CrowdFusion: Multisignal Fusion SLAM Positioning Leveraging Visible Light
IEEE Internet of Things Journal(2023)
摘要
With the fast development of location-based services, an ubiquitous indoor positioning approach with high accuracy and low calibration has become increasingly important. In this work, we target on a crowdsourcing approach with zero calibration effort based on visible light, magnetic field, and WiFi to achieve submeter accuracy. We propose a CrowdFusion simultaneous localization and mapping (SLAM) composed of coarse-grained and fine-grained trace merging, respectively, based on the iterative closest point (ICP) SLAM and GraphSLAM. ICP SLAM is proposed to correct the relative locations and directions of crowdsourcing traces and GraphSLAM is further adopted for fine-grained pose optimization. In CrowdFusion SLAM, visible light is used to accurately detect loop closures and magnetic field to extend the coverage. According to the merged traces, we construct a radio map with visible light and WiFi fingerprints. An enhanced particle filter fusing inertial sensors, visible light, WiFi, and floor plan is designed, in which visible light fingerprinting is used to improve the accuracy and increase the resampling/rebooting efficiency. We evaluate CrowdFusion based on comprehensive experiments. The evaluation results show a mean accuracy of 0.67 m for the merged traces and 0.77 m for positioning, merely replying on crowdsourcing traces without professional calibration.
更多查看译文
关键词
Crowdsourcing,simultaneous localization and mapping (SLAM),visible light
AI 理解论文
溯源树
样例
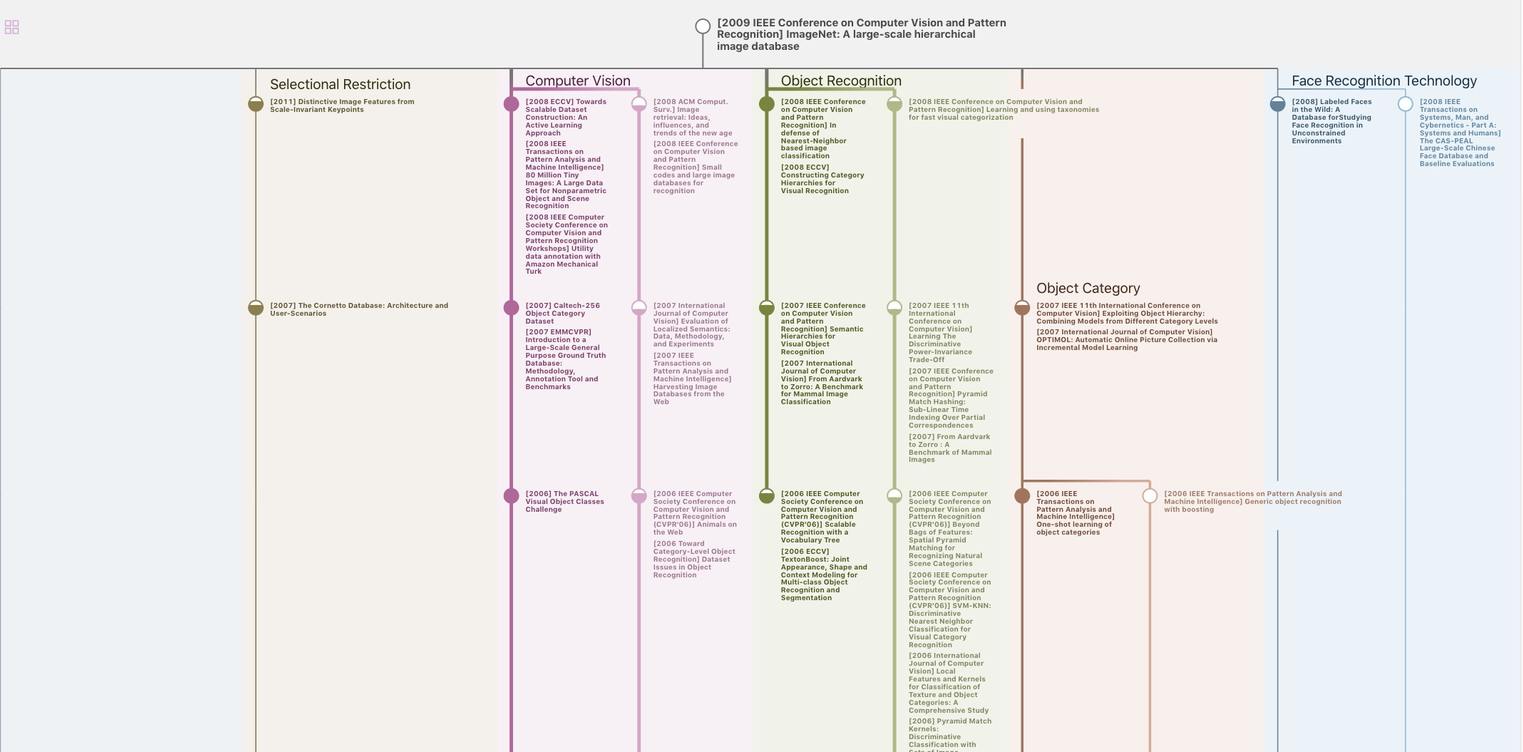
生成溯源树,研究论文发展脉络
Chat Paper
正在生成论文摘要