Explicit Personalization and Local Training: Double Communication Acceleration in Federated Learning
CoRR(2023)
摘要
Federated Learning is an evolving machine learning paradigm, in which multiple clients perform computations based on their individual private data, interspersed by communication with a remote server. A common strategy to curtail communication costs is Local Training, which consists in performing multiple local stochastic gradient descent steps between successive communication rounds. However, the conventional approach to local training overlooks the practical necessity for client-specific personalization, a technique to tailor local models to individual needs. We introduce Scafflix, a novel algorithm that efficiently integrates explicit personalization with local training. This innovative approach benefits from these two techniques, thereby achieving doubly accelerated communication, as we demonstrate both in theory and practice.
更多查看译文
关键词
federated learning,explicit personalization,double communication
AI 理解论文
溯源树
样例
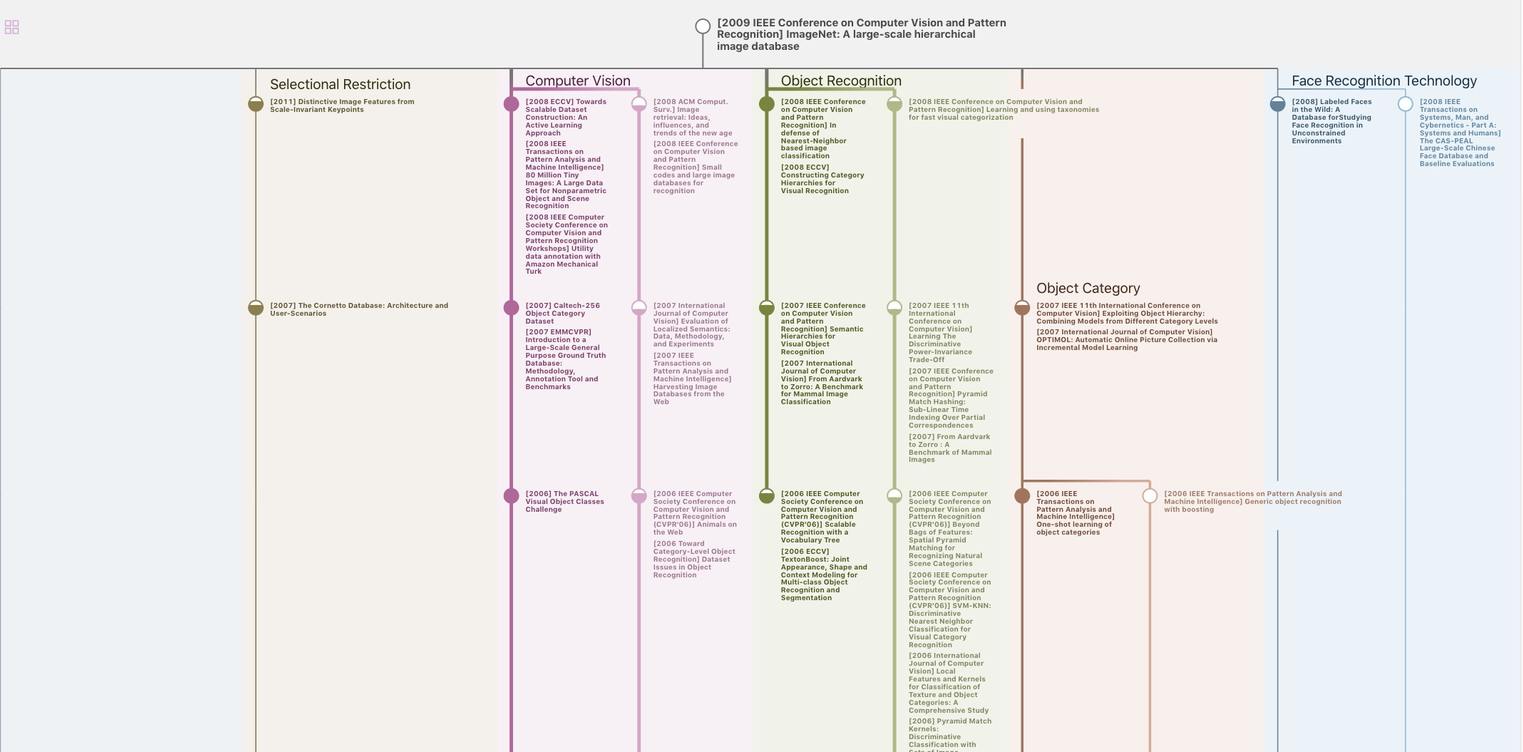
生成溯源树,研究论文发展脉络
Chat Paper
正在生成论文摘要