Preparation of cavity Fock state superpositions by reinforcement learning exploiting measurement back-action
arXiv (Cornell University)(2023)
摘要
Preparation of bosonic and general cavity quantum states usually relies on using open-loop control to reach a desired target state. In this work, a measurement-based feedback approach is used instead, exploiting the non-linearity of weak measurements alongside a coherent drive to prepare these states. The extension of previous work on Lyapunov-based control is shown to fail for this task. This prompts for a different approach, and reinforcement learning (RL) is resorted to here for this purpose. With such an approach, cavity eigenstate superpositions can be prepared with fidelities over 98$\%$ using only the measurements back-action as the non-linearity, while naturally incorporating detection of cavity photon jumps. Two different RL frameworks are analyzed: an off-policy approach recently introduced called truncated quantile critic~(TQC) and the on-policy method commonly used in quantum control, namely proximal policy optimization~(PPO). It is shown that TQC performs better at reaching higher target state fidelity preparation.
更多查看译文
关键词
cavity fock state superpositions,reinforcement
AI 理解论文
溯源树
样例
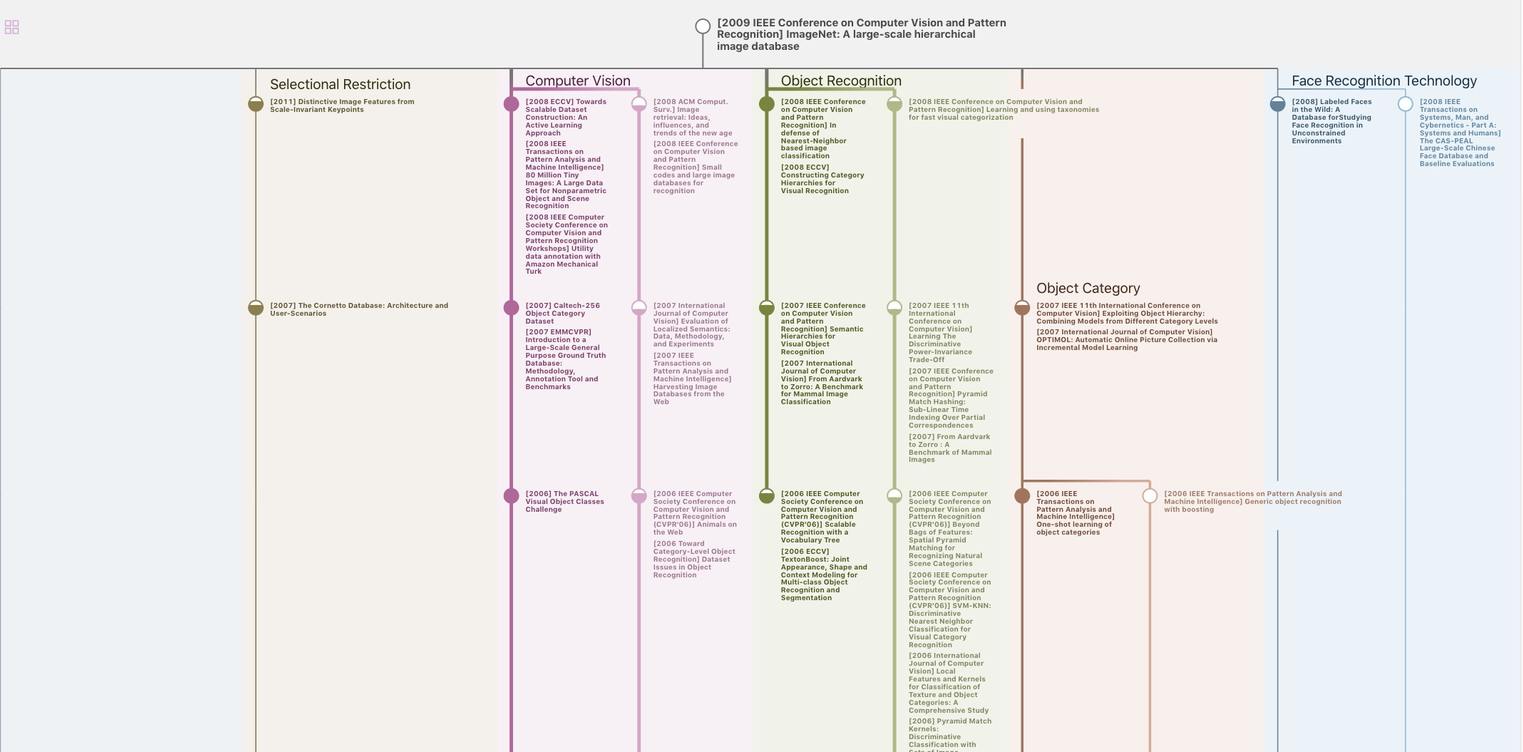
生成溯源树,研究论文发展脉络
Chat Paper
正在生成论文摘要