Efficiently Approximating High-Dimensional Pareto Frontiers for Tree-Structured Networks Using Expansion and Compression
Integration of Constraint Programming, Artificial Intelligence, and Operations Research(2023)
摘要
Real-world decision-making often involves working with many distinct objectives. However, as we consider a larger number of objectives, performance degrades rapidly and many instances become intractable. Our goal is to approximate higher-dimensional Pareto frontiers within a reasonable amount of time. Our work is motivated by a problem in computational sustainability that evaluates the tradeoffs between various ecological impacts of hydropower dam proliferation in the Amazon river basin. The current state-of-the-art algorithm finds a good approximation of the Pareto frontier within hours for three-objective problems, but a six-objective problem cannot be solved in a reasonable amount of time. To tackle this problem, we developed two different approaches: an expansion method, which assembles Pareto-frontiers optimized with respect to subsets of the original set of criteria, and a compression method, which assembles Pareto-frontiers optimized with respect to compressed criteria, which are a weighted sum of multiple original criteria. Our experimental results show that the aggregation of the different methods can reliably provide good approximations of the true Pareto-frontiers in practice. Source code and data are available at
https://github.com/gomes-lab/Dam-Portfolio-Selection-Expansion-and-Compression-CPAIOR
.
更多查看译文
关键词
Multi-objective Optimization, Approximation DP
AI 理解论文
溯源树
样例
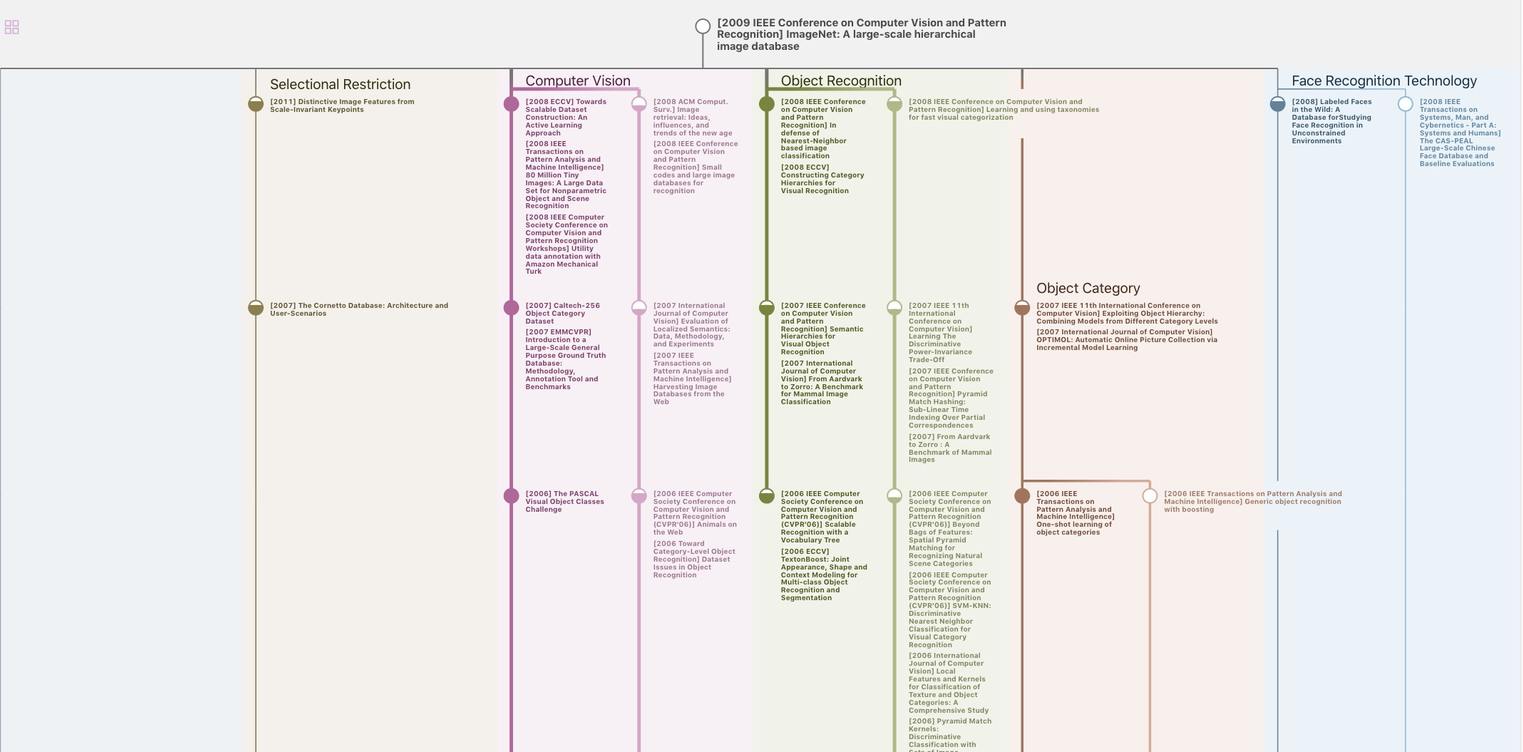
生成溯源树,研究论文发展脉络
Chat Paper
正在生成论文摘要