Repurposing Non-pharmacological Interventions for Alzheimer's Diseases through Link Prediction on Biomedical Literature
medRxiv : the preprint server for health sciences(2023)
摘要
Recently, computational drug repurposing has emerged as a promising method for identifying new pharmaceutical interventions (PI) for Alzheimer's Disease (AD). Non-pharmaceutical interventions (NPI), such as Vitamin E and Music therapy, have great potential to improve cognitive function and slow the progression of AD, but have largely been unexplored. This study predicts novel NPIs for AD through link prediction on our developed biomedical knowledge graph. We constructed a comprehensive knowledge graph containing AD concepts and various potential interventions, called ADInt, by integrating a dietary supplement domain knowledge graph, SuppKG, with semantic relations from SemMedDB database. Four knowledge graph embedding models (TransE, RotatE, DistMult and ComplEX) and two graph convolutional network models (R-GCN and CompGCN) were compared to learn the representation of ADInt. R-GCN outperformed other models by evaluating on the time slice test set and the clinical trial test set and was used to generate the score tables of the link prediction task. Discovery patterns were applied to generate mechanism pathways for high scoring triples. Our ADInt had 162,213 nodes and 1,017,319 edges. The graph convolutional network model, R-GCN, performed best in both the Time Slicing test set (MR = 7.099, MRR = 0.5007, Hits@1 = 0.4112, Hits@3 = 0.5058, Hits@10 = 0.6804) and the Clinical Trials test set (MR = 1.731, MRR = 0.8582, Hits@1 = 0.7906, Hits@3 = 0.9033, Hits@10 = 0.9848). Among high scoring triples in the link prediction results, we found the plausible mechanism pathways of (Photodynamic therapy, PREVENTS, Alzheimer's Disease) and (Choerospondias axillaris, PREVENTS, Alzheimer's Disease) by discovery patterns and discussed them further. In conclusion, we presented a novel methodology to extend an existing knowledge graph and discover NPIs (dietary supplements (DS) and complementary and integrative health (CIH)) for AD. We used discovery patterns to find mechanisms for predicted triples to solve the poor interpretability of artificial neural networks. Our method can potentially be applied to other clinical problems, such as discovering drug adverse reactions and drug-drug interactions.
### Competing Interest Statement
The authors have declared no competing interest.
### Funding Statement
Research reported in this publication was supported by the National Institutes of Health (NIH)/National Institute On Aging (NIA) under Award Number R01AG078154 (PI: RZ) and the NIH/National Center For Complementary & Integrative Health (NCCIH) under Award Number R01AT00945 (PI: RZ). The content is solely the responsibility of the authors and does not necessarily represent the official views of the NIH. GD has received financial support for his mobility to UoM from the French State in the frame of the "Investments for the future" Programme IdEx Bordeaux, reference ANR-10-IDEX-03-02.
### Author Declarations
I confirm all relevant ethical guidelines have been followed, and any necessary IRB and/or ethics committee approvals have been obtained.
Yes
I confirm that all necessary patient/participant consent has been obtained and the appropriate institutional forms have been archived, and that any patient/participant/sample identifiers included were not known to anyone (e.g., hospital staff, patients or participants themselves) outside the research group so cannot be used to identify individuals.
Yes
I understand that all clinical trials and any other prospective interventional studies must be registered with an ICMJE-approved registry, such as ClinicalTrials.gov. I confirm that any such study reported in the manuscript has been registered and the trial registration ID is provided (note: if posting a prospective study registered retrospectively, please provide a statement in the trial ID field explaining why the study was not registered in advance).
Yes
I have followed all appropriate research reporting guidelines, such as any relevant EQUATOR Network research reporting checklist(s) and other pertinent material, if applicable.
Yes
ADInt knowledge graph data is available in the following google drive: https://drive.google.com/drive/folders/187HnI2d-RRFeYk\_C7MYSCHtVX-6IuNVS?usp=sharing. The complete SemMedDB database can be accessed directly on https://lhncbc.nlm.nih.gov/ii/tools/SemRep\_SemMedDB_SKR.html.
[https://lhncbc.nlm.nih.gov/ii/tools/SemRep\_SemMedDB\_SKR.html][1]
[1]: https://lhncbc.nlm.nih.gov/ii/tools/SemRep_SemMedDB_SKR.html
更多查看译文
关键词
alzheimers,link prediction,interventions,non-pharmacological
AI 理解论文
溯源树
样例
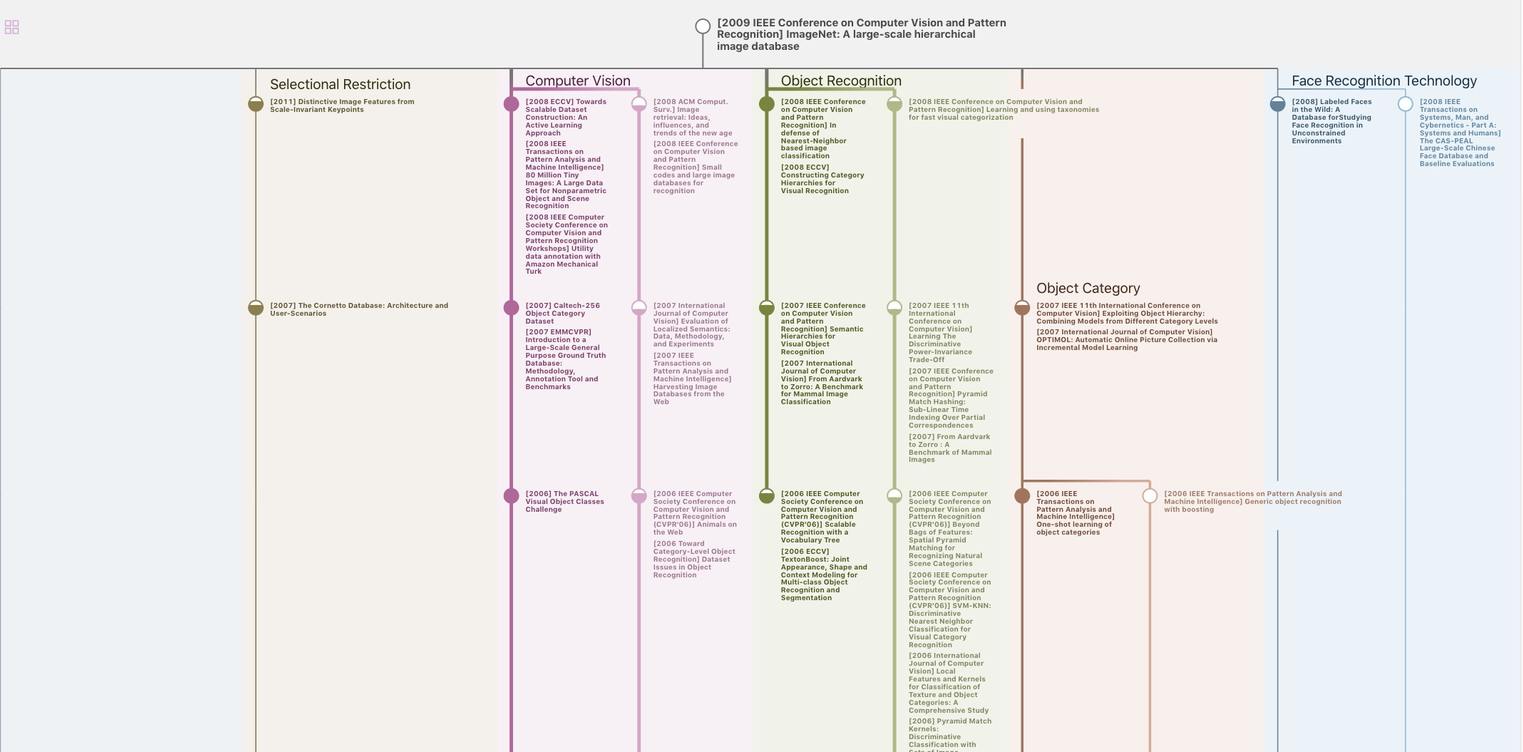
生成溯源树,研究论文发展脉络
Chat Paper
正在生成论文摘要