Joint Feature and Differentiable $ K $-NN Graph Learning Using Dirichlet Energy
NeurIPS 2023(2023)
摘要
Feature selection (FS) plays an important role in machine learning, which extracts important features and accelerates the learning process. In this paper, we propose a deep FS method that simultaneously conducts feature selection and differentiable $ k $-NN graph learning based on the Dirichlet Energy. The Dirichlet Energy identifies important features by measuring their smoothness on the graph structure, and facilitates the learning of a new graph that reflects the inherent structure in new feature subspace. We employ Optimal Transport theory to address the non-differentiability issue of learning $ k $-NN graphs in neural networks, which theoretically makes our method applicable to other graph neural networks for dynamic graph learning. Furthermore, the proposed framework is interpretable, since all modules are designed algorithmically. We validate the effectiveness of our model with extensive experiments on both synthetic and real-world datasets.
更多查看译文
关键词
Feature Selection,Differential k-NN Graph,Dirichlet Energy
AI 理解论文
溯源树
样例
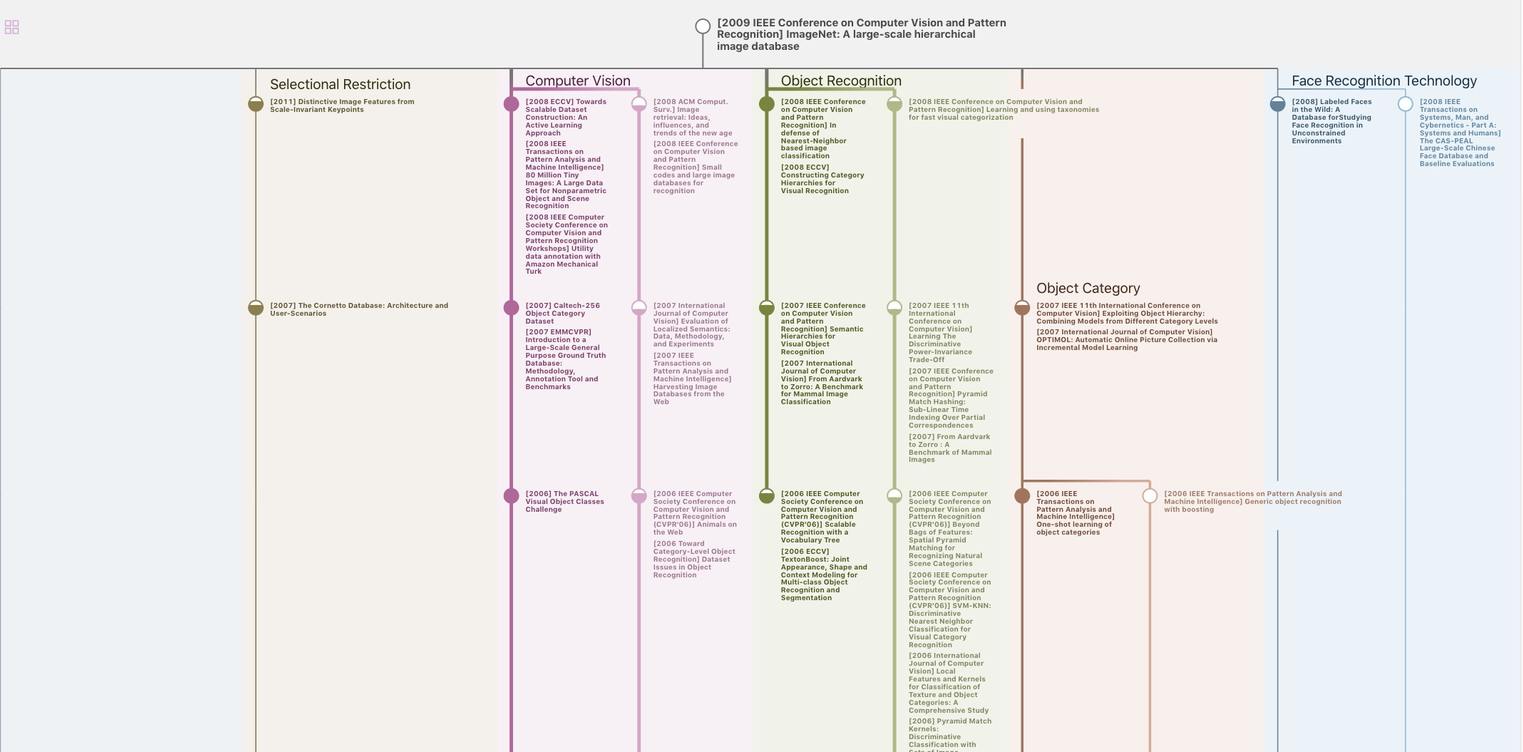
生成溯源树,研究论文发展脉络
Chat Paper
正在生成论文摘要