Exploiting non-interactive exercises in cognitive diagnosis
IJCAI 2023(2023)
摘要
Cognitive Diagnosis aims to quantify the proficiency level of students on specific knowledge concepts. Existing studies merely leverage observed historical students-exercise interaction logs to access proficiency levels. Despite effectiveness, observed interactions usually exhibit a power-law distribution, where the long tail consisting of students with few records lacks supervision signals. This phenomenon leads to inferior diagnosis among few records students. In this paper, we propose the Exercise-aware Informative Response Sampling (EIRS) framework to address the longtail problem. EIRS is a general framework that explores the partial order between observed and unobserved responses as auxiliary ranking-based training signals to supplement cognitive diagnosis. Considering the abundance and complexity of unobserved responses, we first design an Exerciseaware Candidates Selection module, which helps our framework produce reliable potential responses for effective supplementary training. Then, we develop an Expected Ability Change-weighted Informative Sampling strategy to adaptively sample informative potential responses that contribute greatly to model training. Experiments on real-world datasets demonstrate the supremacy of our framework in long-tailed data.
更多查看译文
AI 理解论文
溯源树
样例
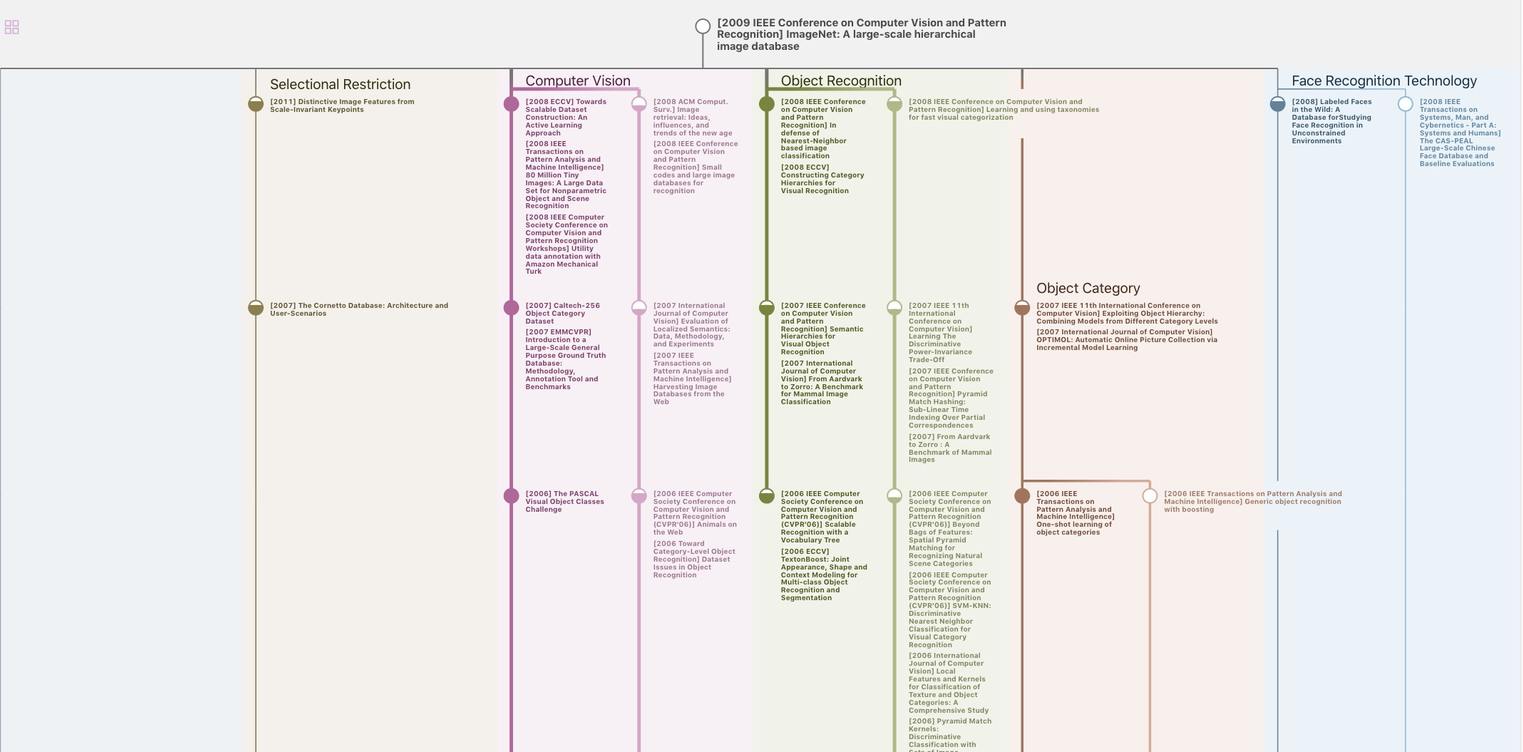
生成溯源树,研究论文发展脉络
Chat Paper
正在生成论文摘要