Realistic cell type annotation and discovery for single-cell RNA-seq data
IJCAI 2023(2023)
摘要
The rapid development of single-cell RNA sequencing (scRNA-seq) technologies allows us to explore tissue heterogeneity at the cellular level. Cell annotation plays an essential role in the substantial downstream analysis of scRNA-seq data. Existing methods usually classify the novel cells in target data as an "unassigned" group and rarely discover the fine-grained cell type structure among them. Besides, these methods carry risks, such as susceptibility to batch effect between reference and target data, thus further compromising of inherent discrimination of target data. Considering these limitations, here we propose a new and practical task called realistic cell type annotation and discovery for scRNA-seq data. In this task, cells from seen cell types are given class labels, while cells from novel cell types are given cluster labels. To tackle this problem, we propose an end-to-end algorithm called scPOT from the perspective of optimal transport (OT). Specifically, we first design an OT-based prototypical representation learning paradigm to encourage both global discriminations of clusters and local consistency of cells to uncover the intrinsic structure of target data. Then we propose an unbalanced OT-based partial alignment strategy with statistical filling to detect the cells from seen cell types across reference and target data. Notably, scPOT also introduces an easy yet effective solution to automatically estimate the total cell type number in target data. Extensive results on our carefully designed evaluation benchmarks demonstrate the superiority of scPOT over various state-of-the-art clustering and annotation methods.
更多查看译文
AI 理解论文
溯源树
样例
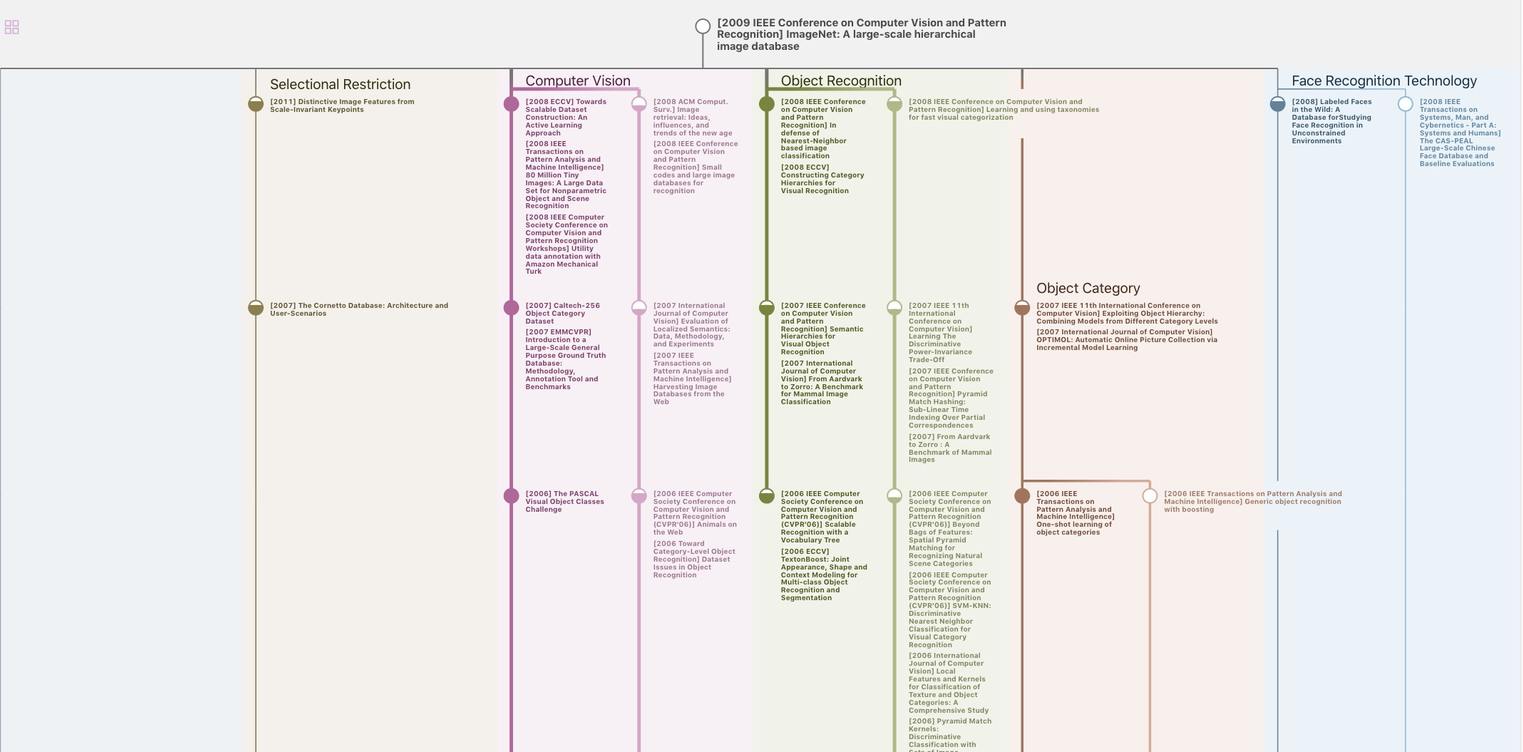
生成溯源树,研究论文发展脉络
Chat Paper
正在生成论文摘要