Prediction of 2-Year Mortality in Patients with Advanced Non-Small Cell Lung Cancer Treated with Immune Checkpoint Inhibitors Based on a Machine Learning Algorithm
Research Square (Research Square)(2023)
Abstract
Abstract Background: Immune checkpoint inhibitors (ICIs) extend the survival time in patients with advanced non-small-cell lung cancer (NSCLC), but not all patients respond to the treatment. However, current immune-related biomarkers, such as programmed death receptor ligand 1 (PD-L1) and tumor mutation burden(TMB) are limited. We created a machine learning (ML) based clinical decision support algorithm to evaluate the 2-Year mortality of NSCLC patients treated with ICIs by comprehensively combining the clinical information. Methods: We collected clinical data, including patient characteristics, mutations and laboratory findings, from the electronic medical records of 345 patients with NSCLC treated with ICIs. Through machine learning and statistical analysis, we constructed effective immune risk score models. Twenty-four clinically meaningful features were used in supervised ML algorithms, including decision trees, random forest and XGBoost , to predict the 2-Year mortality. Results: Several factors, including driver gene mutation status, derived neutrophil to lymphocyte ratio (dNLR), prognostic nutrition index (PNI), could independently predict the 2-Year mortality.ML platforms based on the random forest algorithm using 24 clinical features showed more significant prediction performance (area under the curve [AUC] 0.736). Conclusions: Collectively, our machine learning algorithm offers a clinical decision support model to predict the 2-Year mortality in NSCLC treated with ICIs.
MoreTranslated text
Key words
immune checkpoint inhibitors,lung cancer,machine learning,learning algorithm,non-small
AI Read Science
Must-Reading Tree
Example
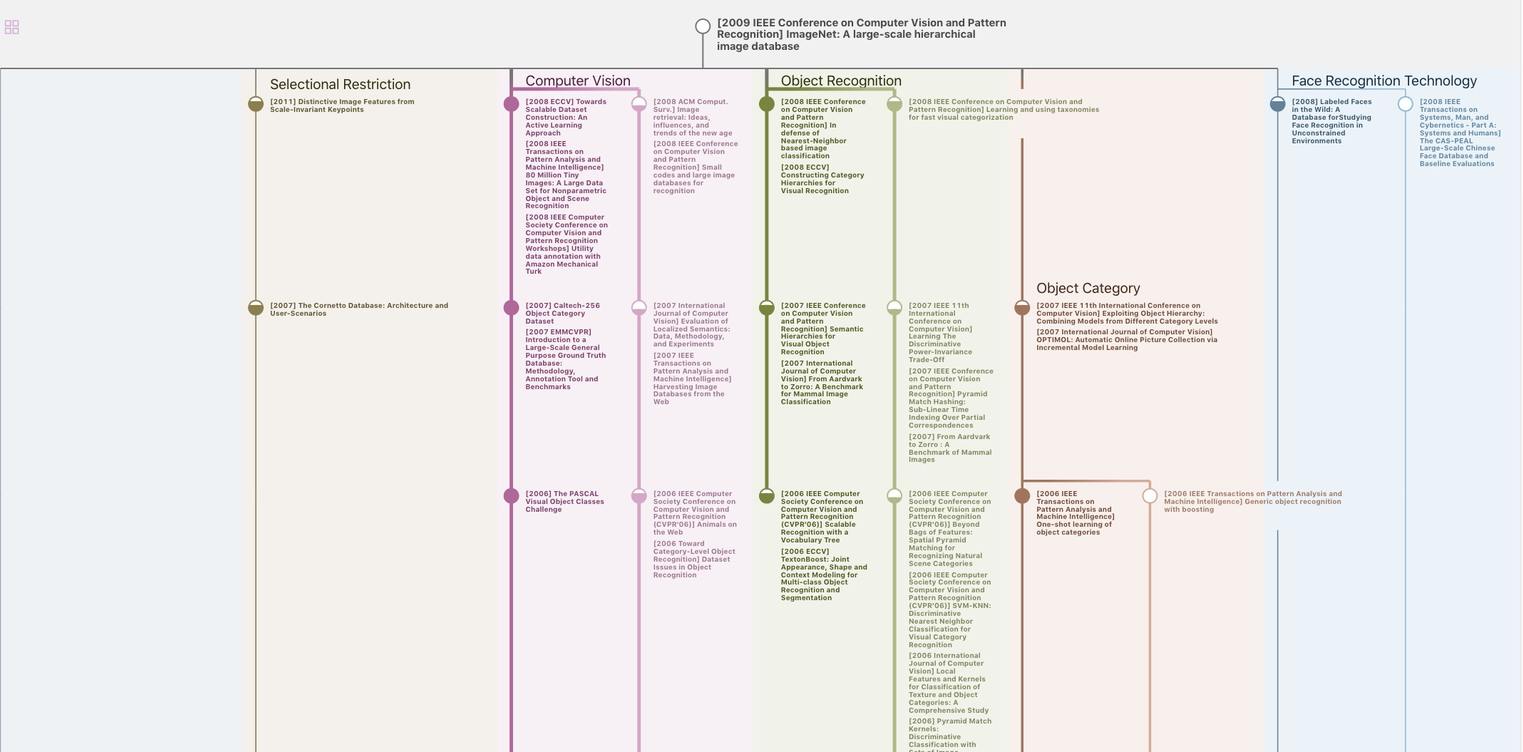
Generate MRT to find the research sequence of this paper
Chat Paper
Summary is being generated by the instructions you defined