Fitting objects with implicit polynomials by deep neural network
Optoelectronics Letters(2023)
摘要
Implicit polynomials (IPs) are considered as a powerful tool for object curve fitting tasks due to their simplicity and fewer parameters. The traditional linear methods, such as 3L, MinVar, and MinMax, often achieve good performances in fitting simple objects, but usually work poorly or even fail to obtain closed curves of complex object contours. To handle the complex fitting issues, taking the advantages of deep neural networks, we designed a neural network model continuity-sparsity constrained network (CSC-Net) with encoder and decoder structure to learn the coefficients of IPs. Further, the continuity constraint is added to ensure the obtained curves are closed, and the sparseness constraint is added to reduce the spurious zero sets of the fitted curves. The experimental results show that better performances have been obtained on both simple and complex object fitting tasks.
更多查看译文
关键词
fitting objects,implicit polynomials,neural
AI 理解论文
溯源树
样例
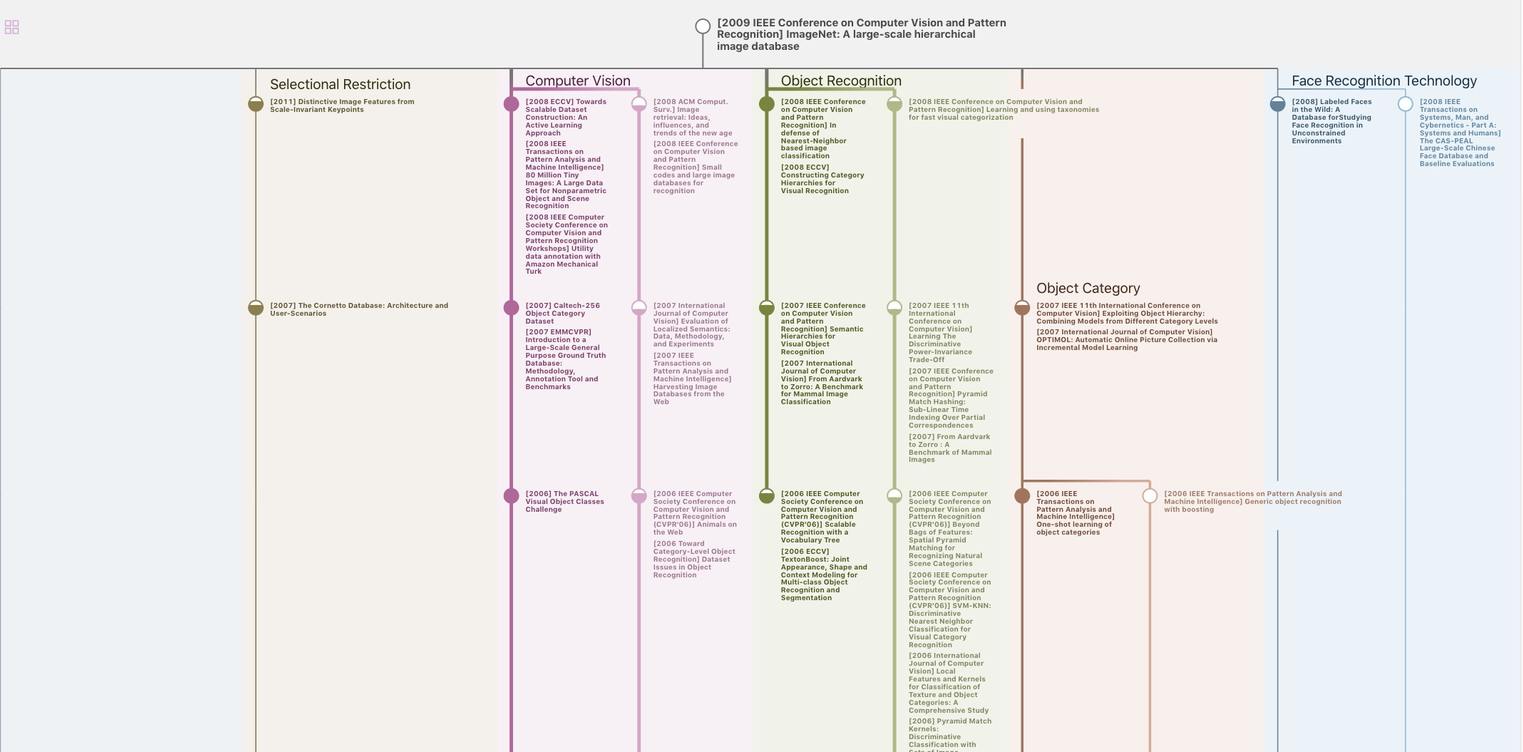
生成溯源树,研究论文发展脉络
Chat Paper
正在生成论文摘要