Decentralized anomaly detection in cooperative multi-agent reinforcement learning
IJCAI 2023(2023)
摘要
We consider the problem of detecting adversarial attacks against cooperative multi-agent reinforcement learning. We propose a decentralized scheme that allows agents to detect the abnormal behavior of one compromised agent. Our approach is based on a recurrent neural network (RNN) trained during cooperative learning to predict the action distribution of other agents based on local observations. The predicted distribution is used for computing a normality score for the agents, which allows the detection of the misbehavior of other agents. To explore the robustness of the proposed detection scheme, we formulate the worst-case attack against our scheme as a constrained reinforcement learning problem. We propose to compute an attack policy via optimizing the corresponding dual function using reinforcement learning. Extensive simulations on various multi-agent benchmarks show the effectiveness of the proposed detection scheme in detecting state of the art attacks and in limiting the impact of undetectable attacks.
更多查看译文
AI 理解论文
溯源树
样例
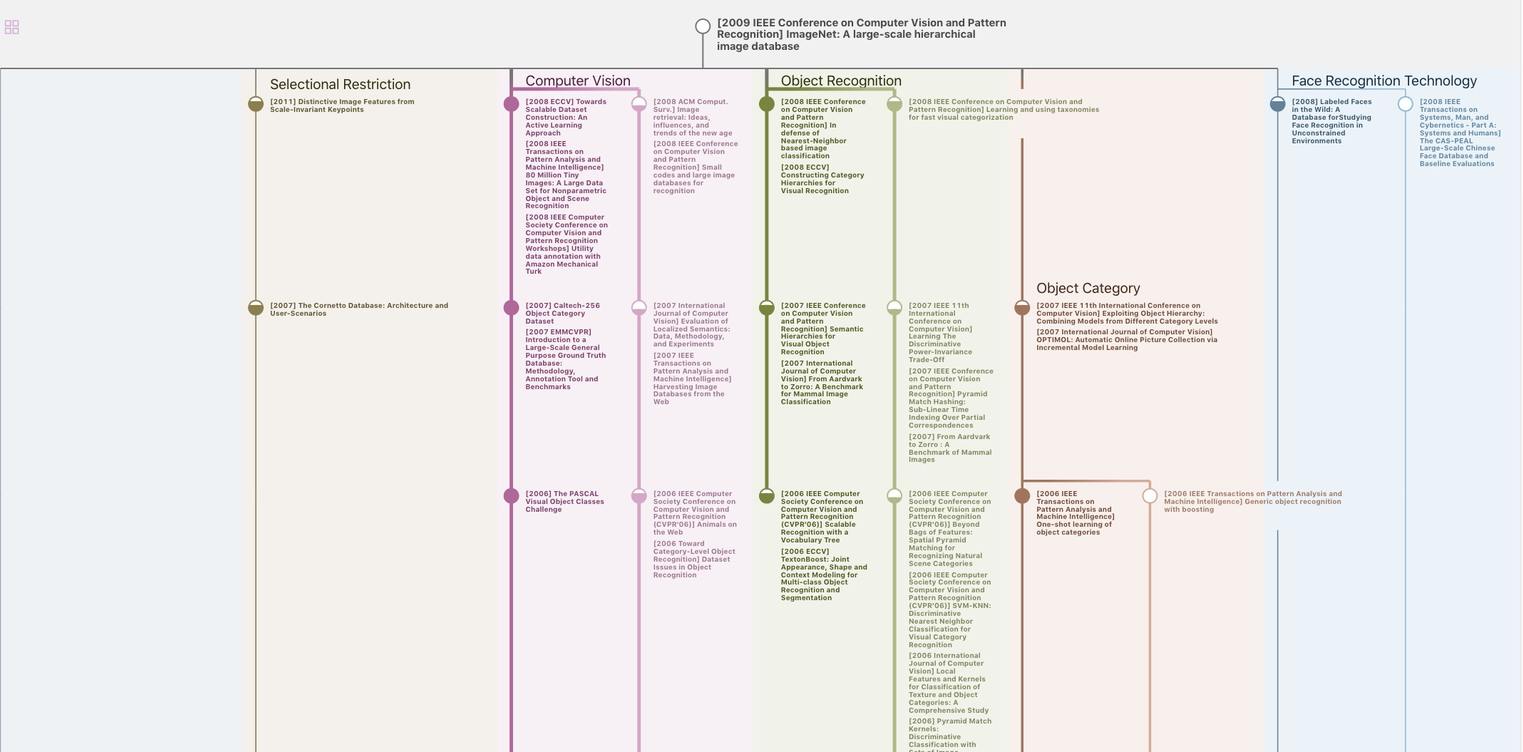
生成溯源树,研究论文发展脉络
Chat Paper
正在生成论文摘要