Gender Identification Using Marginalised Stacked Denoising Autoencoders on Twitter Data
Intelligent Automation & Soft Computing(2023)
摘要
Gender analysis of Twitter could reveal significant socio-cultural differ-ences between female and male users. Efforts had been made to analyze and auto-matically infer gender formerly for more commonly spoken languages' content, but, as we now know that limited work is being undertaken for Arabic. Most of the research works are done mainly for English and least amount of effort for non-English language. The study for Arabic demographic inference like gen-der is relatively uncommon for social networking users, especially for Twitter. Therefore, this study aims to design an optimal marginalized stacked denoising autoencoder for gender identification on Arabic Twitter (OMSDAE-GIAT) model. The presented OMSDAE-GIAR technique mainly concentrates on the identifica-tion and classification of gender exist in the Twitter data. To attain this, the OMS-DAE-GIAT model derives initial stages of data pre-processing and word embedding. Next, the MSDAE model is exploited for the identification of gender into two classes namely male and female. In the final stage, the OMSDAE-GIAT technique uses enhanced bat optimization algorithm (EBOA) for parameter tuning process, showing the novelty of our work. The performance validation of the OMSDAE-GIAT model is inspected against an Arabic corpus dataset and the results are measured under distinct metrics. The comparison study reported the enhanced performance of the OMSDAE-GIAT model over other recent approaches.
更多查看译文
关键词
Arabic twitter, gender identification, bat algorithm, hybrid deep learning, social media, arabic corpus
AI 理解论文
溯源树
样例
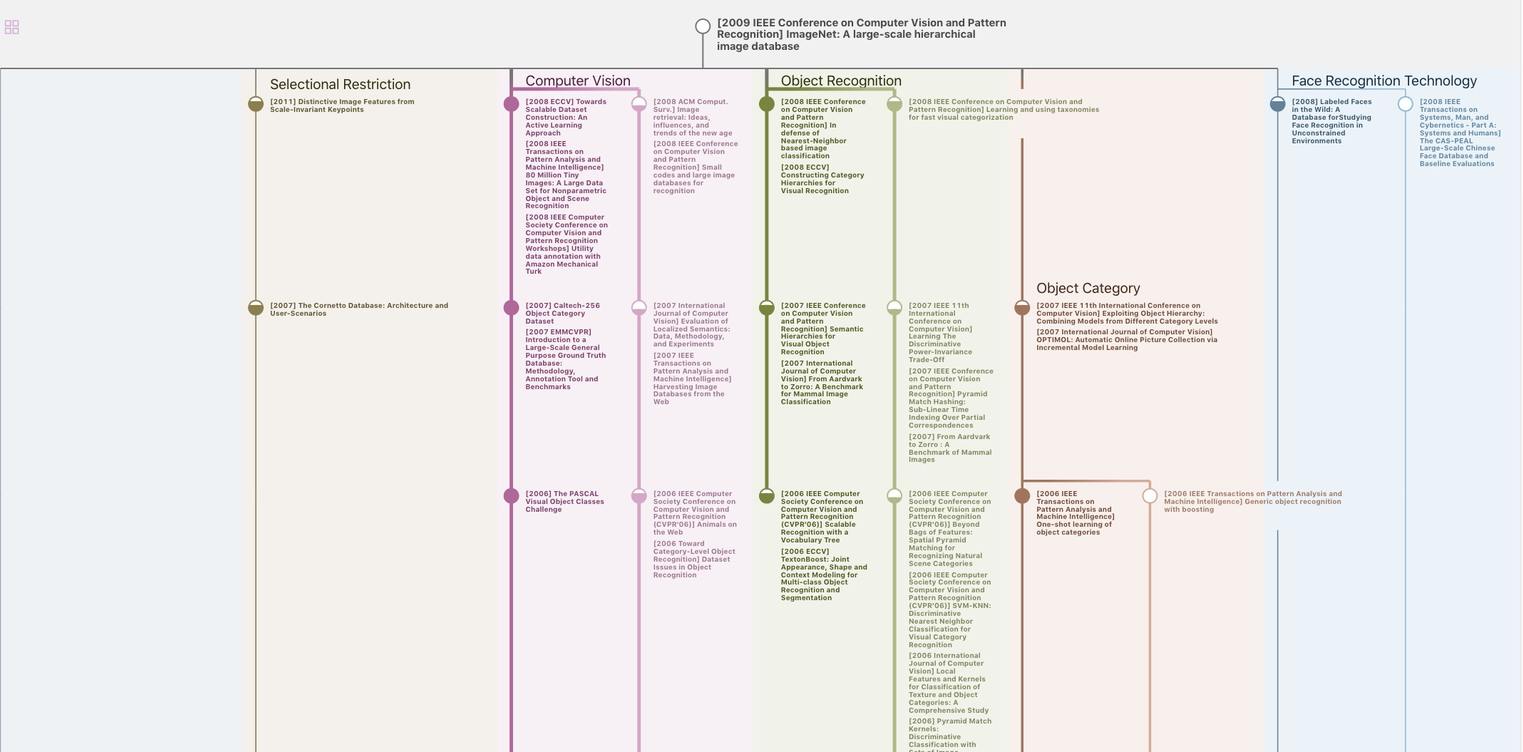
生成溯源树,研究论文发展脉络
Chat Paper
正在生成论文摘要