Gaussian Condensation Filter Based on Cooperative Constrained Particle Flow.
IEEE Internet of Things Journal(2023)
摘要
Real-time high-accuracy localization has a wide range of applications in scenarios, such as pedestrian navigation, emergency rescue, and vehicle networks. In these conditions, the measurement models are often nonlinear, and traditional Kalman and particle filters cannot provide long-time high-precision location-based services. To this end, we propose a Gaussian condensation filter (GCF) algorithm that can achieve high-accuracy localization in a harsh environment. However, aiming at the degradation of sampling points in target tracking based on the GCF, this article proposes a GCF algorithm based on particle flow which transfers the sample points satisfying the prior distribution of the target state to the posterior distribution, thereby improving the practical accuracy of the target-tracking algorithm. Further, to enhance the information fusion in the cooperative network, we propose a multitarget cooperative tracking algorithm to accomplish spatially constrained timing filtering of state information for improving the error correction of the target nodes on timing estimation. Numerical simulations are conducted to determine the effectiveness of our proposed algorithms. Compared with the GCF, its positioning accuracy is improved to 44.6%. Compared with the Gaussian condensation algorithm based on particle flow (PF), the practical accuracy of the GCF algorithm based on cooperative constrained PF in multitarget tracking is improved to 58.1%.
更多查看译文
关键词
Constrained optimization,cumulative error,Gaussian mixture distribution,multitarget tracking,nonlinear filter
AI 理解论文
溯源树
样例
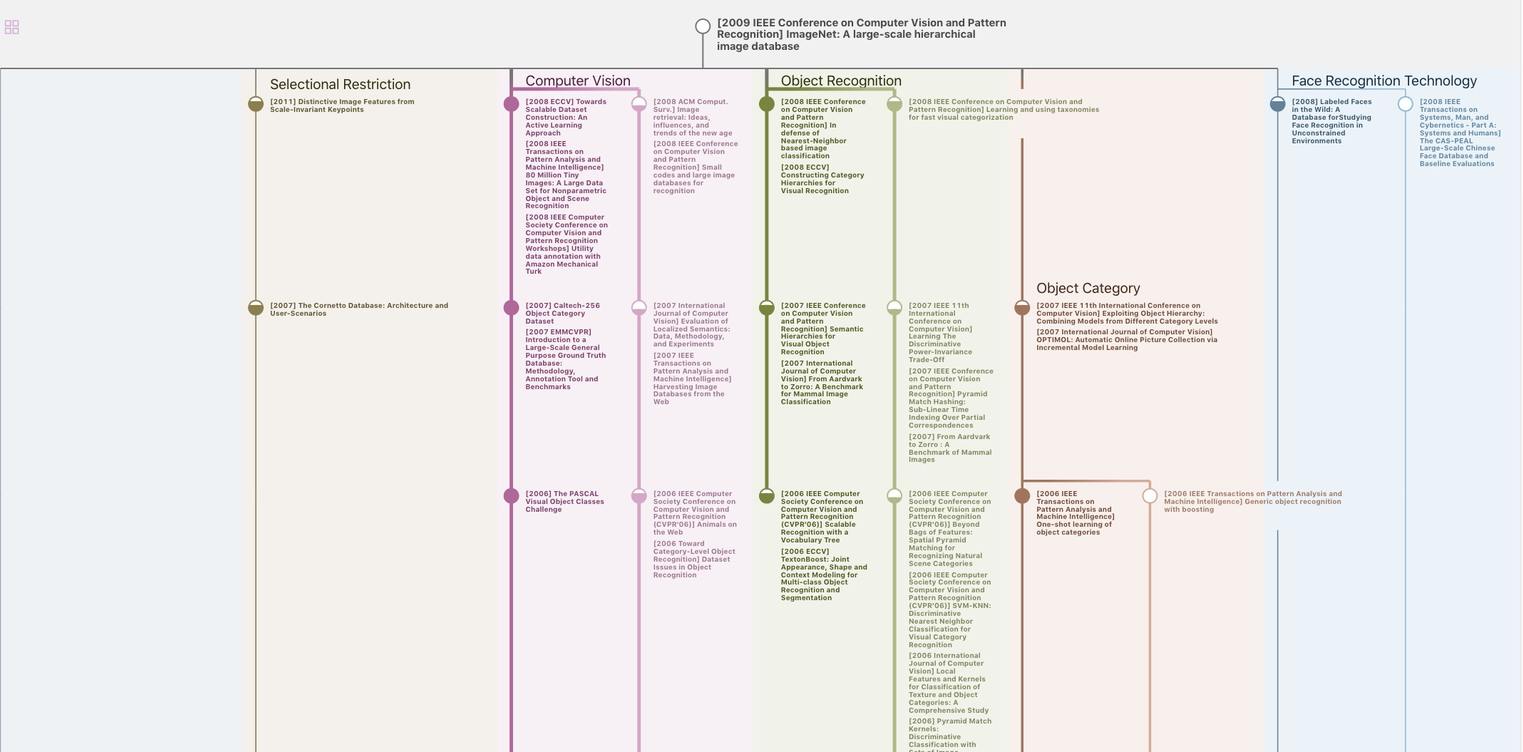
生成溯源树,研究论文发展脉络
Chat Paper
正在生成论文摘要