SAD: semi-supervised anomaly detection on dynamic graphs
IJCAI 2023(2023)
摘要
Anomaly detection aims to distinguish abnormal instances that deviate significantly from the majority of benign ones. As instances that appear in the real world are naturally connected and can be represented with graphs, graph neural networks become increasingly popular in tackling the anomaly detection problem. Despite the promising results, research on anomaly detection has almost exclusively focused on static graphs while the mining of anomalous patterns from dynamic graphs is rarely studied but has significant application value. In addition, anomaly detection is typically tackled from semi-supervised perspectives due to the lack of sufficient labeled data. However, most proposed methods are limited to merely exploiting labeled data, leaving a large number of unlabeled samples unexplored. In this work, we present semi-supervised anomaly detection (SAD) , an end-to-end framework for anomaly detection on dynamic graphs. By a combination of a time-equipped memory bank and a pseudo-label contrastive learning module , SAD is able to fully exploit the potential of large unlabeled samples and uncover underlying anomalies on evolving graph streams. Extensive experiments on four real-world datasets demonstrate that SAD efficiently discovers anomalies from dynamic graphs and outperforms existing advanced methods even when provided with only little labeled data.
更多查看译文
关键词
anomaly detection,graphs,semi-supervised
AI 理解论文
溯源树
样例
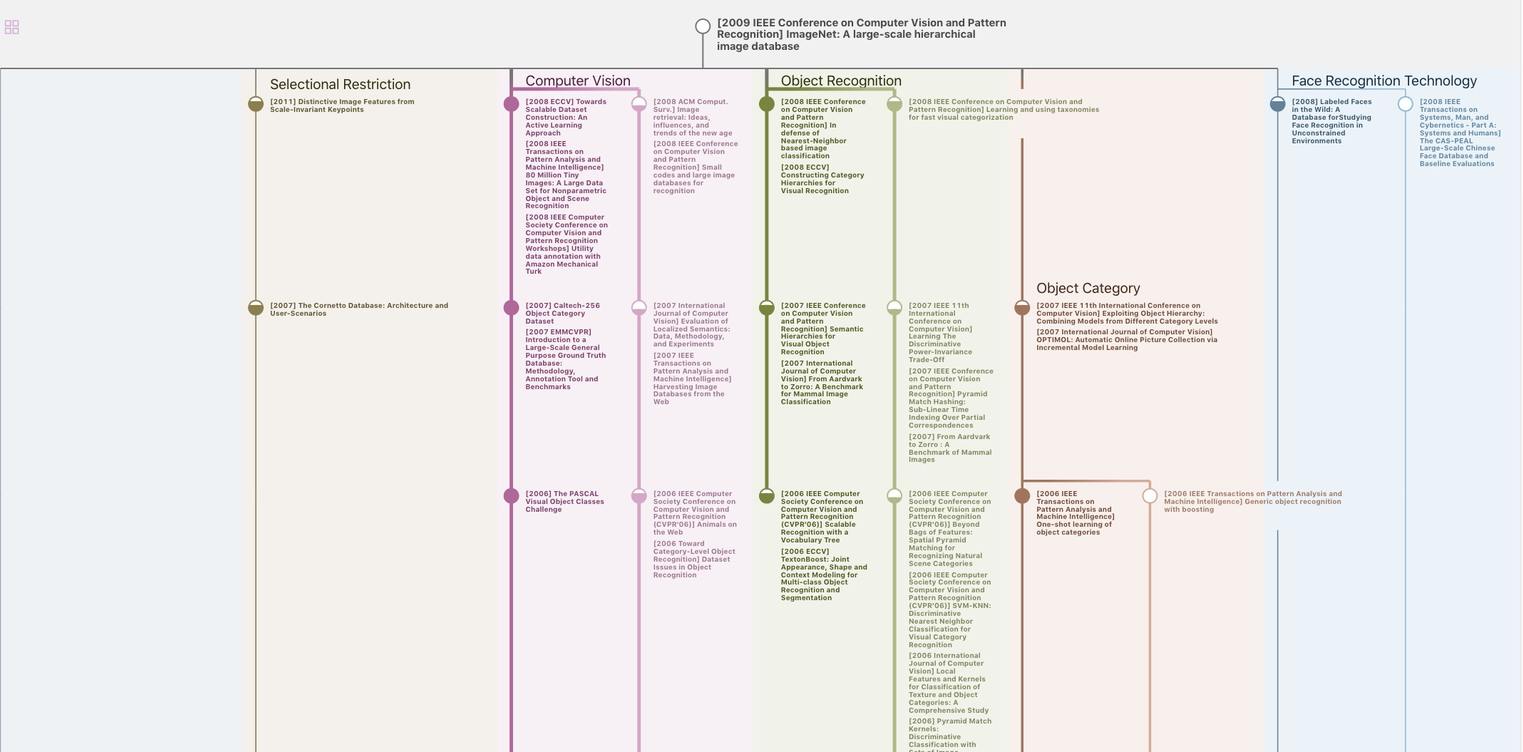
生成溯源树,研究论文发展脉络
Chat Paper
正在生成论文摘要