Randomized Strategies for Robust Combinatorial Optimization with Approximate Separation
ALGORITHMICA(2024)
摘要
In this paper, we study the following robust optimization problem. Given a set family representing feasibility and candidate objective functions, we choose a feasible set, and then an adversary chooses one objective function, knowing our choice. The goal is to find a randomized strategy (i.e., a probability distribution over the feasible sets) that maximizes the expected objective value in the worst case. This problem is fundamental in wide areas such as artificial intelligence, machine learning, game theory, and optimization. To solve the problem, we provide a general framework based on the dual linear programming problem. In the framework, we utilize the ellipsoid algorithm with the approximate separation algorithm. We prove that there exists an alpha-approximation algorithm for our robust optimization problem if there exists an alpha-approximation algorithm for finding a (deterministic) feasible set that maximizes a nonnegative linear combination of the candidate objective functions. Using our result, we provide approximation algorithms for the max-min fair randomized allocation problem and the maximum cardinality robustness problem with a knapsack constraint.
更多查看译文
关键词
Robust optimization,Ellipsoid method,Max-min fair allocation,Maximum cardinality robustness problem
AI 理解论文
溯源树
样例
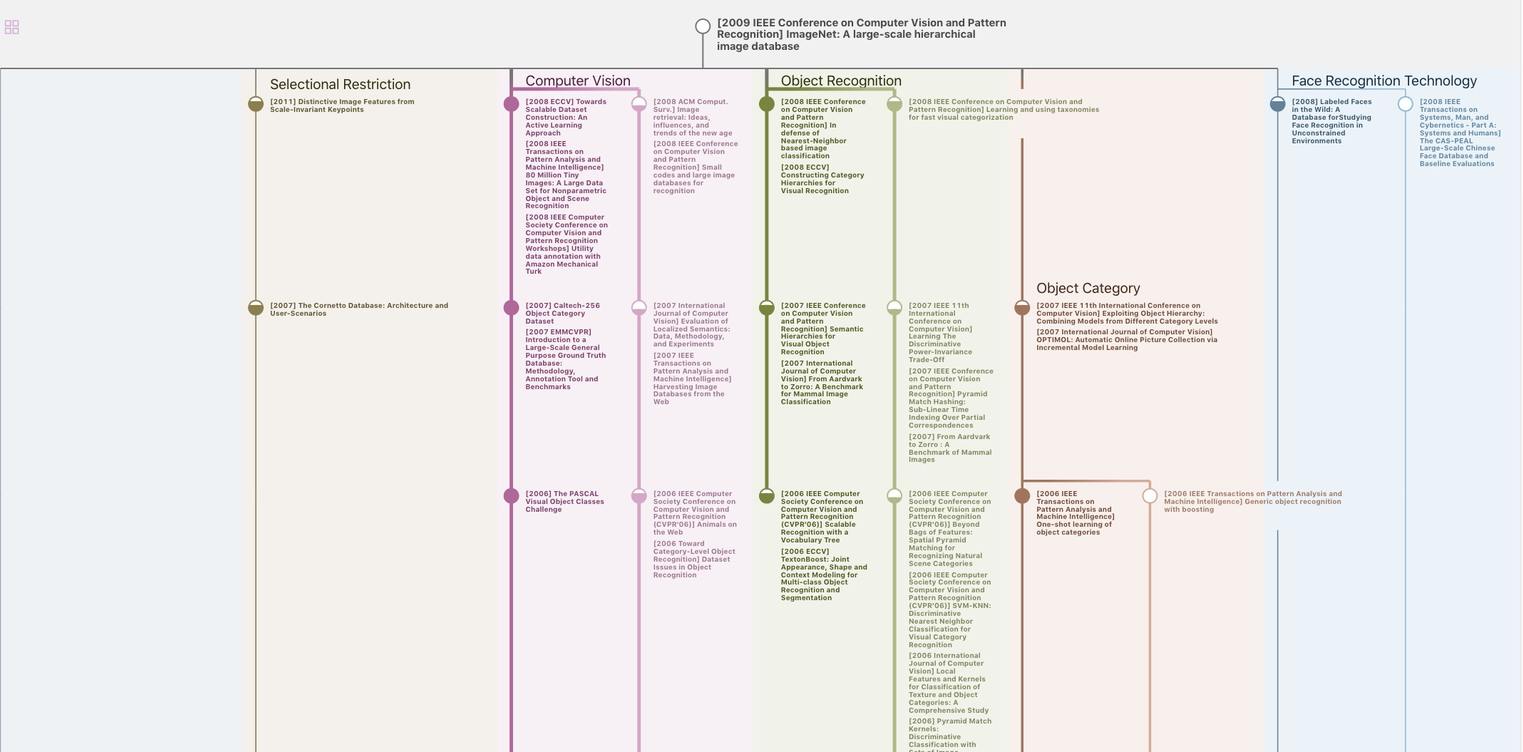
生成溯源树,研究论文发展脉络
Chat Paper
正在生成论文摘要