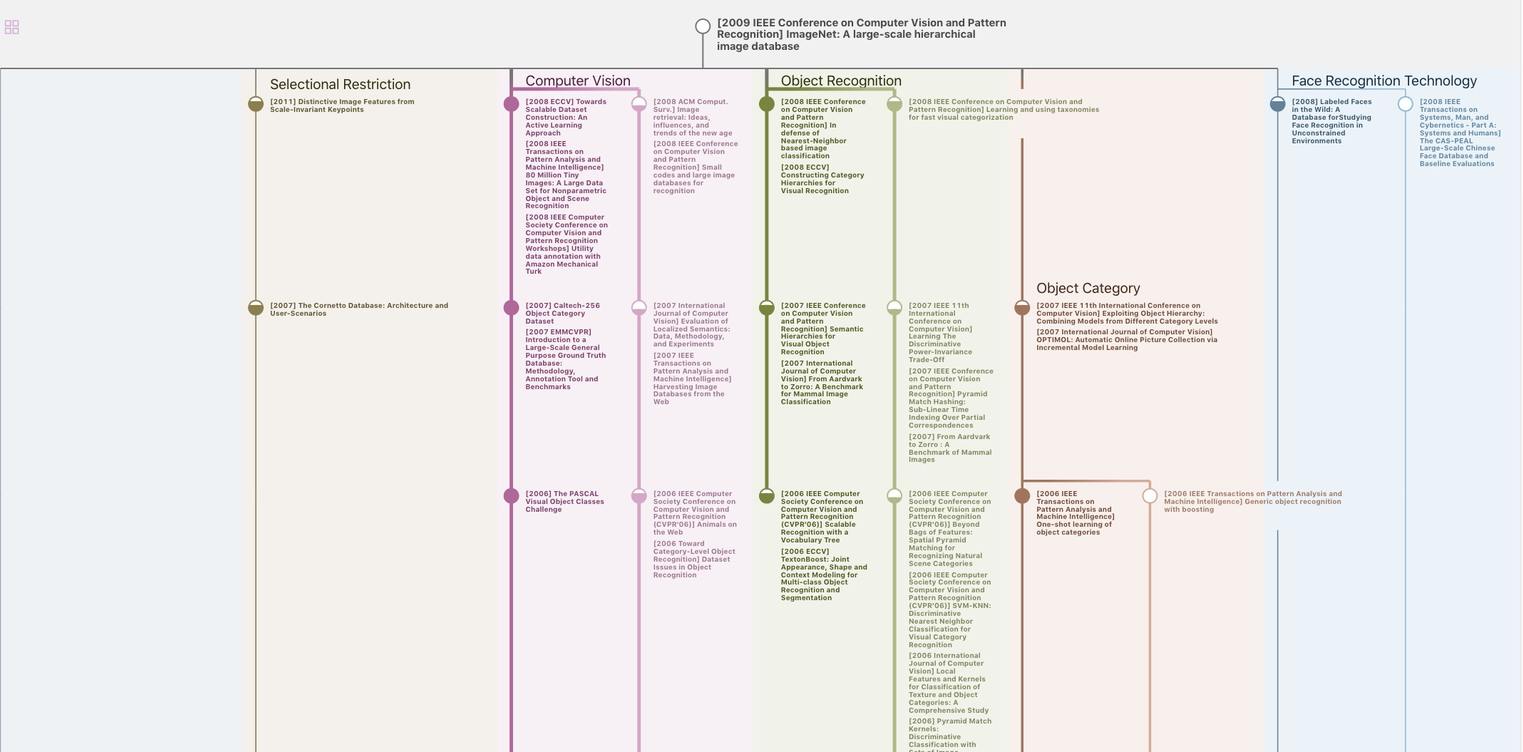
生成溯源树,研究论文发展脉络
Chat Paper
正在生成论文摘要