Photo-zSNthesis: Converting Type Ia Supernova Lightcurves to Redshift Estimates via Deep Learning
CoRR(2023)
摘要
Upcoming photometric surveys will discover tens of thousands of Type Ia supernovae (SNe Ia), vastly outpacing the capacity of our spectroscopic resources. In order to maximize the science return of these observations in the absence of spectroscopic information, we must accurately extract key parameters, such as SN redshifts, with photometric information alone. We present Photo-zSNthesis, a convolutional neural network-based method for predicting full redshift probability distributions from multi-band supernova lightcurves, tested on both simulated Sloan Digital Sky Survey (SDSS) and Vera C. Rubin Legacy Survey of Space and Time (LSST) data as well as observed SDSS SNe. We show major improvements over predictions from existing methods on both simulations and real observations as well as minimal redshift-dependent bias, which is a challenge due to selection effects, e.g. Malmquist bias. The PDFs produced by this method are well-constrained and will maximize the cosmological constraining power of photometric SNe Ia samples.
更多查看译文
关键词
type ia supernova lightcurves,deep learning,photo-zsnthesis
AI 理解论文
溯源树
样例
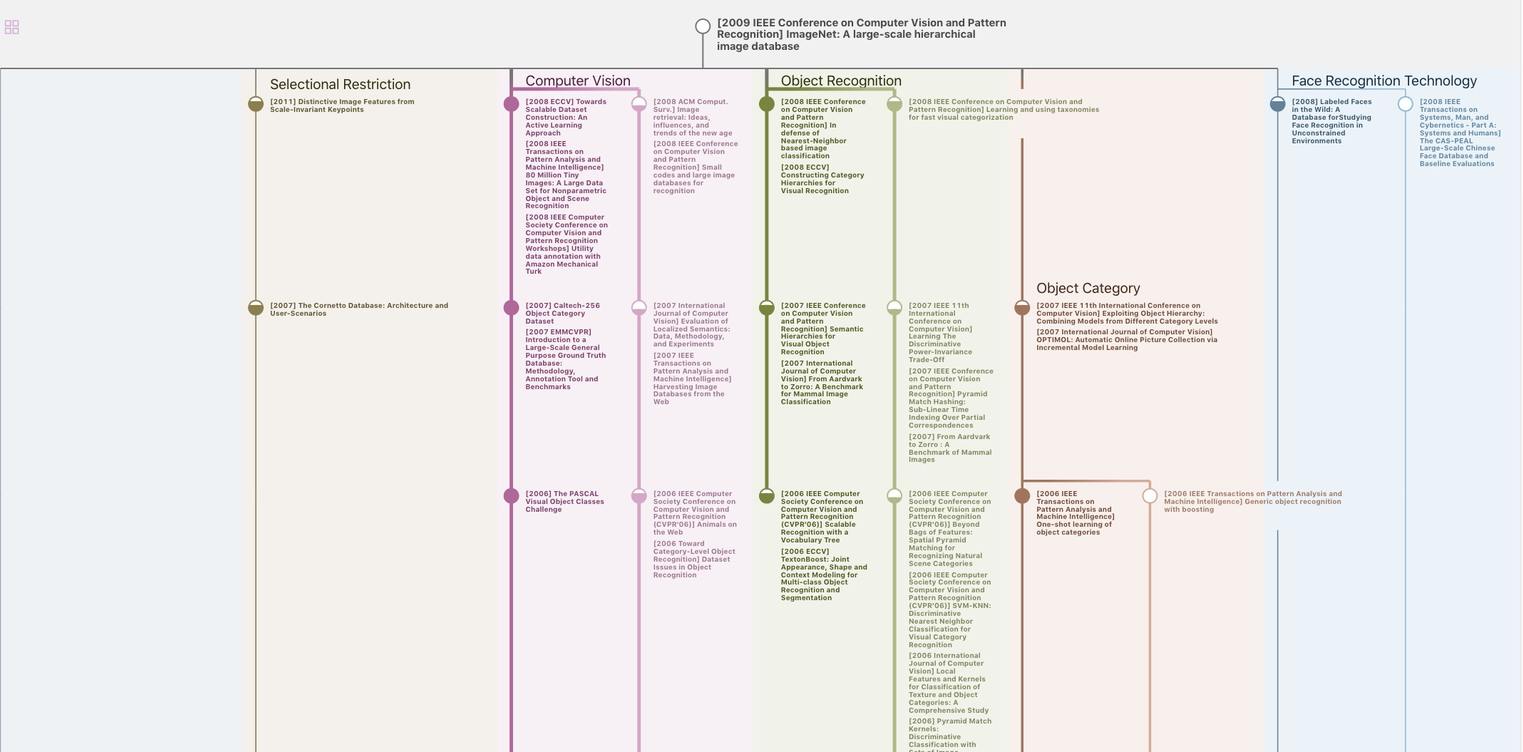
生成溯源树,研究论文发展脉络
Chat Paper
正在生成论文摘要