Structured factorization for single-cell gene expression data
arXiv (Cornell University)(2023)
摘要
Single-cell gene expression data are often characterized by large matrices, where the number of cells may be lower than the number of genes of interest. Factorization models have emerged as powerful tools to condense the available information through a sparse decomposition into lower rank matrices. In this work, we adapt and implement a recent Bayesian class of generalized factor models to count data and, specifically, to model the covariance between genes. The developed methodology also allows one to include exogenous information within the prior, such that recognition of covariance structures between genes is favoured. In this work, we use biological pathways as external information to induce sparsity patterns within the loadings matrix. This approach facilitates the interpretation of loadings columns and the corresponding latent factors, which can be regarded as unobserved cell covariates. We demonstrate the effectiveness of our model on single-cell RNA sequencing data obtained from lung adenocarcinoma cell lines, revealing promising insights into the role of pathways in characterizing gene relationships and extracting valuable information about unobserved cell traits.
更多查看译文
关键词
structured factorization,single-cell single-cell,gene expression
AI 理解论文
溯源树
样例
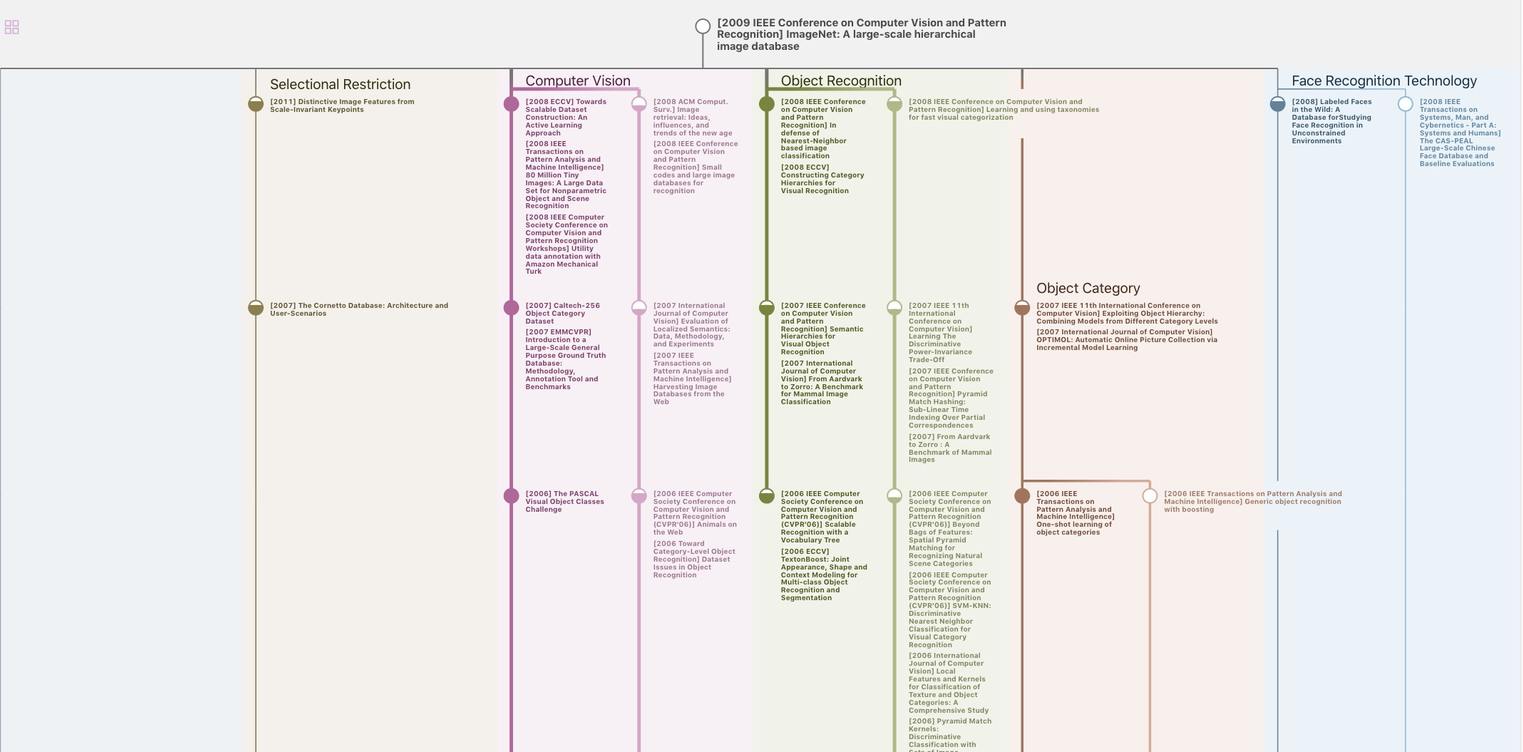
生成溯源树,研究论文发展脉络
Chat Paper
正在生成论文摘要