Object detection method based on CIoU improved bounding box loss function
Chinese Journal of Liquid Crystals and Displays(2023)
摘要
The loss function plays an important role in the detection accuracy and model convergence speed of the object detection task,and the bounding box loss function in the loss function is an important factor affecting the detection results and model convergence speed. To address the problems of low accuracy for traditional model localization and slow convergence of the model during training,an improved bounding box loss function is proposed based on the CIoU bounding box loss function,which solves the problem of gradient explosion and early degradation of the model brought by the bounding box aspect ratio during the derivation of CIoU loss function,and introduces the normalized distance between the aspect relationship and centroid of the overlap region and the target box as additional penalty terms to improve the detection accuracy and convergence speed of the model,which is called BCIoU(Better CIoU). Experimental results on the PASACL VOC 2007 dataset show that the improved BCIoU bounding box loss function improves the IoU loss mAP50 metric by 2. 09% and the AP metric by 6. 88% relatively under the YOLOv3 network,the CIoU loss mAP50 metric improves by 1. 64% and the AP metric improves by 5. 35%. The convergence speed of the model is also improved to some extent. The proposed BCIoU loss function improves detection accuracy and convergence speed of the model,and can be easily incorporated into current object detection algorithms.
更多查看译文
关键词
detection,ciou,box,object
AI 理解论文
溯源树
样例
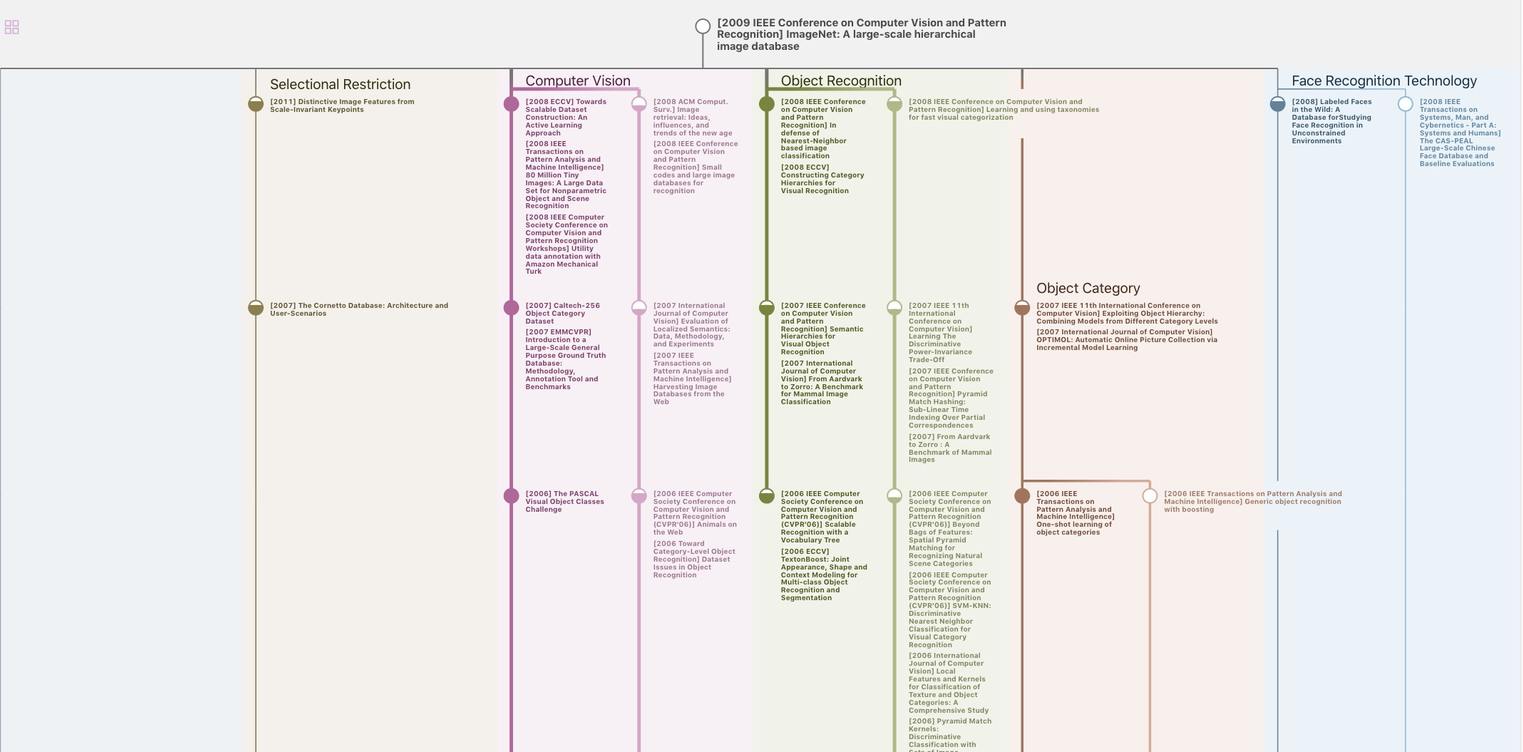
生成溯源树,研究论文发展脉络
Chat Paper
正在生成论文摘要