16.2 A 28nm 53.8TOPS/W 8b Sparse Transformer Accelerator with In-Memory Butterfly Zero Skipper for Unstructured-Pruned NN and CIM-Based Local-Attention-Reusable Engine
2023 IEEE International Solid- State Circuits Conference (ISSCC)(2023)
摘要
Transformer networks, from BERT, GPT to Alphafold, have demonstrated unprecedented advances in a variety of AI tasks. Fig. 16.2.1 shows the computing flow of self-attention - the fundamental operation in transformers. Queries
$(Q)$
, keys
$(K)$
and values (V) are first obtained by multiplying inputs with 3 weight matrices. Afterward, scores that evaluate
$Q-K$
relevance are computed as scaled dot products and converted to probabilities through the softmax function. The probabilities are then multiplied by
$V$
generating the final self-attention results. Transformer networks have led to an explosion in parameter counts, for example, 175B parameters for GPT-3. This demands significant growth in computing hardware and memory. Owing to expanding network sizes and corresponding power consumption, compute-in-memory (CIM) block-wise sparsity-aware architectures were proposed for matrix multiplication [1] and local attention [2] accelerators, where weight storage and compute are skipped for zero-value blocks. Yet, such structured sparsity is at the cost of notable accuracy loss [3]. Consequently, a challenge for CIM-based accelerators is in how to handle unstructured pruned NNs, while maintaining high efficiency. These unstructured patterns can be represented as: 1) irregularly distributed zero weights inside matrices, and 2) varied local attention
s
pans for different attention heads.
更多查看译文
关键词
53.8TOPS/W 8b sparse transformer accelerator,AI tasks,Alphafold,attention heads,BERT,CIM macros,CIM memory reduction,CIM-based accelerators,compute-in-memory block-wise sparsity-aware architectures,computing hardware,GPT-3,in-memory butterfly zero skipper,local attention accelerators,matrix multiplication,network sizes,parameter counts,power consumption,probabilities,Q-K$relevance,scaled dot products,self-attention computing flow,size 28.0 nm,softmax function,transformer networks,transformer operation,unprecedented advances,unstructured patterns,unstructured pruned NNs,weight matrices,weight storage,zero weights,zero-value blocks
AI 理解论文
溯源树
样例
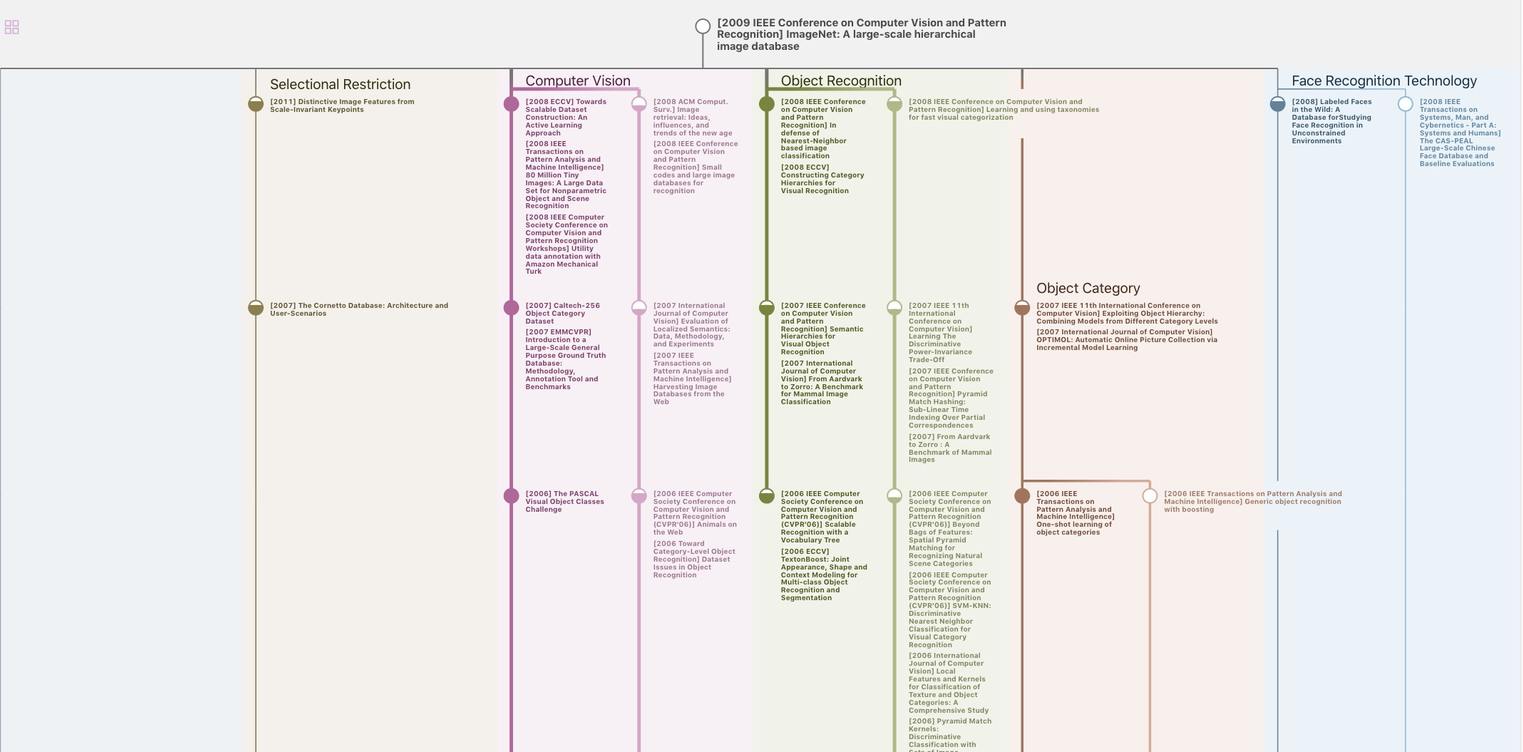
生成溯源树,研究论文发展脉络
Chat Paper
正在生成论文摘要