Self-Reinforcement Attention Mechanism For Tabular Learning
CoRR(2023)
摘要
Apart from the high accuracy of machine learning models, what interests many researchers in real-life problems (e.g., fraud detection, credit scoring) is to find hidden patterns in data; particularly when dealing with their challenging imbalanced characteristics. Interpretability is also a key requirement that needs to accompany the used machine learning model. In this concern, often, intrinsically interpretable models are preferred to complex ones, which are in most cases black-box models. Also, linear models are used in some high-risk fields to handle tabular data, even if performance must be sacrificed. In this paper, we introduce Self-Reinforcement Attention (SRA), a novel attention mechanism that provides a relevance of features as a weight vector which is used to learn an intelligible representation. This weight is then used to reinforce or reduce some components of the raw input through element-wise vector multiplication. Our results on synthetic and real-world imbalanced data show that our proposed SRA block is effective in end-to-end combination with baseline models.
更多查看译文
关键词
tabular learning,attention,mechanism,self-reinforcement
AI 理解论文
溯源树
样例
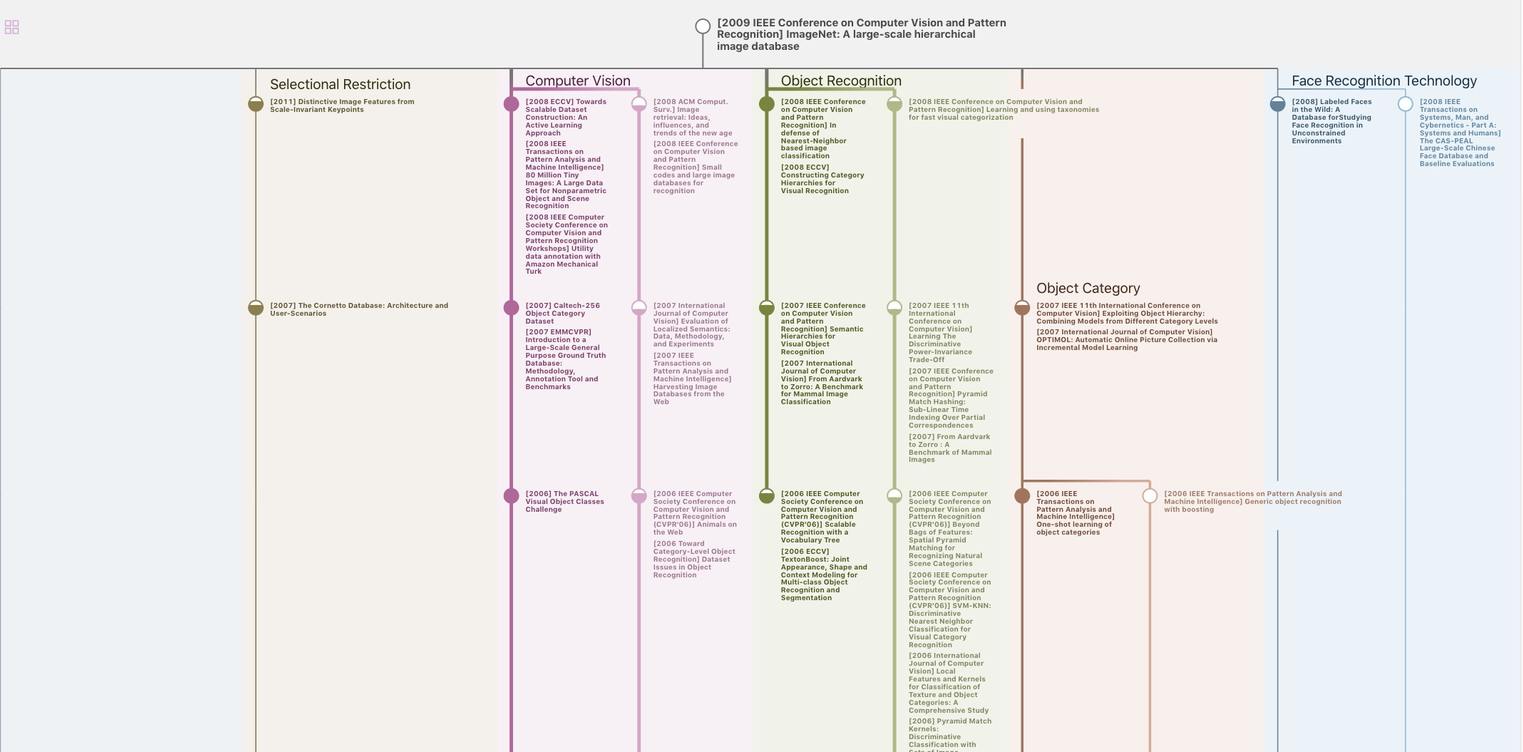
生成溯源树,研究论文发展脉络
Chat Paper
正在生成论文摘要