HighLight: Efficient and Flexible DNN Acceleration with Hierarchical Structured Sparsity
56TH IEEE/ACM INTERNATIONAL SYMPOSIUM ON MICROARCHITECTURE, MICRO 2023(2023)
摘要
Due to complex interactions among various deep neural network (DNN) optimization techniques, modern DNNs can have weights and activations that are dense or sparse with diverse sparsity degrees. To offer a good trade-off between accuracy and hardware performance, an ideal DNN accelerator should have high flexibility to efficiently translate DNN sparsity into reductions in energy and/or latency without incurring significant complexity overhead. This paper introduces hierarchical structured sparsity (HSS), with the key insight that we can systematically represent diverse sparsity degrees by having them hierarchically composed from multiple simple sparsity patterns. As a result, HSS simplifies the underlying hardware since it only needs to support simple sparsity patterns; this significantly reduces the sparsity acceleration overhead, which improves efficiency. Motivated by such opportunities, we propose a simultaneously efficient and flexible accelerator, named HighLight, to accelerate DNNs that have diverse sparsity degrees (including dense). Due to the flexibility of HSS, different HSS patterns can be introduced to DNNs to meet different applications' accuracy requirements. Compared to existing works, HighLight achieves a geomean of up to 6.4x better energy-delay product (EDP) across workloads with diverse sparsity degrees, and always sits on the EDP-accuracy Pareto frontier for representative DNNs.
更多查看译文
关键词
Deep learning accelerator,structured sparsity,hardware-software,co-design,computer architecture
AI 理解论文
溯源树
样例
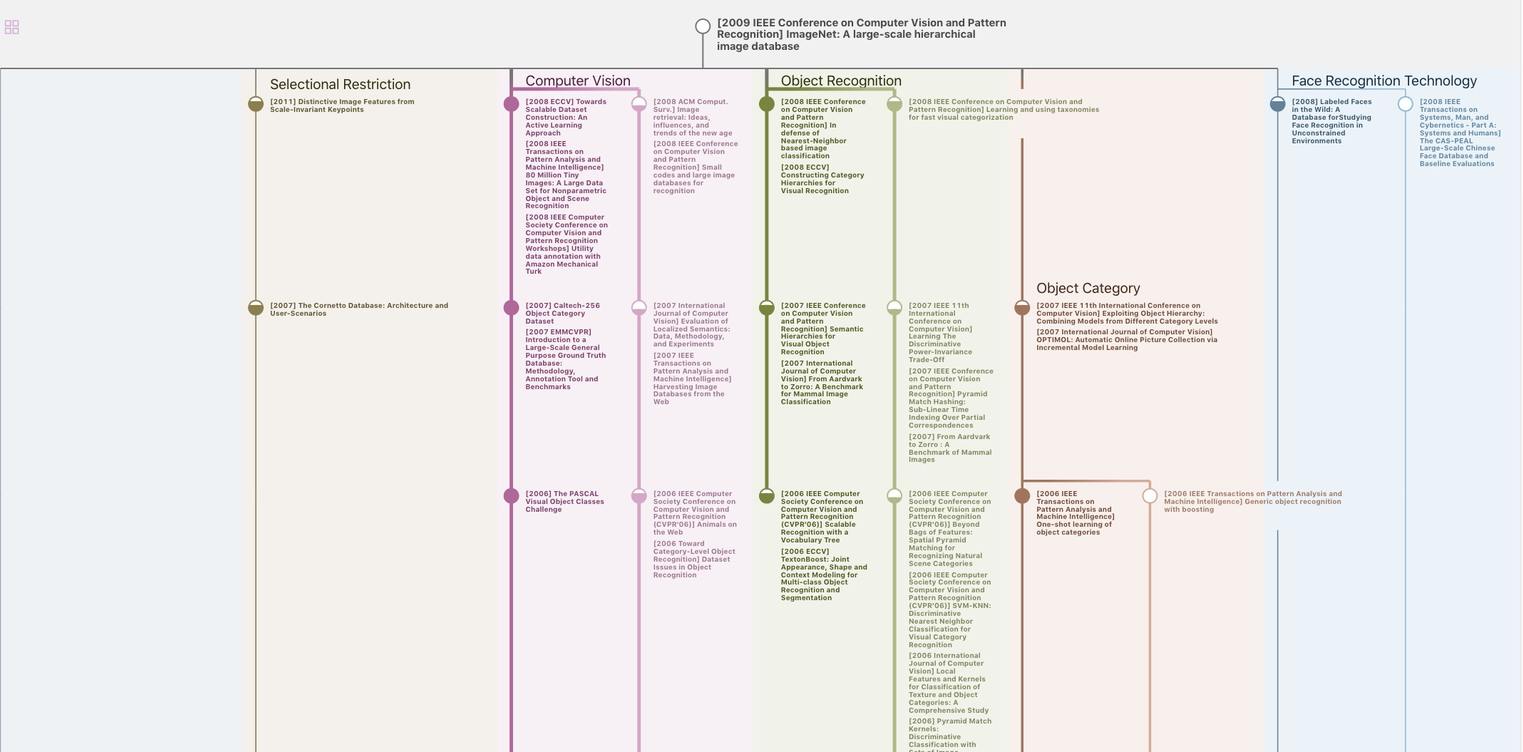
生成溯源树,研究论文发展脉络
Chat Paper
正在生成论文摘要