Examination of machine learning for assessing physical effects: Learning the relativistic continuum mass table with kernel ridge regression*
Chinese Physics C(2023)
摘要
The kernel ridge regression (KRR) method and its extension with odd-even effects (KRRoe) are used to learn the nuclear mass table obtained by the relativistic continuum Hartree-Bogoliubov theory. With respect to the binding energies of 9035 nuclei, the KRR method achieves a root-mean-square deviation of 0.96 MeV, and the KRRoe method remarkably reduces the deviation to 0.17 MeV. By investigating the shell effects, one-nucleon and two-nucleon separation energies, odd-even mass differences, and empirical proton-neutron interactions extracted from the learned binding energies, the ability of the machine learning tool to grasp the known physics is discussed. It is found that the shell effects, evolutions of nucleon separation energies, and empirical proton-neutron interactions are well reproduced by both the KRR and KRRoe methods, although the odd-even mass differences can only be reproduced by the KRRoe method.
更多查看译文
关键词
kernel ridge regression*,physical effects,machine learning
AI 理解论文
溯源树
样例
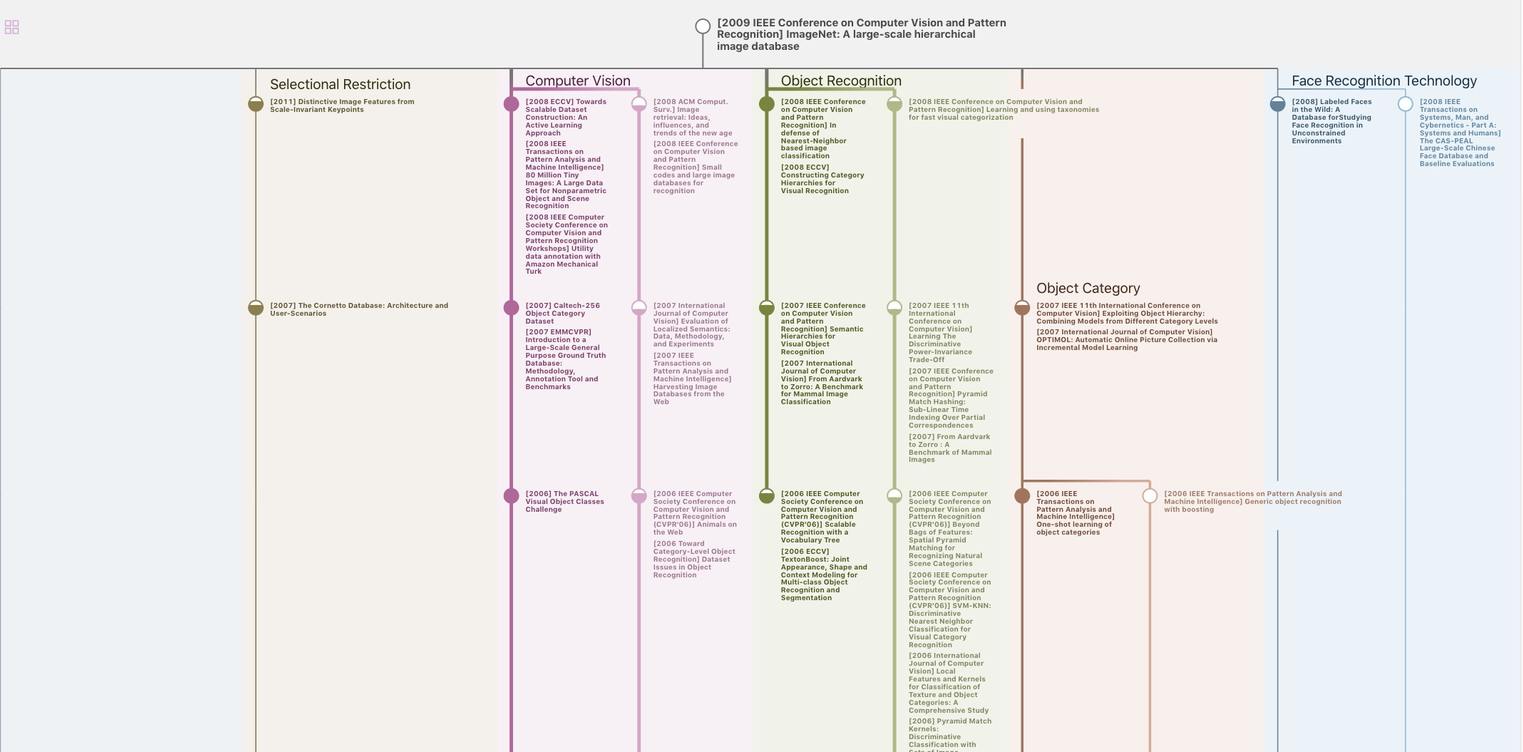
生成溯源树,研究论文发展脉络
Chat Paper
正在生成论文摘要