Spatial-Spectral Siamese and Similarity Network for Hyperspectral Image Classification
Lecture notes in electrical engineering(2023)
摘要
Recently, depth methods have developed rapidly and achieved remarkable achievements in the field of hyperspectral image classification. Deep learning largely depends on high-quality labels. However, for hyperspectral images, obtaining high-quality labels is difficult, expensive and time-consuming. For the problem of the small number of training samples, We propose a Spatial-spectral Siamese network, which randomly selects a number of tasks composed of support sets and query sets on the training set. The network is used to extract spectral and spatial features and fuse them to obtain spatial-spectral features. The fused Spatial-spectral features are trained to optimize the Spatial-spectral Siamese network by contrastive loss, and the similarity loss is used to measure the similarity score between samples to optimize the similarity network. In addition, the cluster labels obtained by clustering are used as supervised information, The performance of network classification is further optimized by monitoring the clustering task and using a large amount of unlabeled sample information. The results of two frequently used hyperspectral data sets show that the proposed method still has good performance and effect in classification accuracy when training samples are very small.
更多查看译文
关键词
hyperspectral image classification,similarity network,spatial-spectral
AI 理解论文
溯源树
样例
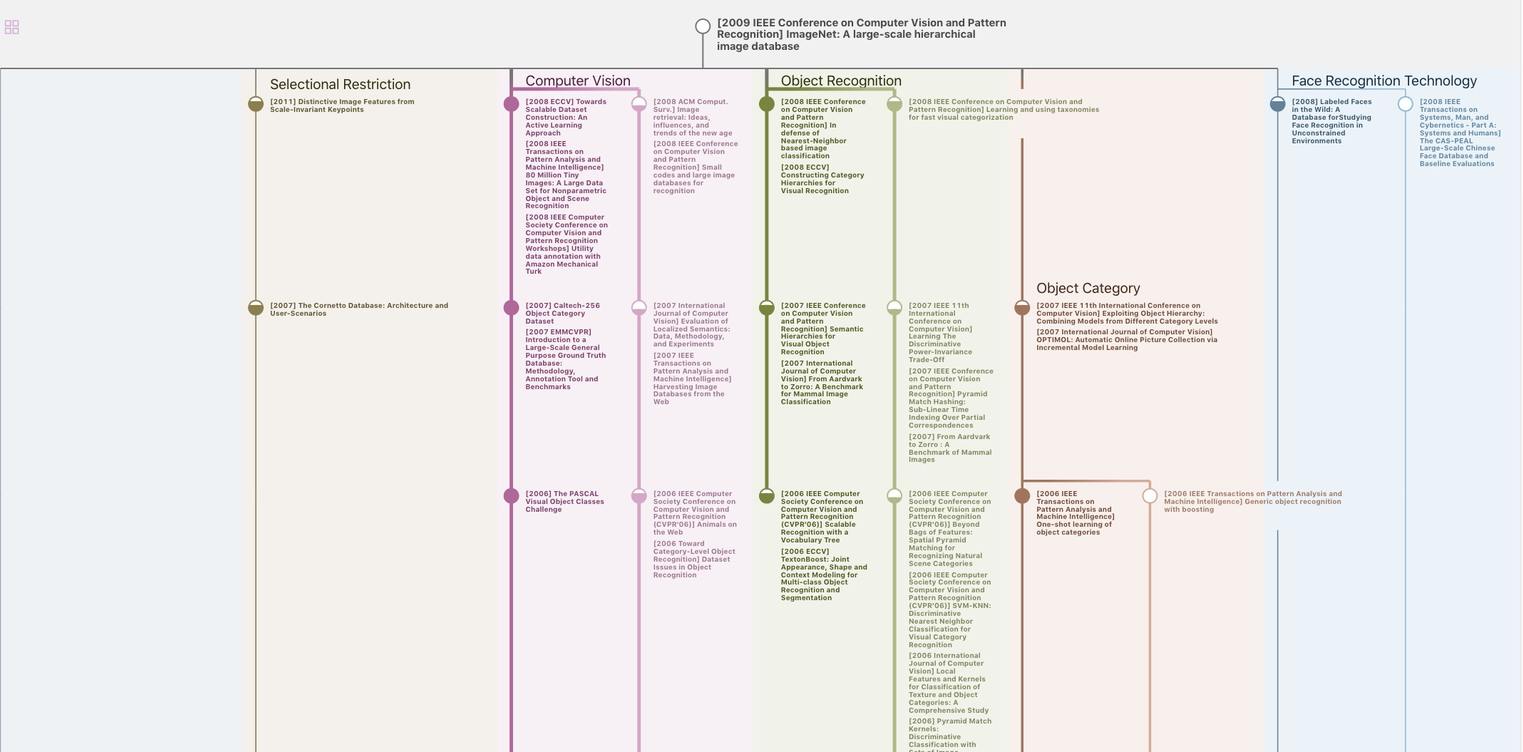
生成溯源树,研究论文发展脉络
Chat Paper
正在生成论文摘要