Bi-VLGM : Bi-Level Class-Severity-Aware Vision-Language Graph Matching for Text Guided Medical Image Segmentation
CoRR(2023)
摘要
Medical reports with substantial information can be naturally complementary to medical images for computer vision tasks, and the modality gap between vision and language can be solved by vision-language matching (VLM). However, current vision-language models distort the intra-model relation and mainly include class information in prompt learning that is insufficient for segmentation task. In this paper, we introduce a Bi-level class-severity-aware Vision-Language Graph Matching (Bi-VLGM) for text guided medical image segmentation, composed of a word-level VLGM module and a sentence-level VLGM module, to exploit the class-severity-aware relation among visual-textual features. In word-level VLGM, to mitigate the distorted intra-modal relation during VLM, we reformulate VLM as graph matching problem and introduce a vision-language graph matching (VLGM) to exploit the high-order relation among visual-textual features. Then, we perform VLGM between the local features for each class region and class-aware prompts to bridge their gap. In sentence-level VLGM, to provide disease severity information for segmentation task, we introduce a severity-aware prompting to quantify the severity level of retinal lesion, and perform VLGM between the global features and the severity-aware prompts. By exploiting the relation between the local (global) and class (severity) features, the segmentation model can selectively learn the class-aware and severity-aware information to promote performance. Extensive experiments prove the effectiveness of our method and its superiority to existing methods. Source code is to be released.
更多查看译文
关键词
medical image segmentation,image segmentation,bi-vlgm,bi-level,class-severity-aware,vision-language
AI 理解论文
溯源树
样例
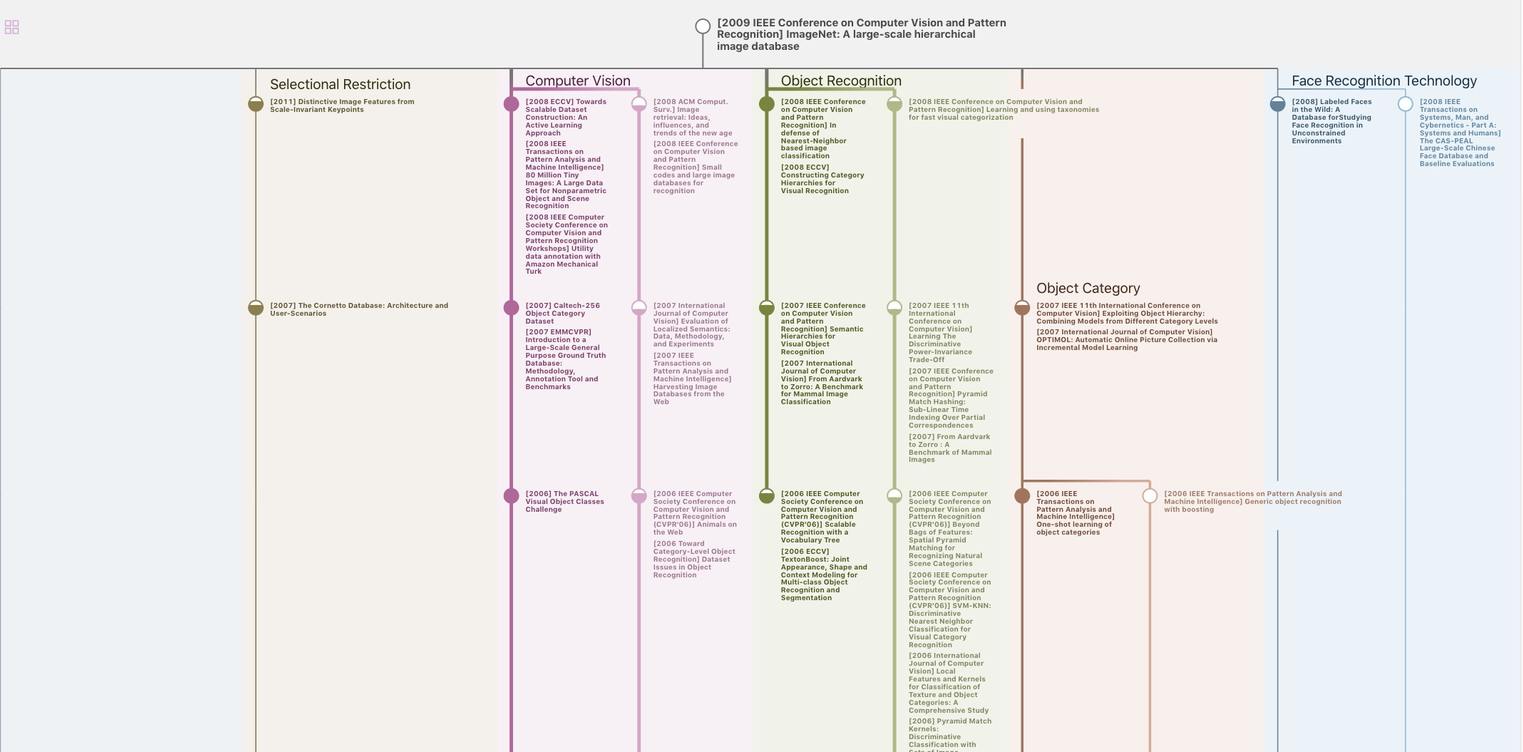
生成溯源树,研究论文发展脉络
Chat Paper
正在生成论文摘要