Can LLMs Facilitate Interpretation of Pre-trained Language Models?
CoRR(2023)
摘要
Work done to uncover the knowledge encoded within pre-trained language models, rely on annotated corpora or human-in-the-loop methods. However, these approaches are limited in terms of scalability and the scope of interpretation. We propose using a large language model, ChatGPT, as an annotator to enable fine-grained interpretation analysis of pre-trained language models. We discover latent concepts within pre-trained language models by applying hierarchical clustering over contextualized representations and then annotate these concepts using GPT annotations. Our findings demonstrate that ChatGPT produces accurate and semantically richer annotations compared to human-annotated concepts. Additionally, we showcase how GPT-based annotations empower interpretation analysis methodologies of which we demonstrate two: probing framework and neuron interpretation. To facilitate further exploration and experimentation in this field, we have made available a substantial ConceptNet dataset comprising 39,000 annotated latent concepts.
更多查看译文
AI 理解论文
溯源树
样例
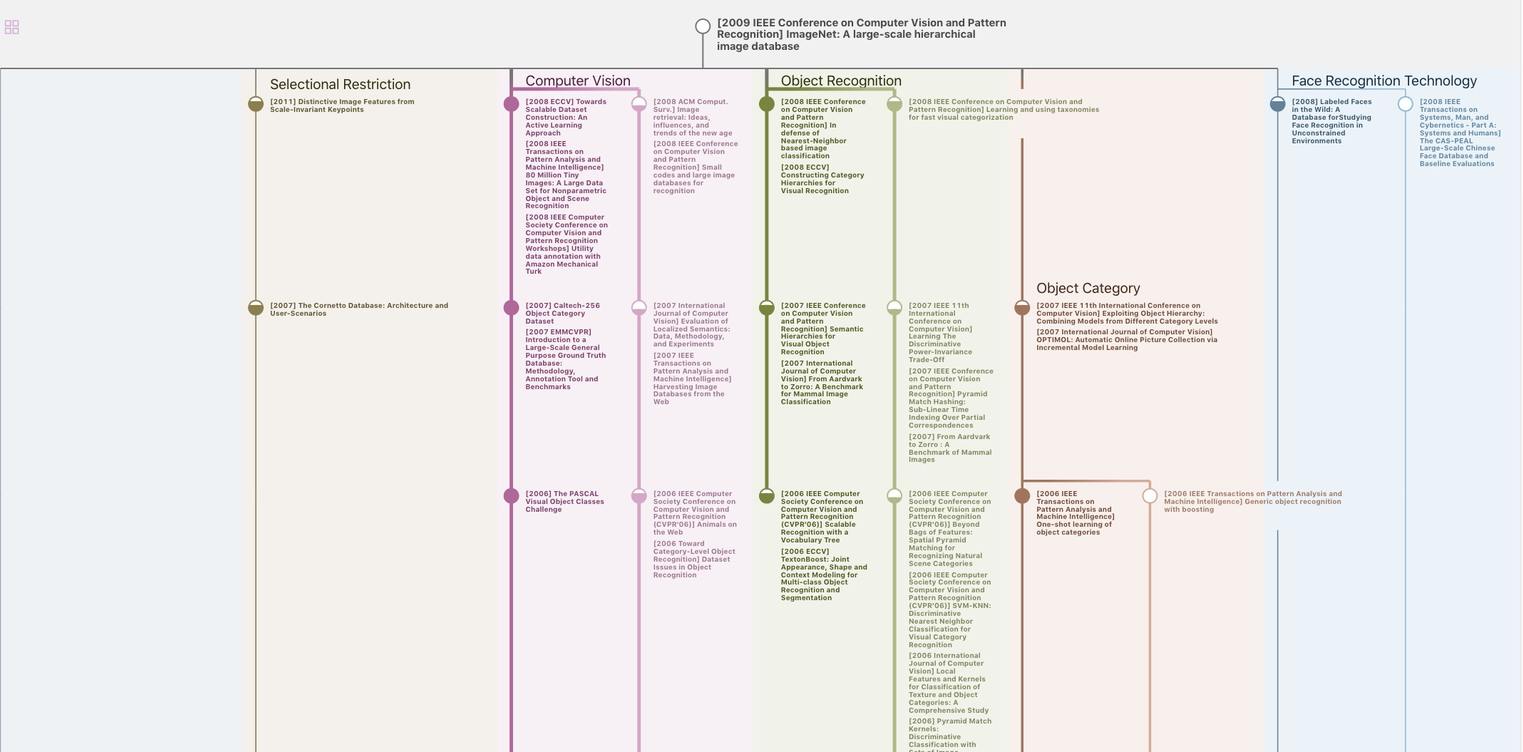
生成溯源树,研究论文发展脉络
Chat Paper
正在生成论文摘要