Assessment of Spatial and Temporal Modeling on Greenhouse Gas Emissions From Electricity Generation
IEEE Access(2023)
摘要
This paper highlights the importance of precise assessments of greenhouse gas (GHG) emissions associated with power generation for effective policy making in environmental sustainability. The current assessment approaches based on historical data or estimated generation using energy models may not accurately reflect the reality of future power systems due to the impact of spatial-temporal and techno-economic characteristics of generation mix and load demands. To address this, the paper presents a comprehensive methodology for accurately quantifying the geographical and temporal variations in GHG emissions associated with generating units' operation, startup, and shutdown at an hourly resolution. The methodology is based on a detailed electricity model that considers various sources of generation, techno-economic, and spatial-temporal characteristics of system components. The study demonstrates the effectiveness of the methodology in quantifying GHG emissions in the IEEE RTS-GLMC system, with a focus on CO2, N2O, and CH4. The analysis reveals significant variations in GHG emissions among different generation buses and hours of the year, attributed to the high proportion of renewable energy in the generation mix. The paper emphasizes the inadequacy of examining marginal environmental impacts based on GHG emission intensity alone and suggests a more thorough analysis based on total GHG emissions generation. Finally, the paper emphasizes the crucial role of time-varying and marginal assessment techniques in identifying effective strategies for reducing GHG emissions in the electricity sector, including optimizing the operation and capacity of generation units, energy storage systems, and electric vehicles, including their locations.
更多查看译文
关键词
Renewable energy sources, Load modeling, Power generation, Greenhouse effect, Data models, Fossil fuels, Energy storage, Energy, GHG emissions, renewable generation, energy storage, electric vehicle
AI 理解论文
溯源树
样例
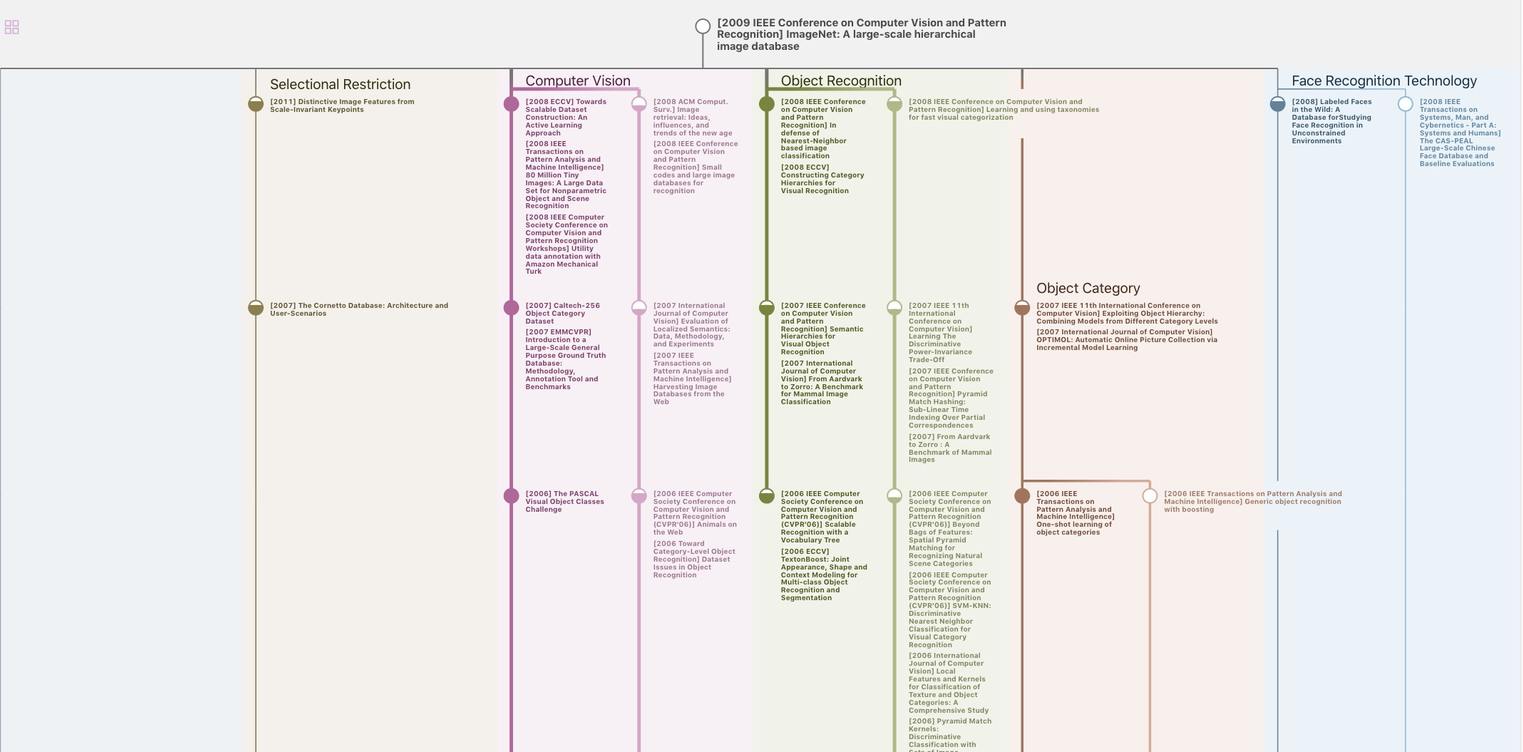
生成溯源树,研究论文发展脉络
Chat Paper
正在生成论文摘要