DarkAI: I. Mapping the large-scale density field of dark matter using AI
arXiv (Cornell University)(2023)
摘要
We develop a deep learning technique to reconstruct the dark matter density field from the redshift-space distribution of dark matter halos. We implement a UNet-architecture neural network and successfully trained it using the COLA fast simulation, which is an approximation of the N-body simulation with $512^3$ particles in a box size of $500 h^{-1}{\rm {Mpc}}$. We evaluate the resulting UNet model not only using the training-like test samples, but also using the typical N-body simulations, including the Jiutian simulation which has $6144^3$ particles in a box size of $1000 h^{-1}{\rm {Mpc}}$, and the ELUCID simulation which has a different cosmology. The real-space dark matter density fields in the three simulations can all be recovered consistently with only a small reduction of the cross-correlation power spectrum at 1\% and 10\% levels at $k=0.1$ and $0.3~h\mathrm{Mpc^{-1}}$, respectively. It is evident that the reconstruction helps to correct for the redshift-space distortions and is unaffected by the different cosmologies between the training sample ({\bf Planck2018}) and the test sample ({\bf WMAP5}). In addition, we tested the application of the UNet-reconstructed density field to recover the velocity \& tidal field and found it outperforms the traditional approach based on the linear bias model, showing a 12.2 percent improvement in the correlation slope and a 21.1 percent reduction in the scatter between the predicted and the true velocities. As a result, our method is highly efficient and has an outstanding level of extrapolation reliability beyond the training set. This offers an optimal solution that determines the three-dimensional underlying density field from the abundant galaxy survey data.
更多查看译文
关键词
darkai matter,mapping,density,large-scale
AI 理解论文
溯源树
样例
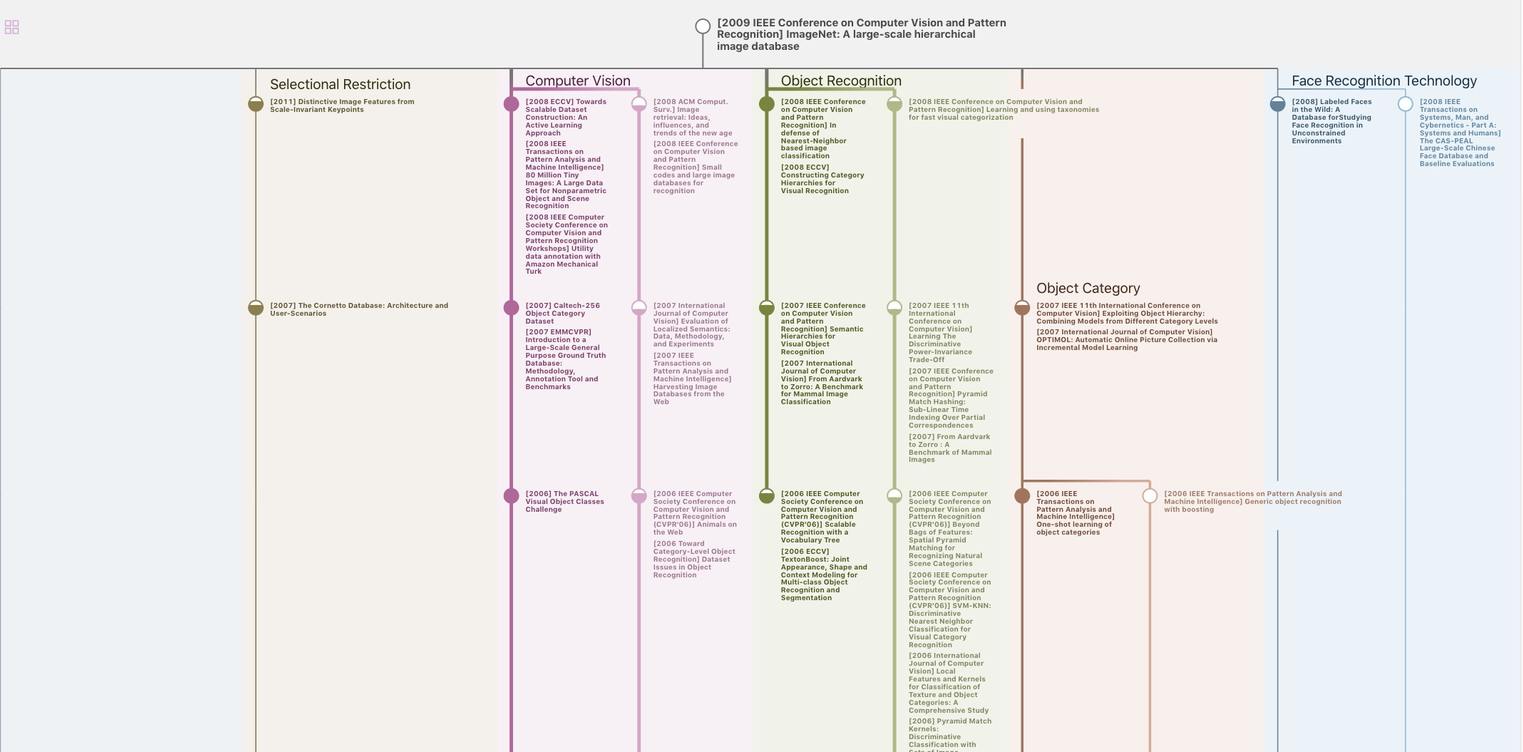
生成溯源树,研究论文发展脉络
Chat Paper
正在生成论文摘要