Battery state-of-health diagnostics during fast cycling using physics-informed deep-learning
JOURNAL OF POWER SOURCES(2023)
摘要
Rapid, in-situ Li-ion battery state-of-health (SOH) quantification is challenging. Li-ion battery aging can vary significantly with chemistry, operating conditions, cycling demands, electrode design, and operation history. As a cell ages, optimal and safe operating conditions need to be adapted to account for battery degradation by tracking critical aging modes such as loss-of-lithium-inventory (LLI), loss-of-active-material (LAM) in either electrode, and/or impedance rise. This manuscript describes a framework for identifying battery aging modes inoperando using fast-rate voltage charge/discharge responses. The framework uses a physically based Li-ion battery model to produce synthetic high-rate responses at aged states. The aging model is calibrated against experimental data from cells with different electrode loadings and cycled under a variety of fast-charging conditions (1 h, 15 min, 10 min, and 7 min charging). The synthetically generated high-rate responses at aged states are then used to train a deep-learning model to identify real cell state-of-health from fast charge/discharge battery voltage responses. The synthetically trained deep-learning model performance is validated by comparing to standard incremental capacity analysis and half-cell measurements. The framework demonstrates the benefits of using high-rate physics-based models to generate synthetic data for training deep-learning models.
更多查看译文
关键词
Li-ion battery model,Machine learning,Synthetic data,In-situ diagnostics
AI 理解论文
溯源树
样例
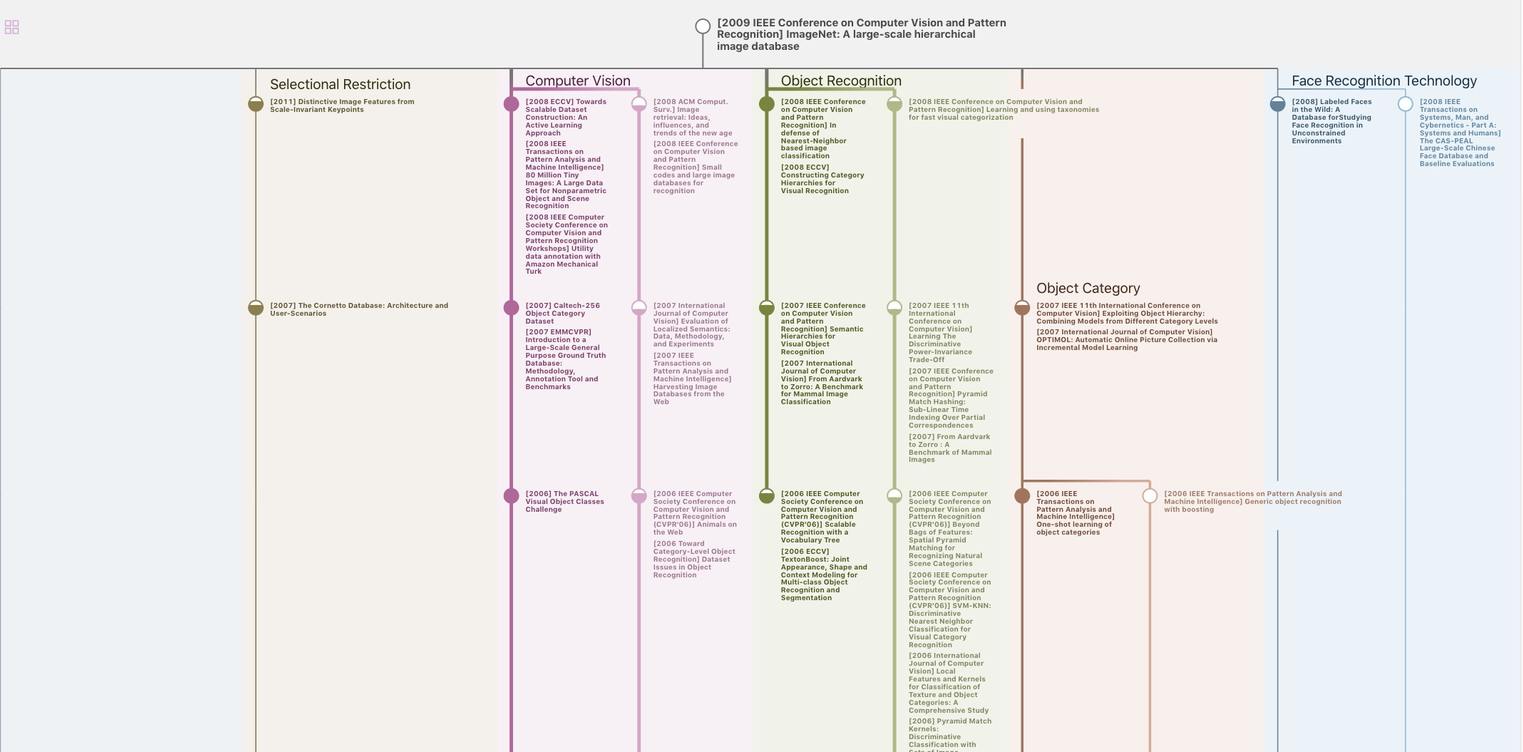
生成溯源树,研究论文发展脉络
Chat Paper
正在生成论文摘要