Dental Detection and Classification of YOLOv3-SPP based on Convolutional Block Attention Module
2022 IEEE 8th International Conference on Computer and Communications (ICCC)(2022)
摘要
The purpose of this research paper is to implement the tooth target detection task by deep convolutional neural networks. In order to solve the problems of low accuracy of target detection due to the high similarity between teeth and complex tooth textures, an improved tooth detection method with YOLOv3-SPP model is proposed in this paper. This method incorporates the convolutional block attention module (CBAM) in the YOLOv3-SPP algorithm framework, and improves the performance of the network features by adding the channel attention mechanism and spatial attention mechanism to the feature extraction network to enhance the saliency of the tooth target region in the image. Secondly, CIoU border regression loss is introduced to improve the localization accuracy. In addition, a Non-maximum suppression (NMS) method is used to solve the candidate frame overlap problem. Through experiments, it is shown that the mAP of the modified YOLOv3-SPP target detection model is improved to 86.8 percent, which indicates that the improved model can be applied to the detection of teeth.
更多查看译文
关键词
Tooth detection classification,YOLOv3-SPP,CBAM,CIoU loss,NMS
AI 理解论文
溯源树
样例
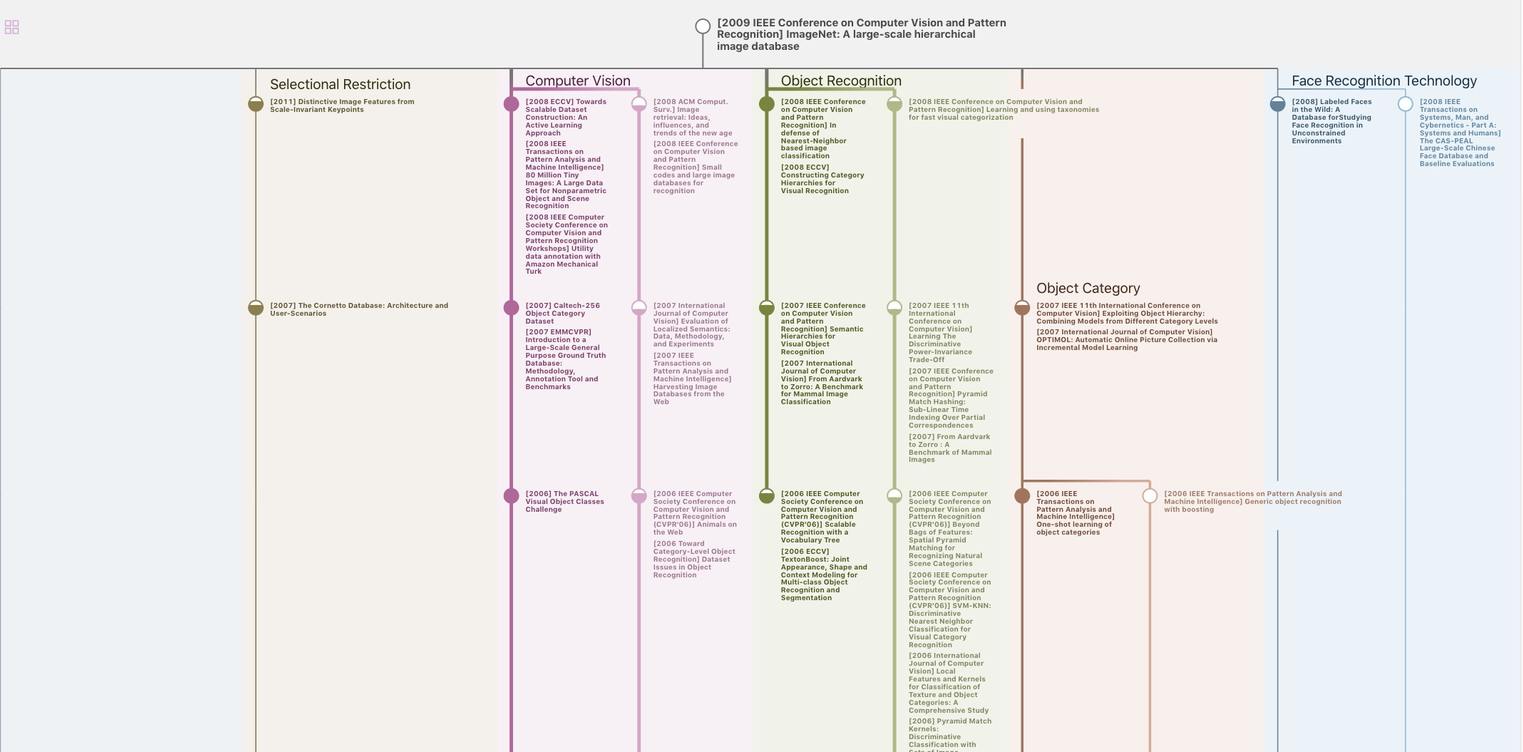
生成溯源树,研究论文发展脉络
Chat Paper
正在生成论文摘要