MetaABR: A Meta-Learning Approach on Adaptative Bitrate Selection for Video Streaming.
IEEE Trans. Mob. Comput.(2024)
摘要
Video streaming is one of the most popular Internet applications that makes up a large amount of Internet traffic. A fundamental mechanism in video streaming is adaptive bitrate (ABR) selection which decides the proper compression level for each chunk of a video to optimize the users' quality of experience (QoE). The existing ABR algorithms require significant tuning and do not generalize to diverse network conditions and personalized QoE objectives. In this paper, we propose a novel framework for meta-learning based ABR design and discuss challenges of deploying learning based ABR mechanism in real-world video streaming systems. We utilize the proposed framework to design MetaABR, a novel adaptive bitrate selection algorithm based on meta-reinforcement learning to maximize users' QoE. By jointly training multiple learning tasks with a shared meta-critic, it can provide transferrable meta-knowledge to supervise bitrate selection across tasks, and can be applied to efficiently learn a new task in unseen environment with only a few trials. We implement MetaABR on an emulation platform which connects to the Linux network protocol stack through virtual network interfaces. Extensive experiments based on real-world traces and wireless testbed show that MetaABR achieves the best comprehensive QoE compared with the state-of-the-art ABR algorithms in a variety of network environments.
更多查看译文
关键词
Video Streaming,Bitrate Adaptation,Reinforcement Learning,Meta-Learning
AI 理解论文
溯源树
样例
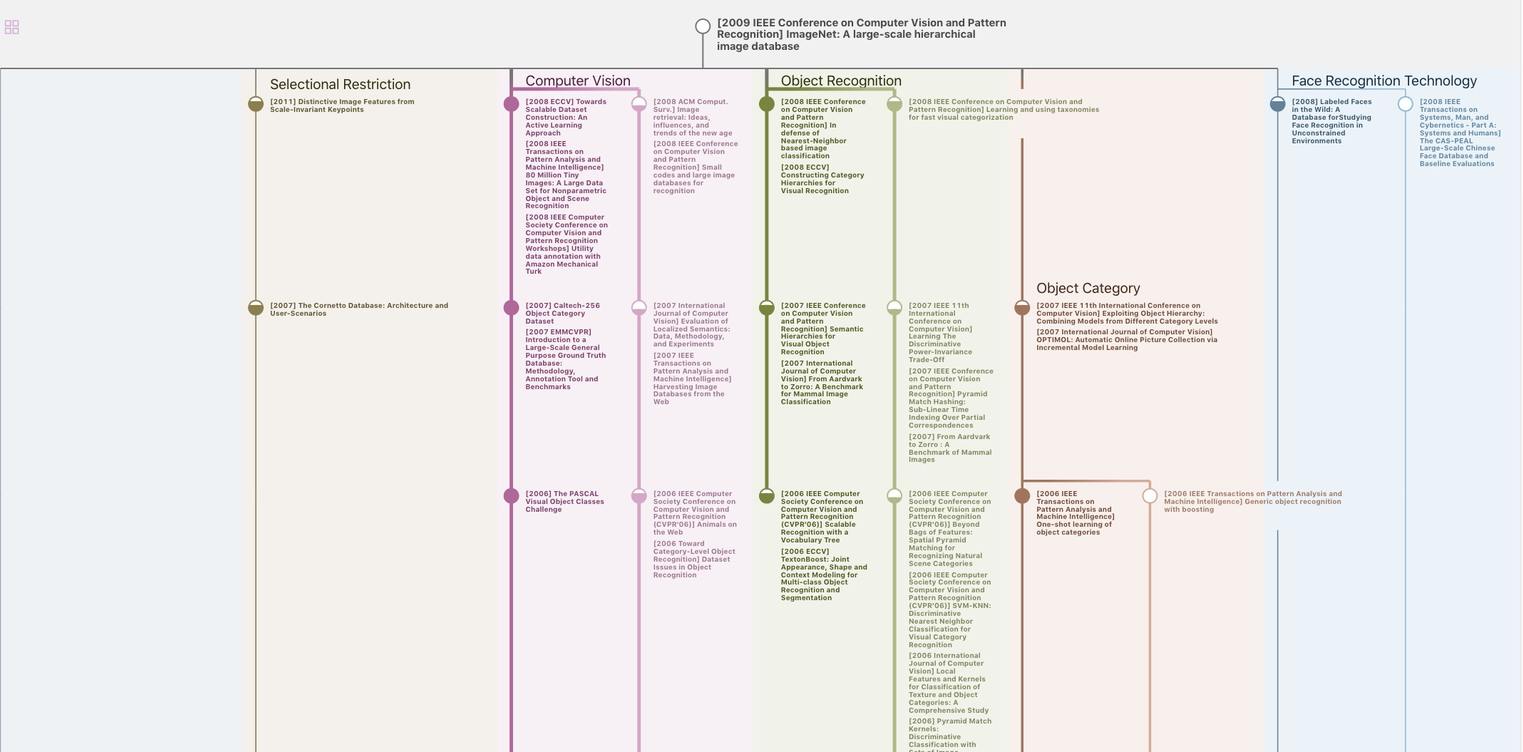
生成溯源树,研究论文发展脉络
Chat Paper
正在生成论文摘要