Concept Drift Handling: A Domain Adaptation Perspective
EXPERT SYSTEMS WITH APPLICATIONS(2023)
Abstract
Data stream prediction is challenging when concepts drift, processing time, and memory constraints come into account. Concept drift refers to changes in data distribution over time that reduces prediction systems’ accuracy. We present a method for handling concept drift with a domain adaptation approach (CDDA) in a data stream. The proposed method passively deals with the concept drift by using the domain adaptation approaches with multiple sources while reducing the model execution time and memory consumption. We introduce two variants of CDDA to transfer the information in the multi-source windows to the target window: weighted multi-source CDDA and multi-source feature alignment CDDA. Then, we theoretically study the behavior of CDDA and find the generalization bound of CDDA for the data stream prediction problem. Moreover, an extensive set of experiments conducted on both synthetic and real-world data streams confirms the validity and excellent performance of the proposed approach. Our code is available at https://github.com/mahan66/cdda.
MoreTranslated text
Key words
Data stream prediction,Domain adaptation,Concept drift,Generalization bound,Uniform entropy number
求助PDF
上传PDF
View via Publisher
AI Read Science
AI Summary
AI Summary is the key point extracted automatically understanding the full text of the paper, including the background, methods, results, conclusions, icons and other key content, so that you can get the outline of the paper at a glance.
Example
Background
Key content
Introduction
Methods
Results
Related work
Fund
Key content
- Pretraining has recently greatly promoted the development of natural language processing (NLP)
- We show that M6 outperforms the baselines in multimodal downstream tasks, and the large M6 with 10 parameters can reach a better performance
- We propose a method called M6 that is able to process information of multiple modalities and perform both single-modal and cross-modal understanding and generation
- The model is scaled to large model with 10 billion parameters with sophisticated deployment, and the 10 -parameter M6-large is the largest pretrained model in Chinese
- Experimental results show that our proposed M6 outperforms the baseline in a number of downstream tasks concerning both single modality and multiple modalities We will continue the pretraining of extremely large models by increasing data to explore the limit of its performance
Upload PDF to Generate Summary
Must-Reading Tree
Example
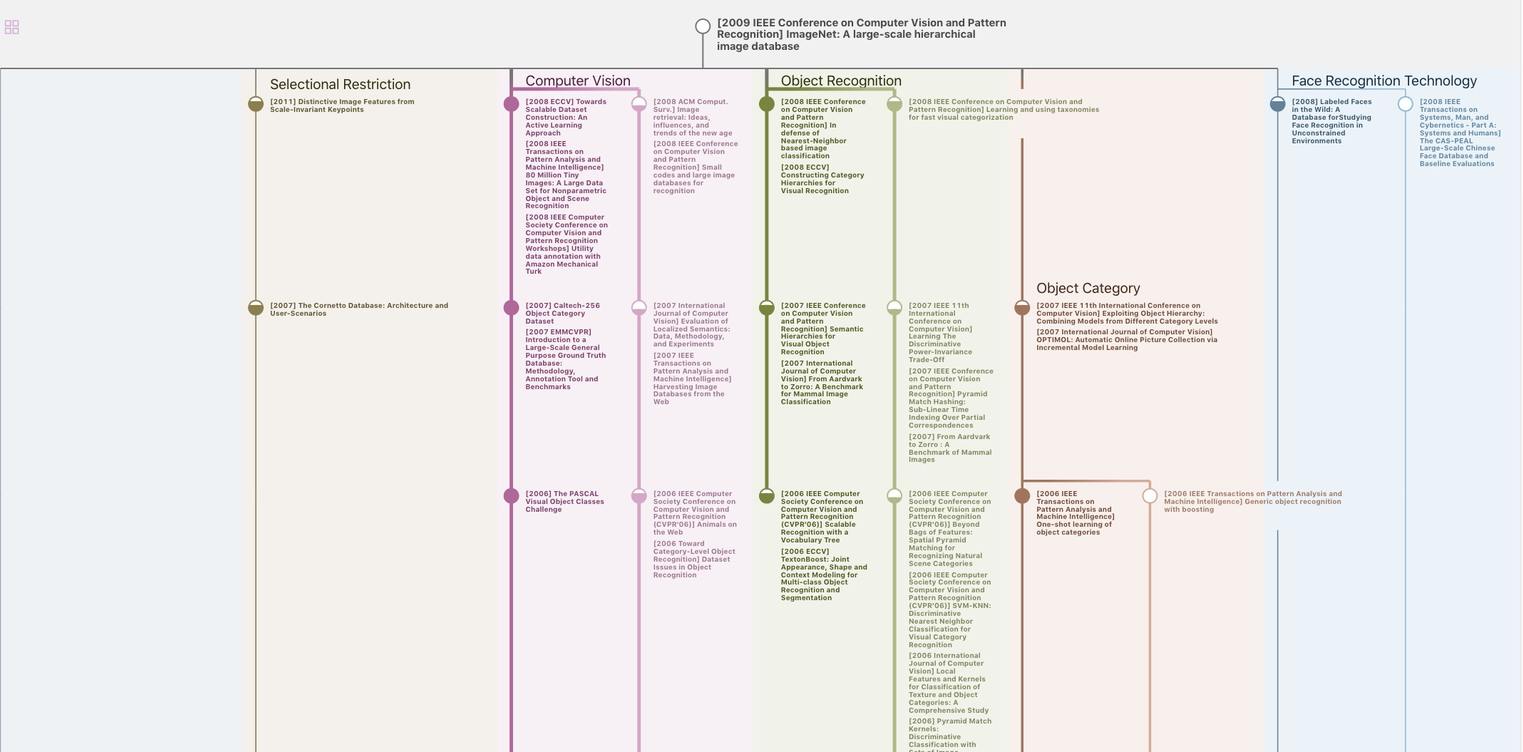
Generate MRT to find the research sequence of this paper
Related Papers
A Novel Edge Architecture and Solution for Detecting Concept Drift in Smart Environments
Future Generation Computer Systems 2023
被引用5
EXPERT SYSTEMS WITH APPLICATIONS 2023
被引用6
ABT-SVDD: A Method for Uncertainty Handling in Domain Adaptation Using Belief Function Theory
APPLIED SOFT COMPUTING 2023
被引用0
Transfer Learning for Concept Drifting Data Streams in Heterogeneous Environments
Knowledge and Information Systems 2024
被引用0
Unsupervised Domain Adaptation for Inter-Session Re-Calibration of Ultrasound-Based HMIs
SENSORS 2024
被引用0
ENGINEERING APPLICATIONS OF ARTIFICIAL INTELLIGENCE 2024
被引用0
Data Disclaimer
The page data are from open Internet sources, cooperative publishers and automatic analysis results through AI technology. We do not make any commitments and guarantees for the validity, accuracy, correctness, reliability, completeness and timeliness of the page data. If you have any questions, please contact us by email: report@aminer.cn
Chat Paper