Corruption depth: Analysis of DNN depth for misclassification
NEURAL NETWORKS(2024)
摘要
Many large and complex deep neural networks have been shown to provide higher performance on various computer vision tasks. However, very little is known about the relationship between the complexity of the input data along with the type of noise and the depth needed for correct classification. Existing studies do not address the issue of common corruptions adequately, especially in understanding what impact these corruptions leave on the individual part of a deep neural network. Therefore, we can safely assume that the classification (or misclassification) might be happening at a particular layer(s) of a network that accumulates to draw a final correct or incorrect prediction. In this paper, we introduce a novel concept of corruption depth, which identifies the location of the network layer/depth until the misclassification persists. We assert that the identification of such layers will help in better designing the network by pruning certain layers in comparison to the purification of the entire network which is computationally heavy. Through our extensive experiments, we present a coherent study to understand the processing of examples through the network. Our approach also illustrates different philosophies of example memorization and a one-dimensional view of sample or query difficulty. We believe that the understanding of the corruption depth can open a new dimension of model explainability and model compression, where in place of just visualizing the attention map, the classification progress can be seen throughout the network.
更多查看译文
关键词
Neural network functioning,Explainability,Corruption robustness,Corruption vulnerability
AI 理解论文
溯源树
样例
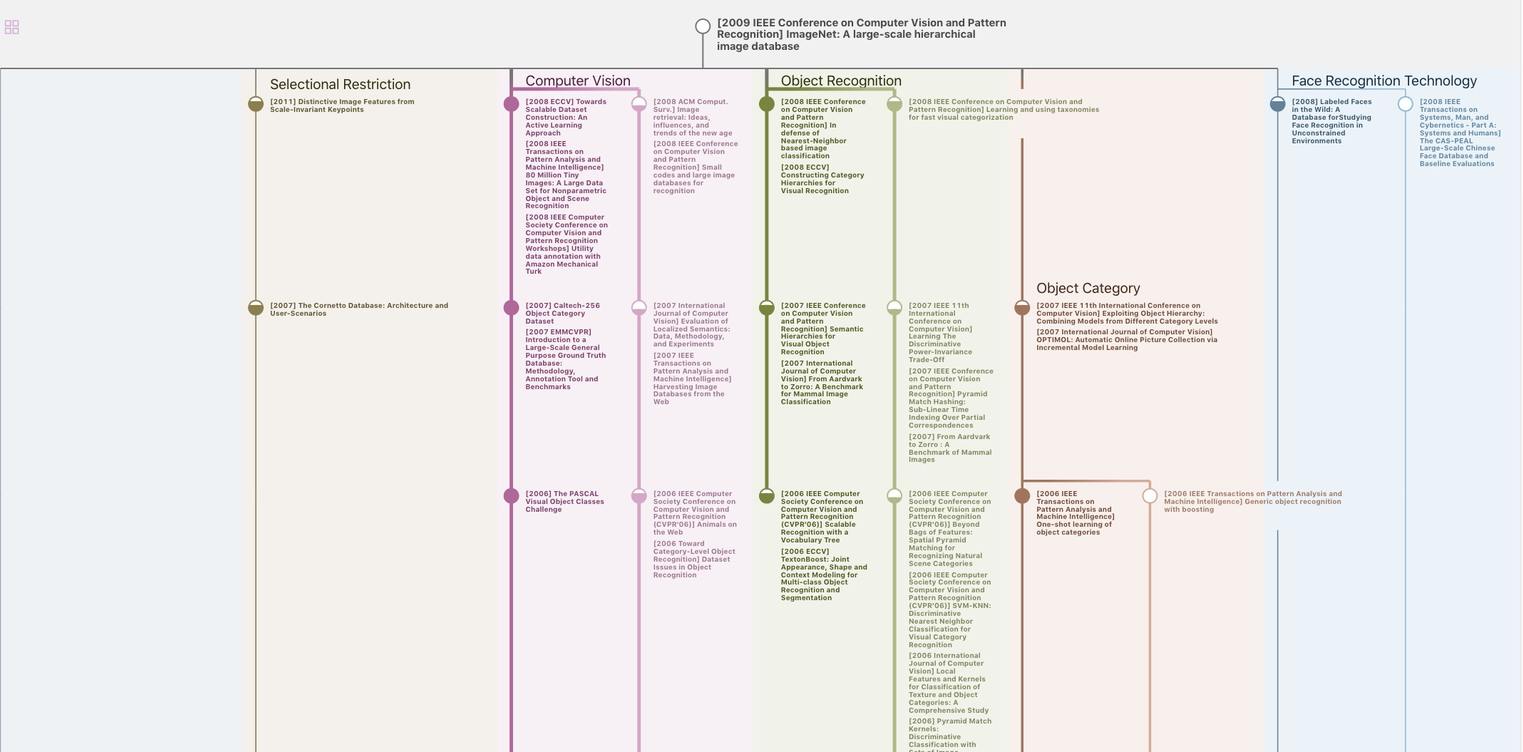
生成溯源树,研究论文发展脉络
Chat Paper
正在生成论文摘要