Modeliranje dinamike pečenja za določanje stanja pečenja z mrežami LSTM
ROSUS 2023 - Računalniška obdelava slik in njena uporaba v Sloveniji 2023: Zbornik 17. strokovne konference(2023)
Abstract
Osnovni način, da dosežemo dobre rezultate pri pečenju je prilagoditev časa – če želi ljubiteljski kuhar bolj zapečene piškote bo podaljšal čas peke. Ta pristop ne zagotavlja vedno istih rezultatov in lahko vodi v preveč ali premalo zapečene jedi. Za reševanje tega problema je bilo razvitih že več sistemov računalniškega vida, ni pa še bilo izvedene sistematične študije, ki bi razviti sistem primerjala z izkušenim domačim kuharjem. V tem delu predstavimo sistem računalniškega vida, ki je sestavljen iz pečice s kamero, sistema za zajemanje slik in globokih nevronskih modelov. Delovanje sistema primerjamo z modelom ljubiteljskega kuharja. Ker se videz jedi v pečici spreminja skozi čas, poleg konvolucijskega modela CNN uporabimo dve vrsti modelov, ki na vhodu sprejmeta zaporedje slik - CNN-LSTM in ConvLSTM. Rezultati kažejo, da model ConvLSTM prekaša model ljubiteljskega kuharja za 5 odstotnih točk v metriki F1. Da so modeli primerni za spremljanje kvalitete jedi v pečici, morajo imeti sposobnost učenja dinamike pečenja.
MoreTranslated text
求助PDF
上传PDF
View via Publisher
AI Read Science
AI Summary
AI Summary is the key point extracted automatically understanding the full text of the paper, including the background, methods, results, conclusions, icons and other key content, so that you can get the outline of the paper at a glance.
Example
Background
Key content
Introduction
Methods
Results
Related work
Fund
Key content
- Pretraining has recently greatly promoted the development of natural language processing (NLP)
- We show that M6 outperforms the baselines in multimodal downstream tasks, and the large M6 with 10 parameters can reach a better performance
- We propose a method called M6 that is able to process information of multiple modalities and perform both single-modal and cross-modal understanding and generation
- The model is scaled to large model with 10 billion parameters with sophisticated deployment, and the 10 -parameter M6-large is the largest pretrained model in Chinese
- Experimental results show that our proposed M6 outperforms the baseline in a number of downstream tasks concerning both single modality and multiple modalities We will continue the pretraining of extremely large models by increasing data to explore the limit of its performance
Upload PDF to Generate Summary
Must-Reading Tree
Example
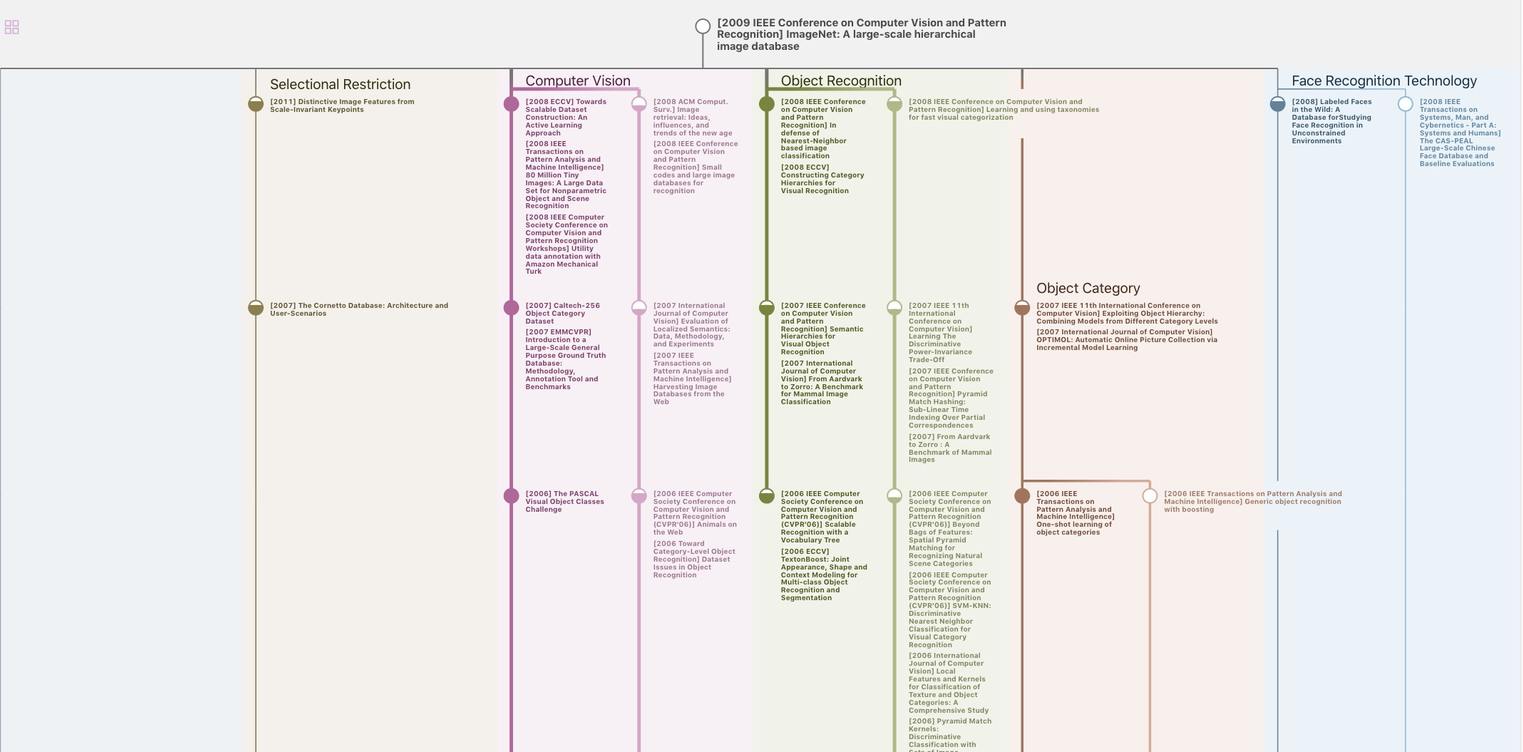
Generate MRT to find the research sequence of this paper
Data Disclaimer
The page data are from open Internet sources, cooperative publishers and automatic analysis results through AI technology. We do not make any commitments and guarantees for the validity, accuracy, correctness, reliability, completeness and timeliness of the page data. If you have any questions, please contact us by email: report@aminer.cn
Chat Paper