CM-MaskSD: Cross-Modality Masked Self-Distillation for Referring Image Segmentation
IEEE TRANSACTIONS ON MULTIMEDIA(2024)
摘要
Referring image segmentation (RIS) is a fundamental vision-language task that intends to segment a desired object from an image based on a given natural language expression. Due to the essentially distinct data properties between image and text, most of existing methods either introduce complex designs towards fine-grained vision-language alignment or lack required dense alignment, resulting in scalability issues or mis-segmentation problems such as over- or under-segmentation. To achieve effective and efficient fine-grained feature alignment in the RIS task, we explore the potential of masked multimodal modeling coupled with self-distillation and propose a novel cross-modality masked self-distillation framework named CM-MaskSD, in which our method inherits the transferred knowledge of image-text semantic alignment from CLIP model to realize fine-grained patch-word feature alignment for better segmentation accuracy. Moreover, our CM-MaskSD framework can considerably boost model performance in a nearly parameter-free manner, since it shares weights between the main segmentation branch and the introduced masked self-distillation branches, and solely introduces negligible parameters for coordinating the multimodal features. Comprehensive experiments on three benchmark datasets (i.e. RefCOCO, RefCOCO+, G-Ref) for the RIS task convincingly demonstrate the superiority of our proposed framework over previous state-of-the-art methods.
更多查看译文
关键词
Referring image segmentation,cross-modality guidance,masked self-distillation,vision and language
AI 理解论文
溯源树
样例
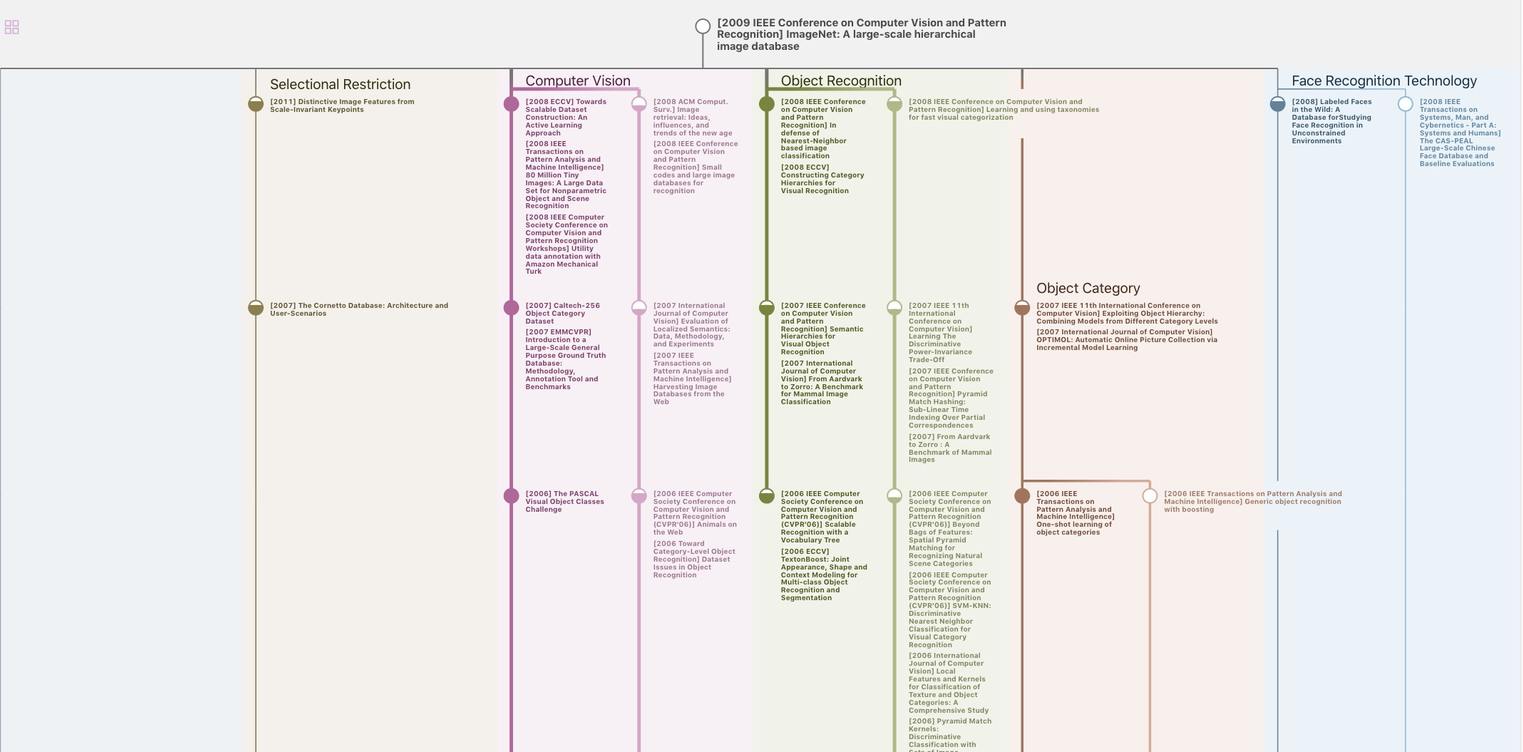
生成溯源树,研究论文发展脉络
Chat Paper
正在生成论文摘要