An evaluation of ECG data fusion algorithms for wearable IoT sensors
Information Fusion(2023)
摘要
In wearable sensing, accurate estimation of physiological parameters is paramount, although these signals can be corrupted by noise. The fusion of data from multiple sensor sources has the potential to enhance accuracy, even in the presence of disruptive noise. This paper aims to introduce and compare various existing state-of-the-art and novel data fusion techniques to improve the reliability of heart rate estimation. The comparisons were implemented using the MIT-BIH Arrhythmia database with additive noise signals taken from MIT Noise Stress Test Database. When it comes to the challenging low signal-to-noise ratio (SNR) regions, the Kalman fusion and the alpha-trim mean filtering approach exhibits the best performance. The Kalman fusion approach dominates when both channels are corrupted, while the alpha-trim mean filtering elimination algorithm takes the lead when at least one channel is clean. To make the most of these strengths, we have developed an innovative algorithm that can switch between the two fusion methods based on a signal quality indicator (SQI) that serves as a surrogate SNR. This algorithm outperforms the baseline 2-channel RR-interval averaging approach by similar or equal to 54% and similar or equal to 21% at SNRs of 20 dB and -20 dB respectively. Moreover, it outperforms other cutting-edge heart rate estimation methods.
更多查看译文
关键词
ecg data fusion algorithms,wearable iot sensors,data fusion
AI 理解论文
溯源树
样例
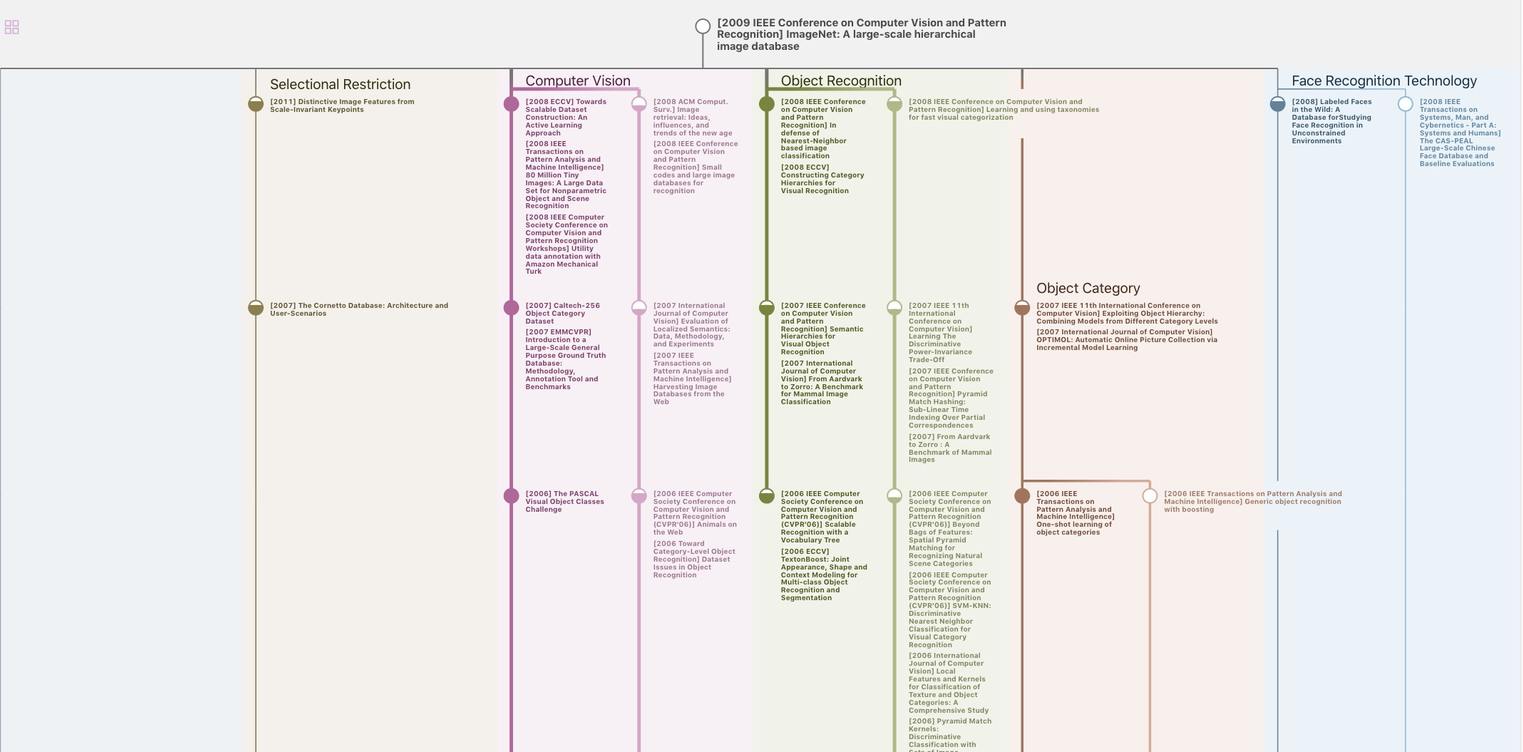
生成溯源树,研究论文发展脉络
Chat Paper
正在生成论文摘要