History-Agnostic Battery Degradation Inference
Journal of energy storage(2024)
摘要
Lithium-ion batteries (LIBs) have attracted widespread attention as an efficient energy storage device on electric vehicles (EV) to achieve emission-free mobility. However, the performance of LIBs deteriorates with time and usage, and the state of health of used batteries are difficult to quantify. Having accurate estimations of a battery’s remaining life across different life stages would benefit maintenance, safety, and serve as a means of qualifying used batteries for second-life applications. Since the full history of a battery may not always be available in downstream applications, in this study, we demonstrate a deep learning framework that enables dynamic degradation rate prediction, including both short-term and long-term forecasting, while requiring only the most recent battery usage information. Specifically, our model takes a rolling window of current and voltage time-series inputs, and predicts the near-term and long-term capacity fade via a recurrent neural network. We exhaustively benchmark our model against a naive extrapolating model by evaluating the error on reconstructing the discharge capacity profile under different settings. We show that our model’s performance in accurately inferring the battery’s degradation profile is agnostic with respect to cell cycling history and its current state of health. This approach can provide a promising path towards evaluating battery health in running vehicles, enhance edge-computing battery diagnostics, and determine the state of health for used batteries with unknown cycling histories.
更多查看译文
关键词
Battery degradation,Used battery,Unknown cycling history,Deep learning,Second life applications
AI 理解论文
溯源树
样例
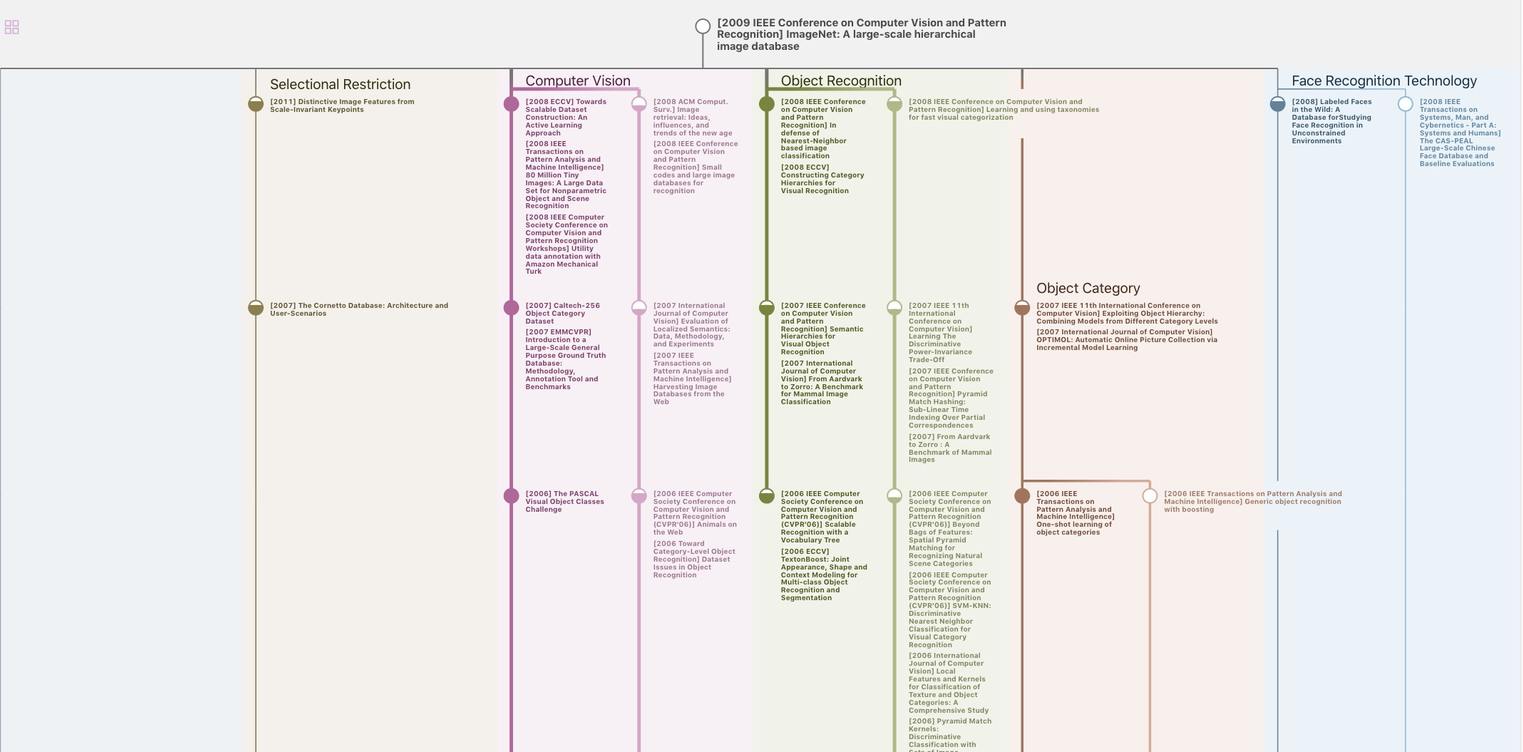
生成溯源树,研究论文发展脉络
Chat Paper
正在生成论文摘要