Incorporating Unlikely Negative Cues for Distinctive Image Captioning
IJCAI 2023(2023)
摘要
Recent neural image captioning models have achieved promising results on some automatic metrics, yet suffer badly from the generic sentence problem, limiting their applications to a few toy scenarios. An interesting approach, namely negative training, has been proposed to remind the model not to generate a high-frequency while meaningless sentence. However, its usability in image captioning is hindered by one issue, only considering frequency perspective will ignore the low-frequency but generic and vague sentences, especially facing diversified visual scenes. In this paper, we propose to incorporate unlikely \emph{negative} knowledge into image captioning, to keep the model away from undesirable generic descriptions while avoiding the above problems. Specifically, we first train a negative teacher model that can produce image-wise generic sentences with retrieval entropy-filtered data, and then the student model is required to maximize the distance with multi-level negative knowledge transferring. Empirical results on the MS COCO benchmark verify that our plug-and-play unlikely negative framework shows a significant performance gain in both accuracy and diversity, compared to previous state-of-the-art distinctive image captioning methods.
更多查看译文
AI 理解论文
溯源树
样例
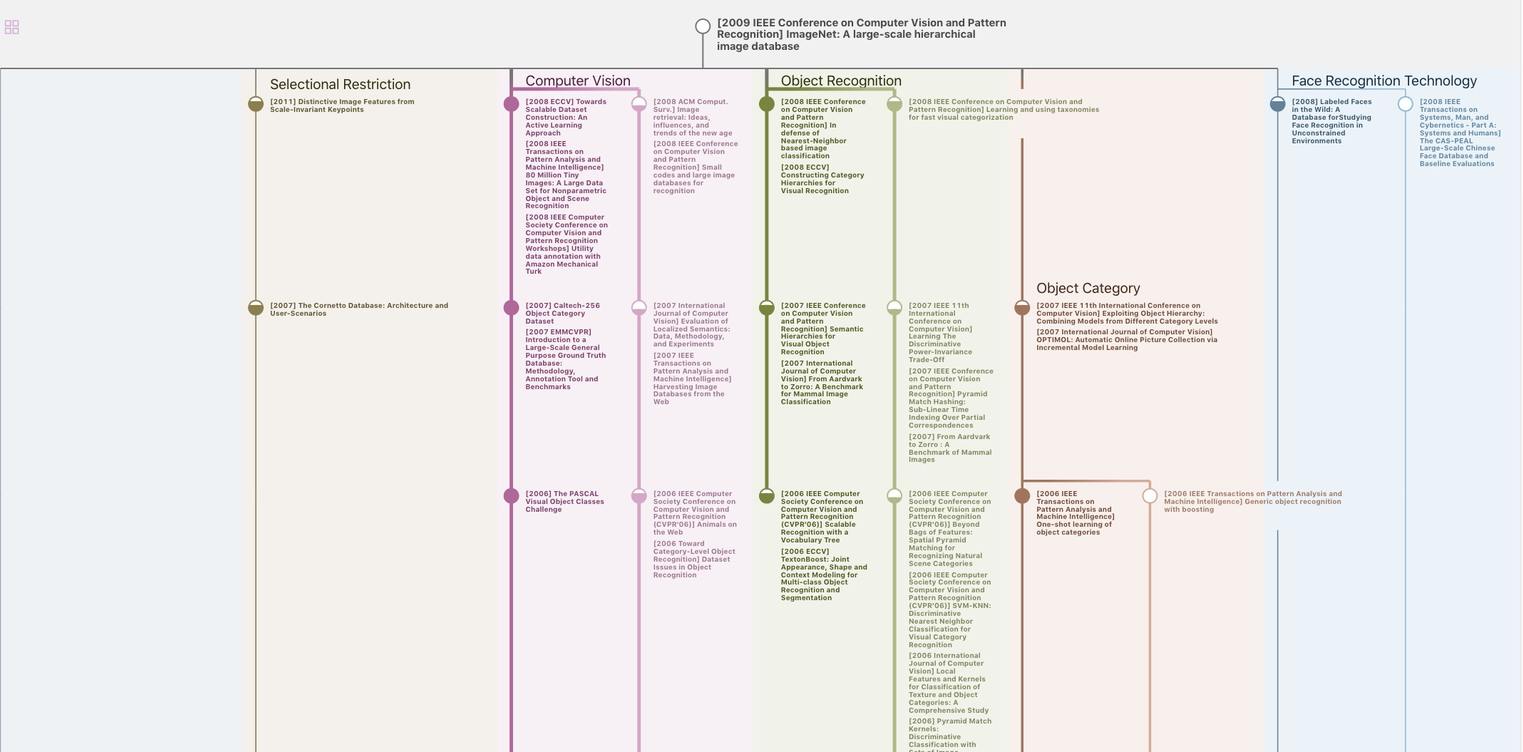
生成溯源树,研究论文发展脉络
Chat Paper
正在生成论文摘要