Denoised Self-Augmented Learning for Social Recommendation
IJCAI 2023(2023)
摘要
Social recommendation has been increasingly investigated in a broad spectrum of online applications (e.g., e-commerce, online streaming) to leverage social information for help user-item interaction modeling. Recently, Self-Supervised Learning (SSL) has been outstandingly successful in alleviating data sparsity with the augmented learning tasks. Inspired by this, recent attempts bring the benefits of SSL into social recommendation by supplementing the main supervised task with social-aware self-supervised signals. However, social information is unavoidably noisy for characterizing user preference, due to the ubiquitous presence of interest-irrelevant social connections, e.g., colleagues or classmates who do not share many common interests. To rectify this, we propose a new social recommender with a Denoised Cross-view Self-Augmented Learning paradigm (DSAL). It not only preserves the helpful social relations for enhancing user-item interaction modeling, but also allows the personalized cross-view knowledge transfer with adaptive semantic alignment in embedding space. Experimental results on various recommendation benchmarks verify the advantages of our DSAL over state-of-the-art methods.
更多查看译文
关键词
social,learning,self-augmented
AI 理解论文
溯源树
样例
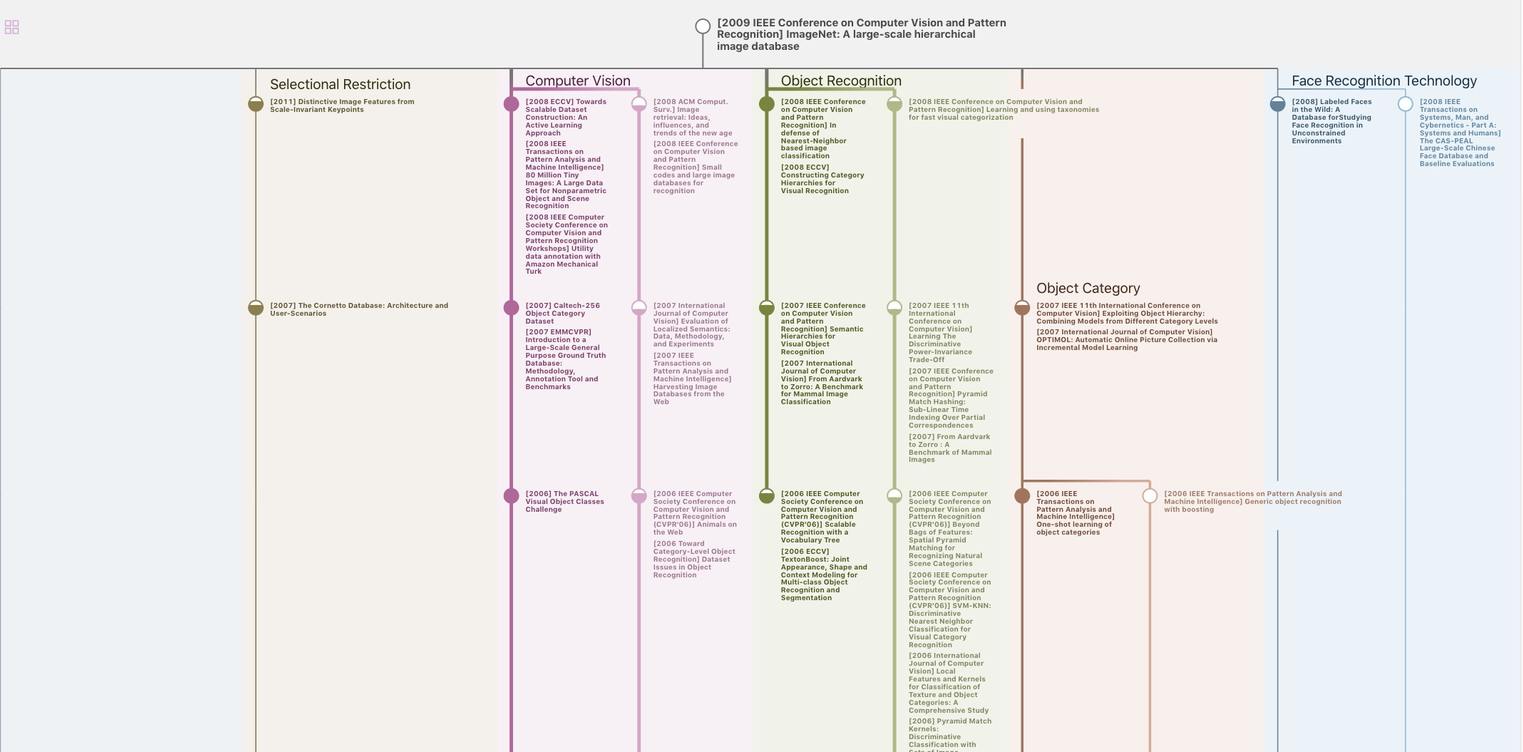
生成溯源树,研究论文发展脉络
Chat Paper
正在生成论文摘要