S-JEA: Stacked Joint Embedding Architectures for Self-Supervised Visual Representation Learning
CoRR(2023)
摘要
The recent emergence of Self-Supervised Learning (SSL) as a fundamental paradigm for learning image representations has, and continues to, demonstrate high empirical success in a variety of tasks. However, most SSL approaches fail to learn embeddings that capture hierarchical semantic concepts that are separable and interpretable. In this work, we aim to learn highly separable semantic hierarchical representations by stacking Joint Embedding Architectures (JEA) where higher-level JEAs are input with representations of lower-level JEA. This results in a representation space that exhibits distinct sub-categories of semantic concepts (e.g., model and colour of vehicles) in higher-level JEAs. We empirically show that representations from stacked JEA perform on a similar level as traditional JEA with comparative parameter counts and visualise the representation spaces to validate the semantic hierarchies.
更多查看译文
关键词
visual
AI 理解论文
溯源树
样例
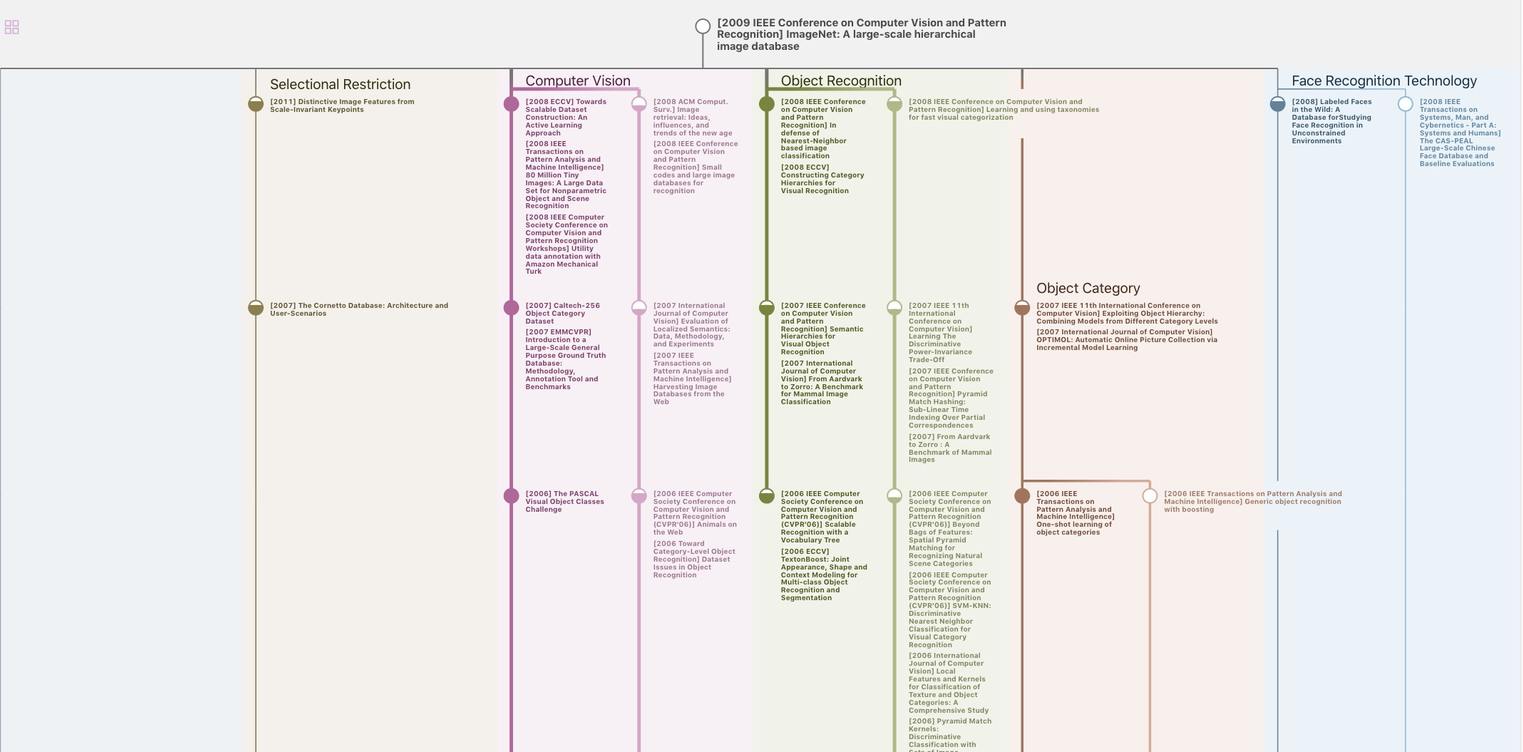
生成溯源树,研究论文发展脉络
Chat Paper
正在生成论文摘要