Doubly Stochastic Graph-based Non-autoregressive Reaction Prediction
IJCAI 2023(2023)
摘要
Organic reaction prediction is an important task in drug discovery. Recently, non-autoregressive reaction prediction has been achieved through modeling redistribution of electrons, reaching state-of-the-art top-1 accuracy and enabling parallel sampling. However, the current non-autoregressive decoder does not simultaneously fulfill two important rules of electron distribution modeling, the electron-counting rule and the symmetry rule, which violates the physical constraints of chemical reactions and thereby impairs the model performance. In this work, we propose a novel framework ReactionSink by combining two doubly stochastic self-attention mappings to obtain electron redistribution predictions that follow the above two constraints and further extend our solution to general multi-head attention mechanism with augmented constraints. To achieve this, we apply Sinkhorn’s algorithm to iteratively update self-attention mappings, which imposes doubly conservative constraint as an additional information prior on electron redistribution modeling. We theoretically show that our ReactionSink can satisfy both rules at the same time while the current decoder mechanism has to violate either of them. Empirical results demonstrate that our approach consistently improves the predictive performance of non-autoregressive models and does not bring unbearable additional computational cost.
更多查看译文
关键词
stochastic,prediction,graph-based,non-autoregressive
AI 理解论文
溯源树
样例
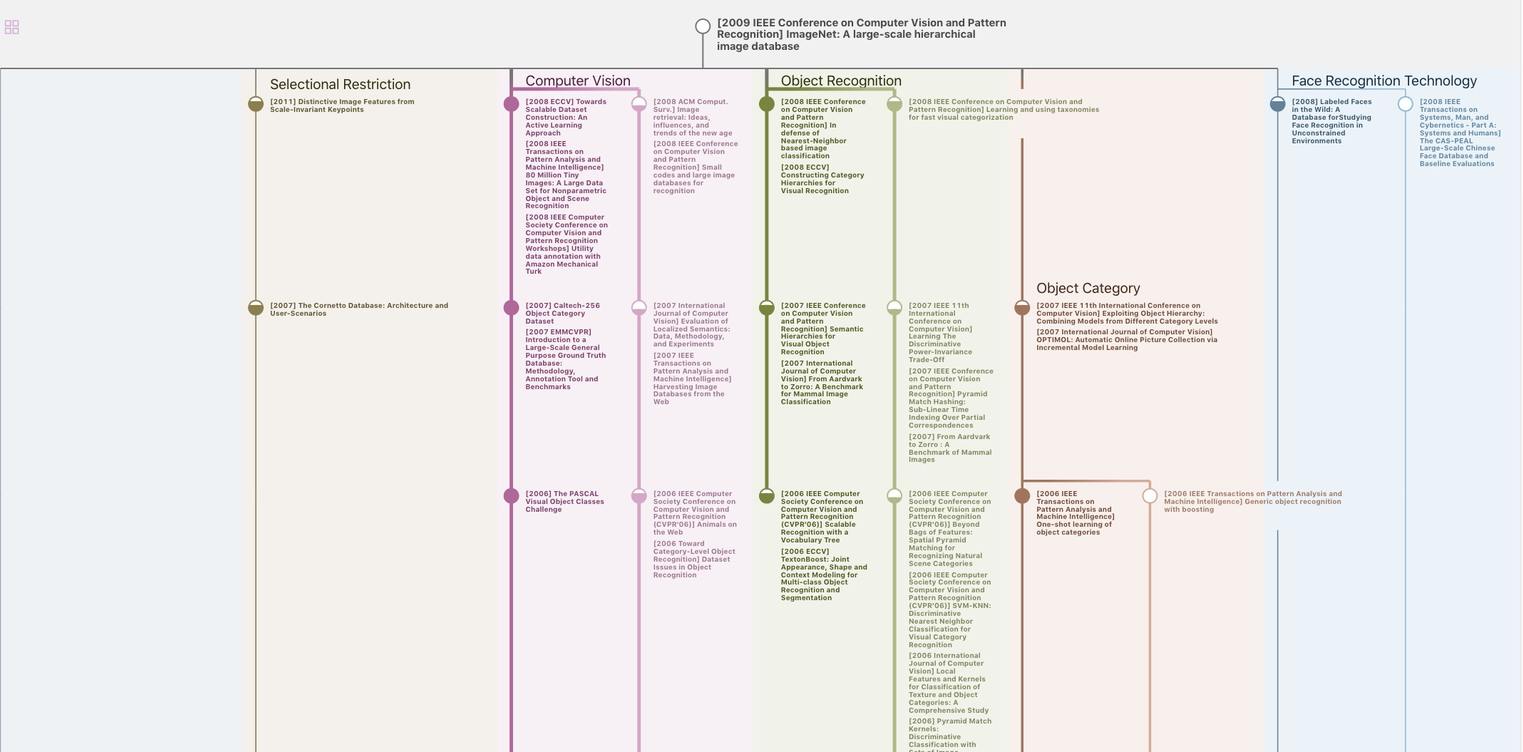
生成溯源树,研究论文发展脉络
Chat Paper
正在生成论文摘要