Moment Matching Denoising Gibbs Sampling
arXiv (Cornell University)(2023)
摘要
Energy-Based Models (EBMs) offer a versatile framework for modeling complex data distributions. However, training and sampling from EBMs continue to pose significant challenges. The widely-used Denoising Score Matching (DSM) method for scalable EBM training suffers from inconsistency issues, causing the energy model to learn a `noisy' data distribution. In this work, we propose an efficient sampling framework: (pseudo)-Gibbs sampling with moment matching, which enables effective sampling from the underlying clean model when given a `noisy' model that has been well-trained via DSM. We explore the benefits of our approach compared to related methods and demonstrate how to scale the method to high-dimensional datasets.
更多查看译文
关键词
sampling,matching
AI 理解论文
溯源树
样例
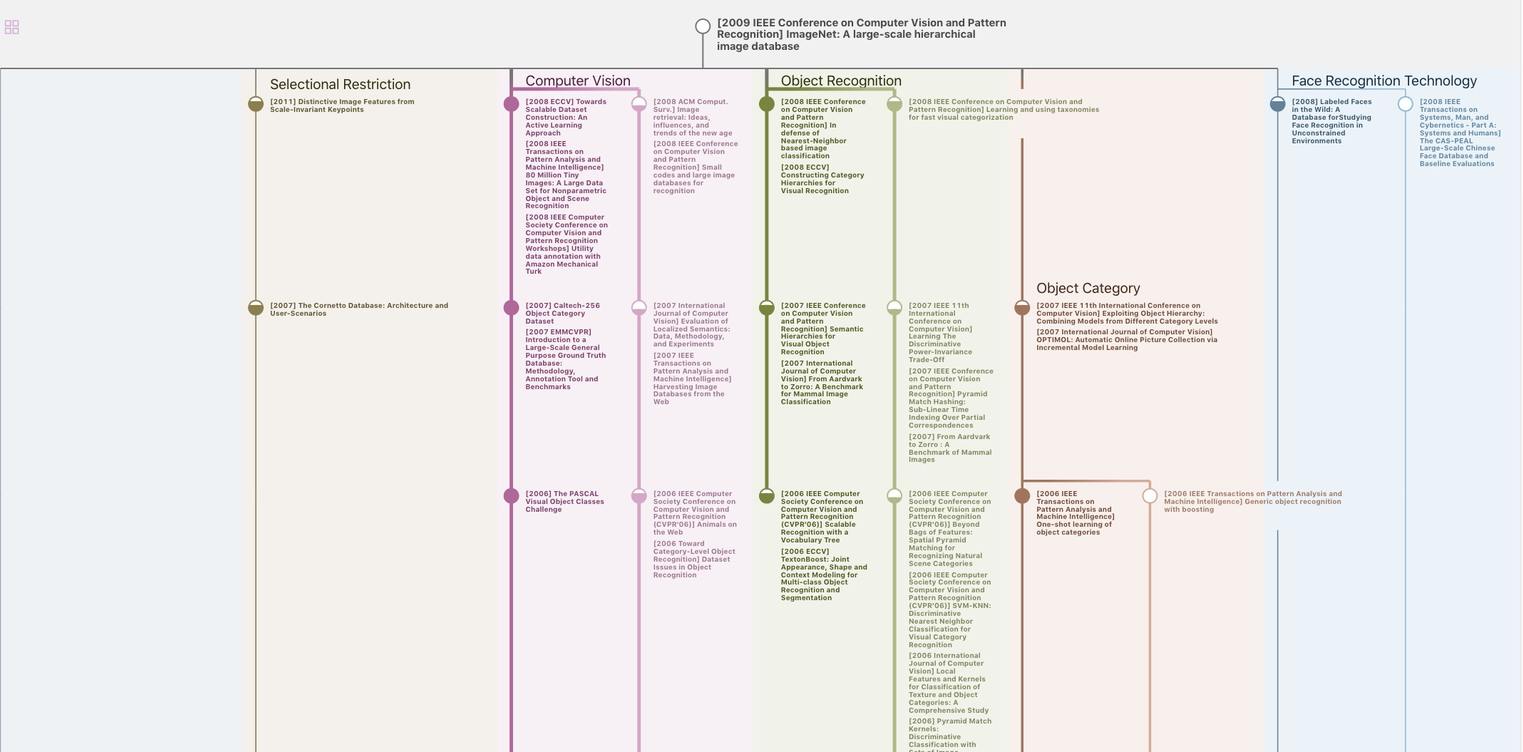
生成溯源树,研究论文发展脉络
Chat Paper
正在生成论文摘要